Automated Data Catalog: What is It & Why It is the Future?
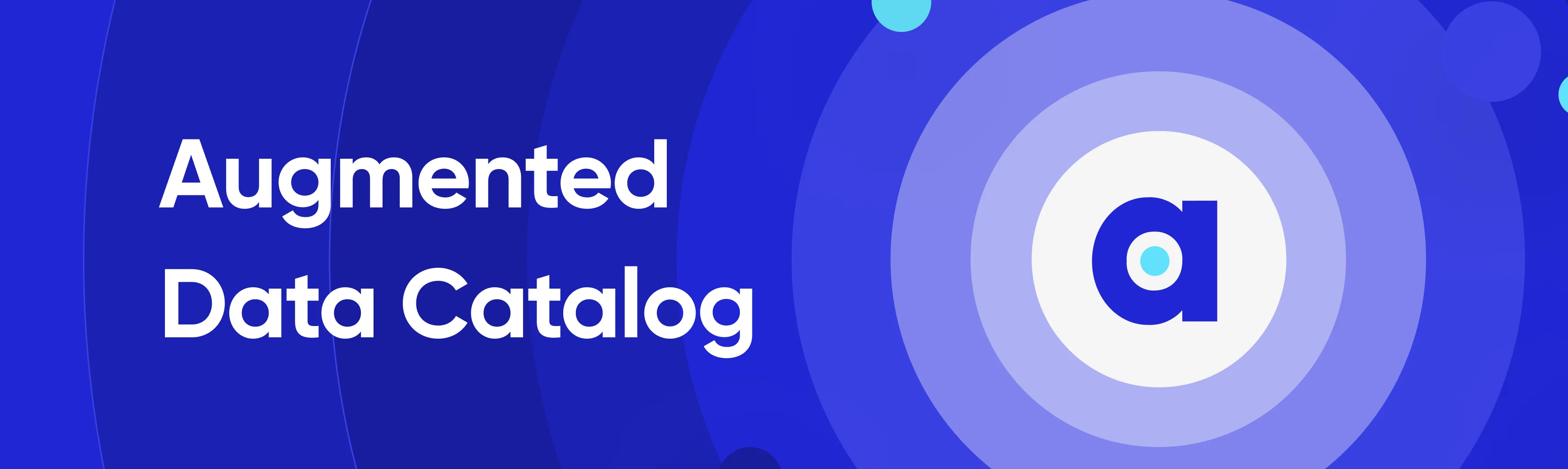
Share this article
Augmented data catalogs are advanced data catalogs that use artificial intelligence and machine learning (ML) to automate and streamline various aspects of metadata management.
These data catalogs are designed to collect, analyze, and share all forms of metadata from across an organization’s data management landscape.
They have a singular goal of turning this passive metadata into active metadata that can inform and automate data management tasks.
In this blog, we will understand what are augmented data catalogs and how artificial intelligence and machine learning technologies are empowering them for efficient data management and collaboration.
Let’s dive in!
Table of contents
- Understanding augmented data catalogs better
- What should you look for in augmented data catalogs?
- How AI and ML empower data catalogs for efficient data management and collaboration
- 6 Reasons why Atlan is the best AI-augmented data catalog tool
- Augmented data catalog: Related reads
Understanding augmented data catalogs better
The phrase “data catalogs” itself predates the augmentation with ML and AI. It refers to a tool used in data management that helps organizations find and manage their data assets. Data catalogs were often part of a larger data management or business intelligence system and served as a centralized resource for data governance and discovery.
The advent of big data and distributed data ecosystems highlighted the need for more advanced solutions, leading to the emergence of ML-augmented or “augmented” data catalogs.
Why “augmented” in augmented data catalogs?
The “augmented” part of the phrase “augmented data catalogs” is likely derived from the concept of augmented intelligence, a design pattern for a human-centered partnership model of people and artificial intelligence (AI) working together to enhance cognitive performance, including learning, decision making, and new experiences.
- The term recognizes the fact that these data catalogs are not merely passive repositories of metadata but are active, ML-driven tools that help automate, inform, and improve data management processes.
- The use of ML and AI in these data catalogs enables them to go beyond traditional metadata management by facilitating the discovery, profiling, and inventorying of distributed metadata within complex data ecosystems such as data lakes.
- Moreover, they can also generate a knowledge graph of relationships between various forms of metadata. This becomes an invaluable asset in informing and even automating parts of data management and integration.
”Augmented data catalogs” originated from the combination of traditional data catalog techniques with advanced machine learning and artificial intelligence capabilities to better manage, utilize, and automate tasks in complex and distributed data landscapes.
What should you look for in augmented data catalogs?
Based on Gartner’s recommendations back in 2019, if you are evaluating augmented data catalogs, it’s crucial to consider certain features and capabilities that differentiate modern data catalogs from traditional metadata management tools.
Here’s a simplified and expanded explanation of the factors to look for in augmented data catalogs:
- Machine learning (ML) integration
- User interface (UI)
- Automated profiling and clustering
- Anomaly Detection and Reporting
- Enhanced Search and Querying
- Collaboration Support
Now, let us look into each of the above factors in brief:
1. Machine Learning (ML) Integration
Look for embedded ML capabilities that can automate the processes of inventorying and curating metadata. This implies that the system can identify and manage data assets without extensive manual intervention, making the process more efficient.
2. User Interface (UI)
The UI should be designed with business users in mind, such as data stewards and analysts. It should be intuitive and easy to use, enabling non-technical users to efficiently access and manage data.
