Achieving High Data Quality During Cloud Data Migrations: A 2024 guide!
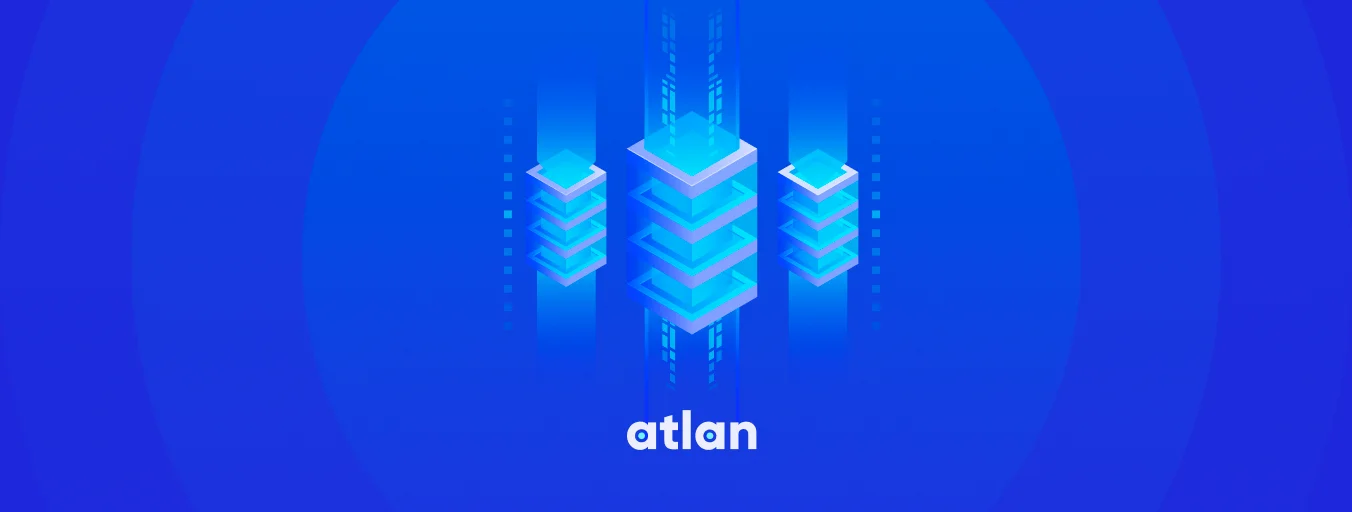
Share this article
High data quality is crucial during cloud data migrations because it ensures the integrity, accuracy, and reliability of the data being transferred.
A successful migration with high-quality data enables organizations to fully leverage the advantages of cloud computing, such as scalability and cost-efficiency, while also improving decision-making and customer satisfaction.
Modern data problems require modern solutions - Try Atlan, the data catalog of choice for forward-looking data teams! 👉 Book your demo today
Migrating poor-quality data not only jeopardizes your organization’s operational efficiency but also risks compliance, security, and ultimately, customer satisfaction. A seamless migration with high data quality at its core is essential for leveraging the full benefits of the cloud.
In this article, we will cover all you need to know for ensuring high data quality during your cloud transition.
Table of contents #
- Data quality in cloud data migration: A quick sneak peek!
- Importance of ensuring data quality in cloud data migration
- How to ensure high-quality data during cloud migrations?
- 10 Challenges in maintaining data quality in cloud migration
- Summarizing it all together
- High data quality during cloud data migrations: Related reads
Data quality in cloud data migration: A quick sneak peek! #
Data quality refers to the accuracy, completeness, reliability, and relevance of data for the purpose it is intended for.
In the context of cloud data migration, data quality is important to ensure that the data transferred from on-premises systems or other clouds to the new cloud environment retains these attributes.
Specific metrics can include:
- Accuracy: Data should be correct and free from errors.
- Completeness: All necessary data should be present, without any missing values or gaps.
- Consistency: Data should not have any discrepancies when compared across different data sets or time periods.
- Accessibility: Data should be easily retrievable and understandable.
- Timeliness: Data should be up-to-date and available when needed.
- Relevance: Only the data that is needed for the specific use case should be migrated.
- Integrity: Relationships between datasets should be maintained after migration.
Importance of ensuring data quality in cloud data migration #
Ensuring data quality during cloud data migration is critical for several reasons:
- Operational efficiency
- Decision-making
- Compliance and risk management
- Customer satisfaction
- Cost-efficiency
- Data utilization
- Business reputation
Let us understand each of them in detail:
1. Operational efficiency #
- Impact: In any organization, smooth operations are vital for success. Poor data quality can disrupt workflows, requiring manual checks and corrections that take time and resources.
- Example: Imagine an inventory system where item names are inconsistent. Employees may waste time searching for items or, worse, ordering new stock because they believe an item is out.
- Solution: Implementing data quality checks and standardizations can drastically reduce these inefficiencies, freeing employees to focus on more value-added tasks.
2. Decision-making #
- Impact: Decision-making processes rely heavily on data analytics. Poor data quality can lead to incorrect conclusions and, consequently, poor decisions.
- Example: If a company uses flawed data to decide which product line to expand, they might invest in something that’s actually underperforming.
- Solution: Consistently validating and cleaning data ensures that decision-makers have accurate and up-to-date information, resulting in better outcomes.
3. Compliance and risk management #
- Impact: For businesses in regulated industries like healthcare, finance, or energy, maintaining data quality isn’t optional; it’s often legally required.
- Example: In healthcare, incorrect patient data can not only result in bad patient care but also in severe legal consequences for the institution.
- Solution: Adopting strict data governance policies and employing data stewards can help maintain the quality of data and ensure compliance with legal requirements.
4. Customer satisfaction #
- Impact: Poor data quality can affect how well a company understands and serves its customers, leading to lower satisfaction and trust.
- Example: If customer contact information is outdated in a CRM system, marketing campaigns might not reach the intended audience, causing missed opportunities and frustration.
- Solution: Regularly updating and validating customer data can significantly improve the quality of customer interactions, building long-term trust and satisfaction.
5. Cost-efficiency #
- Impact: Bad data is expensive to correct and can increase storage costs for redundant or obsolete information.
- Example: Companies might need to hire additional staff or services to clean up poor-quality data, which is far more expensive than maintaining it in the first place.
- Solution: Implementing automated data quality checks at the point of data entry can catch errors before they become expensive problems.
6. Data utilization #
- Impact: Data analytics tools are only as good as the data fed into them. Poor-quality data can render these expensive tools virtually useless.
- Example: An analytics dashboard might show skewed key performance indicators (KPIs) if fed with inaccurate data, making it difficult to derive actionable insights.
- Solution: Data quality should be assessed and ensured before it is used in analytics tools to maximize the return on investment (ROI) on such platforms.
7. Business reputation #
- Impact: Consistently poor data quality can severely damage an organization’s reputation among customers, stakeholders, and even within its own employee base.
- Example: If a financial institution repeatedly makes errors in customer accounts due to bad data, it may gain a reputation for being unreliable.
- Solution: A comprehensive data governance strategy that involves all stakeholders can help prevent data quality issues from tarnishing a company’s reputation.
To resolve data quality issues check the following article → