Efficient Data Governance: Roadmap, Barriers to Success, and Best Practices
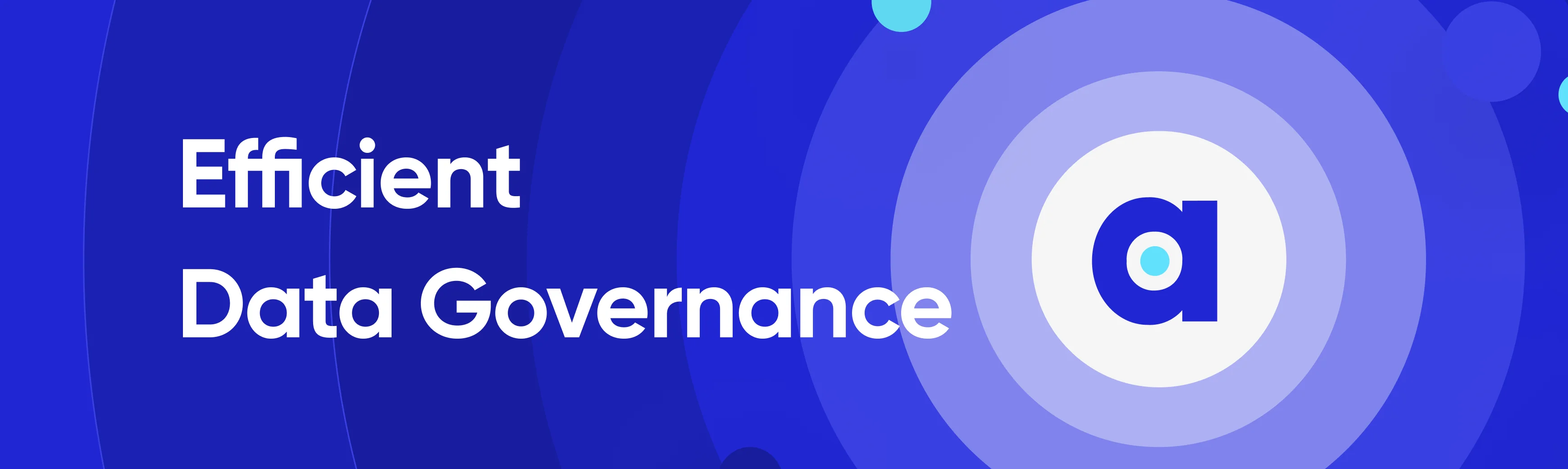
Share this article
Efficient data governance plays a crucial role in ensuring the quality, security, and proper utilization of data throughout its lifecycle. By implementing robust data governance practices, organizations can establish guidelines, standards, and best practices that promote data accuracy, consistency, reliability, and quality.
In addition to data quality and compliance, efficient data governance helps organizations manage and control their data assets effectively by defining roles and responsibilities that ensure clear accountability and ownership.
In this article, we’ll look at what makes a data governance program efficient by delving into the framework, challenges, and best practices.
Table of contents #
- Efficient data governance framework: A roadmap on getting started
- Barriers to efficient data governance
- Best practices for efficient data governance
- Summing up
- Efficient data governance: Related reads
Efficient data governance framework: A roadmap on getting started #
Implementing a robust data governance framework is essential for organizations to effectively collect, manage, secure, and store their data assets. This involves:
- Articulating data governance goals and objectives
- Evaluating the architecture of your current data landscape
- Developing a framework that’s community-centered and collaborative
- Defining data governance metrics to maximize business value
- Starting with small prototypes
Let’s explore each aspect further.
Articulating data governance goals and objectives #
Start by clearly articulating the goals and objectives of your data governance program. Make sure that they align with your business goals.
Here are the primary goals of data governance:
- Minimize risks associated with data management
- Establish standards and compliance requirements to ensure legal, ethical, and responsible data practices
- Improve internal and external communication regarding data management practices, as well as collaboration between different teams and stakeholders
- Increase data asset value by improving data quality, consistency, and availability
- Reduce costs by optimizing data storage, use, management, and maintenance, and avoiding non-compliance fines
Also, read → Key objectives of data governance
Evaluating the architecture of your current data landscape #
Next, evaluate the architecture of your current data landscape. Identify existing data assets, data sources, data flows, data systems, and data-related processes. Explore how your organization handles:
- Data integration and interoperability
- Data modeling and design
- Data storage and operations
- Metadata management
- Reference and master data management
- Data documentation and context
- BI and reporting
- Data security, compliance, and quality assurance
Developing a framework that’s community-centered and collaborative #
To successfully implement data governance, organizations should adopt a structured, community-centered, and collaborative approach.
The Three Lines of Defense model is an example of a data governance model that involves collaboration between business stakeholders, IT departments, and dedicated data governance teams.
Collaboration among stakeholders is crucial throughout the data governance journey. Business stakeholders provide insights into data requirements, usage, and compliance needs, while IT teams offer technical expertise in implementing data governance solutions.
Also, read → Data governance framework examples
Defining data governance metrics to maximize business value #
Define key performance indicators (KPIs) and metrics to measure the effectiveness of your data governance framework. This could include:
- Data quality metrics, such as data accuracy rate, data completeness rate, and data timeliness
- Compliance metrics, such as the percentage of data assets complying with data privacy laws and the number of data security incidents
- Data governance adoption metrics, such as the percentage of data assets covered by the data governance framework, adoption rate of data governance tools and platforms, etc.
- Impact on business outcomes in terms of cost savings, quality and speed of decision-making, improvement in business processes and daily workflows, etc.