3. Automated profiling and clustering
The catalog should automatically profile data, cluster related datasets together, index data for easy retrieval, and create semantic relationships. This will aid in the understanding and organization of your data.
4. Anomaly detection and reporting
Seek a catalog that can automatically detect and report anomalies, including the detection of Personally Identifiable Information (PII). This helps maintain data accuracy and comply with data protection regulations.
5. Enhanced search and querying
The catalog should offer ML-assisted search and querying capabilities, making it easier for users to find the specific data they need.
6. Collaboration support
The catalog should allow collaboration and interaction with downstream analytics and data science tools through Application Programming Interfaces (APIs). This interoperability with other tools can increase productivity and streamline workflows.
In short, when evaluating an augmented data catalog, look for one that leverages machine learning for automation, focuses on user experience, offers advanced data management features, supports collaboration, and enhances data protection.
How AI and ML empower data catalogs for efficient data management and collaboration
An augmented data catalog is a comprehensive and intelligent data catalog that leverages AI and machine learning (ML) capabilities to automate and enhance various aspects of data cataloging and management. Here’s how AI and ML are augmenting data catalogs:
- Automated documentation
- User-centric design
- Automated profiling and clustering
- Anomaly detection and reporting
- ML-assisted search and querying
- Real-time, on-demand documentation
- Collaboration and interoperability
Let us look into each of the above aspects in brief.
1. Automated documentation
AI enables the automation of the documentation process, which traditionally has been a tedious, manual task. By leveraging AI, hundreds of data assets can be documented in mere minutes, making the process far more efficient and less prone to human error.
2. User-centric design
Augmented data catalogs focus on providing a user interface that’s intuitive for business users, not just IT professionals. This makes it easier for data stewards, analysts, and other non-technical roles to navigate and utilize the catalog, leading to better data accessibility and democratization across the organization.
3. Automated profiling and clustering
AI algorithms automate the processes of data profiling, clustering, and indexing. They can identify semantic relationships within the data, making it easier to understand the data’s context and relevance.
4. Anomaly detection and reporting
Augmented data catalogs use AI to automatically detect and report anomalies, including Personally Identifiable Information (PII). This feature is critical for maintaining data quality, ensuring data compliance, and protecting sensitive information.
5. ML-assisted search and querying
ML can enhance search functionality by learning from user behaviors and preferences, thereby providing more relevant and personalized search results.
6. Real-time, on-demand documentation
One of the unique aspects of AI augmentation is the ability to generate up-to-date documentation on the fly. This contrasts with the traditional approach of maintaining pre-written, static documentation.
7. Collaboration and interoperability
Modern data catalogs provide APIs that facilitate integration with other tools within an organization’s data ecosystem, enabling better collaboration among different analytics and data science tools.
6 Reasons why Atlan is the best AI-augmented data catalog tool
Atlan is a great example of a modern, AI-augmented data catalog tool. Here’s why:
- AI-Driven assistance
- Dynamic and collaborative
- Automated and scalable documentation
- Self-service capabilities
- Continuous updates
- Empowering users
Let us look into each of the above reasons one by one:
1. AI-Driven assistance
Atlan’s AI-driven approach extends across a wide variety of functions, from SQL generation to business term documentation, making it far more powerful than traditional data catalogs. It uses AI to bridge the gap between technical and non-technical users, simplifying tasks like understanding complex SQL transformations and business definitions.
2. Dynamic and collaborative
Atlan promotes collaboration among team members by allowing visibility into questions previously asked by team members. The ability to understand the lineage and schema of a data asset fosters a dynamic environment for data exploration and improves the overall efficiency of a data team.
3. Automated and scalable documentation
Atlan uses AI to auto-generate documentation for business terms and data assets. This feature is particularly useful for organizations with a large volume of data assets, as it drastically reduces the time and effort needed to maintain up-to-date documentation.
4. Self-service capabilities
Atlan’s AI acts as a self-service assistant, offering natural language search for data discovery, helping business users to be more independent and not reliant solely on data analysts. This democratizes data access and allows for more data-driven decision-making across the organization.
5. Continuous updates
One of the key challenges with traditional data catalogs is maintaining them. Atlan addresses this by using AI to ensure the data catalog is always updated, even generating documentation for every new data asset.
6. Empowering users
Atlan empowers all users, regardless of their SQL knowledge, to ask questions and get insights from their data. This aspect democratizes data understanding and fosters a data-driven culture within the organization.
In summary, Atlan serves as a compelling case for an AI-augmented, modern data catalog due to its AI-driven functionality, collaborative features, automated and scalable documentation, self-service capabilities, continuous updates, and its ability to empower all users to interact with data more effectively.
Augmented data catalog: Related reads
- AI Data Catalog: Exploring the Possibilities That Artificial Intelligence Brings to Your Metadata Applications & Data Interactions
- 8 Ways AI-Powered Data Catalogs Save Time Spent on Documentation, Tagging, Querying & More
- Data Catalog: Does Your Business Really Need One?
- Open Source Data Catalog Software: 5 Popular Tools to Consider in 2023
- Enterprise data catalog: Definition, Importance & benefits
- Google Cloud Data Catalog Guide - Everything You Need to Know
- AWS Glue Data Catalog: Architecture, Components, and Crawlers
- Data Catalog Platform: The Key To Future-Proofing Your Data Stack
Share this article