Forrester on Data Quality: Approach, Challenges, and Best Practices
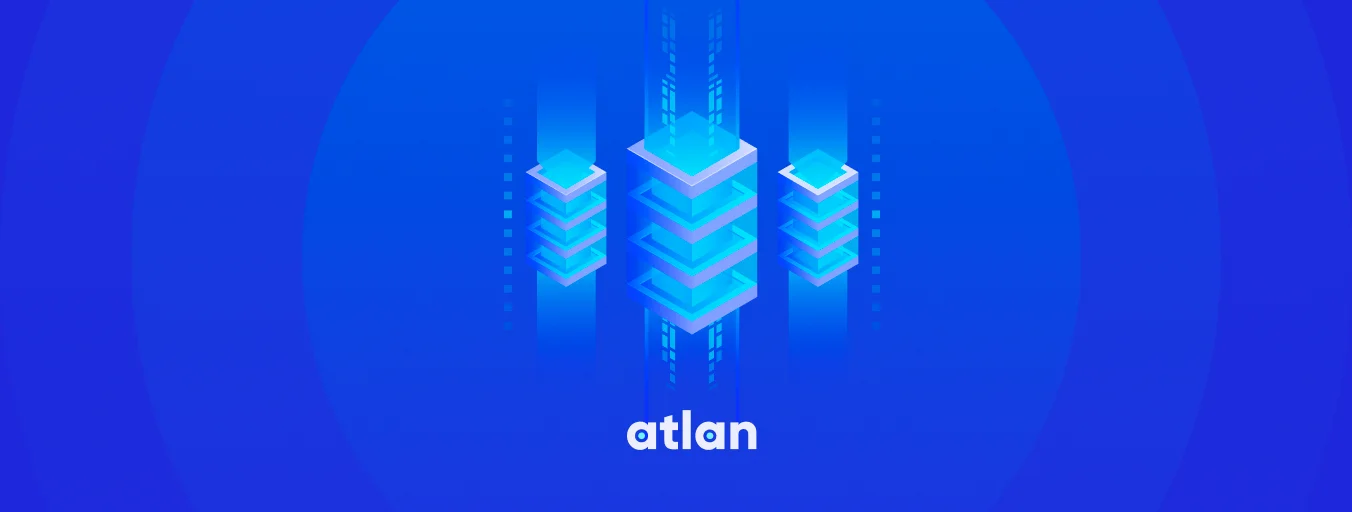
Share this article
Leverage Forrester’s research to understand modern data quality and implement appropriate measures to improve it for your data estate.
Forrester is a research and consulting firm that specializes in offering insights on technology, marketing, customer experience (CX), product, and sales functions. Forrester runs annual surveys of 675,000+ consumers, business leaders, and technology leaders worldwide to get these insights, along with its Forrester Wave™ evaluations.
In this article, we’ll review how Forrester perceives data quality and its role in data governance and decision-making.
Modern data problems require modern solutions - Try Atlan, the data catalog of choice for forward-looking data teams! 👉 Book your demo today
Table of contents
- Forrester on data quality
- How does Forrester define data quality?
- Forrester on the challenges with data quality
- Wrapping up
- Related reads
Forrester on data quality
Forrester views data quality as one of the five pillars of a successful data governance program.
In the report titled “How to Build A Data Governance Practice”, Michele Goetz, VP, Principal Analyst, identifies five pillars of data governance:
- Data quality
- Master data management (MDM)
- Data security
- Information lifecycle management
- Metadata management
Goetz further states how linking the metrics related to data quality with business objectives makes data governance programs more effective.
“A promotional products distributor treated data quality as a symptom, rather than the issue, and looked at business process inefficiency. The company standardized its workflows to remove the inefficiency, leading to better product master quality and lesser errors.”
How does Forrester define data quality?
According to Jayesh Chaurasia, Forrester’s analyst focusing on data quality, master data management, and data governance, data quality involves:
- Ensuring accurate and complete data
- Explaining how data quality differs from data governance
- Highlighting why insights are impossible without quality data
At Atlan, we define data quality as a measure of the condition of data based on factors such as accuracy, completeness, consistency, reliability, and timeliness.
Good data quality can affect everything from decision-making and operational efficiency to regulatory compliance.
Data quality and data governance: Perspectives from Forrester
As mentioned earlier, data quality is essential to good data governance.
Forrester’s best practice report titled “Break Through Data Governance Fatigue” identifies four data governance archetypes — efficiency, risk, growth, and strategy.
Data quality is the starting point for the efficiency archetype. Here’s how a multinational food products company leveraged product data quality to enable digital experiences:
“By aligning priorities of the work, data meaning, and contextual information delivery, the company improved product merchandising and brought products to market faster. Culturally, this meant increasing the data literacy of the marketing staff. [Meanwhile], the technology and operations teams needed to align with product commercialization (or speed-to-market) business processes to enable data collection and management within a new product information management (PIM) environment.”
Read more → Data quality vs. data governance: What’s the difference?
Forrester on the challenges with data quality and how to tackle them
Forrester’s Noel Yuhanna, a VP, Principal Analyst, states that most organizations struggle with data quality.
“Data quality is one of the top challenges, as per recent Forrester survey data.”
According to Simon Daniels, Principal Analyst at Forrester, data decay is a top reason for challenges in data quality management. Data decays as companies and their professionals merge, grow, and change.
Other challenges in maintaining data quality include the diversity of data sources and formats, constraints in terms of time, resources, and budget, scalability issues because of manual processes, and inconsistencies in data handling.
Undertaking data clean-up initiatives to regularly “batch cleanse” and tackle such issues isn’t a sustainable solution.
Instead, you should “build quality into the heart of an organization’s processes and operations,” according to Daniels. It should be a continuous, ongoing process:
“Data quality — like a dog — is for life, not just for the holidays. [It’s] not a one-off exercise… but an ongoing commitment that requires management buy-in and appropriate resourcing.”
At Atlan, we believe that data governance can play a significant role in improving data quality.
Establishing clear data governance policies, creating data quality dashboards, and continuously assessing and monitoring data quality metrics are some of the steps you could take to improve data quality.
Read more → 12 steps to enhancing data quality in your organization
In terms of technology, Yuhanna proposes using generative AI and data fabric to automate the detection of anomalies, perform data cleansing, and validate data in real time.
While automation can help with quality checks and scaling your data quality maintenance efforts, it’s important to start by understanding your data and establishing effective data governance policies and frameworks.
Tooling comes toward the end, once you have these data quality measures and best practices in place.
Also, read → 6 popular open-source data quality tools to know in 2023
Wrapping up
Forrester identifies data quality as a cornerstone of effective data governance programs. Several Forrester analysts highlight its impact on data governance and business process efficiency.
Forrester acknowledges that many organizations encounter challenges in maintaining data quality. The research and consulting firm recommends incorporating data quality into the organizational culture and processes continuously.
Forrester Data Quality: Related reads
- The Forrester Wave™: Enterprise Data Catalog for DataOps, Q2 2022
- Past, present, and future of data catalogs: A masterclass with Forrester
- Forrester changed the way they think about data catalogs
- Automated quality control of data pipelines
- How to Improve Data Quality in 10 Actionable Steps
- Data Quality Measures: Best Practices to Implement
- Data Quality in Data Governance: The Crucial Link that Ensures Data Accuracy and Integrity
- 6 Popular Open Source Data Quality Tools in 2023: Overview, Features & Resources
- Gartner Magic Quadrant for Data Quality: Overview, Capabilities, Criteria
Share this article