Also, read → Maximizing business value through data governance
Starting with small prototypes #
To ensure the success of data governance initiatives, it is recommended to start with a manageable or application-specific prototype project. It is essential to define goals, understand the benefits, analyze the current state, and develop a roadmap before convincing stakeholders and budgeting for the project.
By implementing the framework with small prototypes that can translate into quick wins, you can demonstrate the benefits to all the stakeholders involved. This approach also helps you to learn from the implementation process and gain valuable insights before scaling the data governance program across the organization.
Barriers to efficient data governance #
Implementing data governance initiatives can be met with several common challenges and problems. These include:
- Lack of data leadership and organizational buy-in: Without clear leadership, data governance initiatives may lack the necessary support and direction, hindering their effectiveness.
- Lack of resources allocated to data governance: Budget constraints and insufficient staffing can impede the progress of data governance initiatives.
- Inadequate data governance framework and strategy: Organizations often lack a proper strategy outlining data governance goals, objectives, and roadmap. Lack of clarity or direction in data governance is a major roadblock to efficient data governance.
- Siloed data: Over time, data tends to become fragmented and segregated as different lines of business or functions develop new data sources and adopt new technologies. Siloed data can lead to inconsistencies, duplication, and poor data quality.
- Data quality issues: Data quality issues, such as inconsistencies and inaccuracies, can undermine the integrity of data and diminish trust in its usage.
- Data ownership issues: When data is generated and used by multiple teams, it can be tough to understand who owns what data. When data ownership is unclear or ambiguous, assigning data stewardship roles and responsibilities becomes a challenge.
- Resistance to change: Implementing data governance often requires changes to existing processes, systems, and workflows. However, change is hard and your employees may resist it as they’re comfortable with existing practices.
To overcome these challenges, practical strategies can be implemented. Let’s explore some best practices to ensure the effectiveness of data goverannce programs.
Best practices for efficient data governance #
Implementing efficient data governance requires adherence to a set of best practices that promote effective data management and decision-making. Here are practical guidelines for successful data governance implementation:
- Identify critical data elements and explore how to classify them
- Set policies and procedures for the entire data lifecycle
- Involve business users in the process
- Don’t neglect active metadata management
- Don’t over-restrict data use
- Ensure continuous monitoring, evaluation, and adaptability
Let’s explore the specifics of each best practice.
Identify critical data elements and explore how to classify them #
Determine the key data elements that are vital for your organization’s operations and decision-making. Create an inventory of all such assets and then explore how to classify them in terms of sensitivity, criticality, and compliance requirements.
Set policies and procedures for the entire data lifecycle #
Establish clear policies and procedures that govern the collection, storage, processing, and sharing of data throughout its lifecycle.
Also, define data governance processes that align with industry best practices and regulatory requirements.
Make sure that you communicate these policies and procedures clearly with all stakeholders across your organization.
Read more → Data governance policy with examples and templates
Involve business users in the process #
Collaborating with all stakeholders ensures that data governance initiatives align with business objectives and address their specific needs. So, engage business users as active participants in the data governance process.
A better approach is to treat each data asset producer as a product owner and make them responsible for the security, integrity, privacy, and governance of the assets they create.
Also, read → How to get started with a personalized, bottom-up approach to data governance
Don’t neglect active metadata management #
Metadata is crucial for contextual information on your data assets. Active metadata management can help with data classification, data access controls, data retention policies, and meeting data privacy requirements.
Read more → Your 101 guide to active metadata management
Don’t over-restrict data use #
Balance the need for data security and compliance with enabling data accessibility and usability. Avoid excessive restrictions that hinder innovation and productivity, while ensuring data privacy and protection.
Read more → How data governance and compliance is an act of checks and balances
Ensure continuous monitoring, evaluation, and adaptability #
Continuous monitoring and adaptability allow organizations to refine their data governance approach over time, ensuring its effectiveness in meeting evolving business and regulatory requirements.
So, implement mechanisms for ongoing monitoring and evaluation of data governance processes and outcomes. Keep track of your data governance KPIs and get feedback from the relevant stakeholders to improve further.
Also, embrace a culture of continuous improvement and adaptability to address emerging challenges and evolving data management needs.
Also, read → Modern data culture 101
Summing up #
Efficient data governance is a critical component of successful data management, enabling organizations to harness the full potential of their data assets. We have explored various aspects of efficient data governance, including a roadmap with success factors, challenges, and best practices.
Bear in mind that data governance is a continuous process that requires consistent efforts to review, refine, and improve your existing data governance framework, policies, procedures, and practices.
Efficient data governance: Related reads #
- What is Data Governance? Its Importance, Principles & How to Get Started?
- Key Objectives of Data Governance: How Should You Think About Them?
- Data Governance Framework Examples, Templates, Standards, Best Practices & How to Create One?
- Data Governance and Compliance: Act of Checks & Balances
- How to implement data governance? Steps, Prerequisites, Essential Factors & Business Case
- How to Improve Data Governance? Steps, Tips & Template
- 7 Steps to Simplify Data Governance for Your Entire Organization
- Automated Data Governance: How Does It Help You Manage Access, Security & More at Scale?
- Enterprise Data Governance Basics, Strategy, Key Challenges, Benefits & Best Practices
- Data Governance in Manufacturing: Steps, Challenges, and Practical Examples
- Data Governance in Retail: Best Practices, Challenges, and Viable Solutions
- Data Governance in Insurance: Why is it Important and How it Drives Positive Business Outcomes
- Data Governance in Healthcare: Benefits, Framework, and Tooling
- Achieving HIPAA Compliance with Data Governance
Share this article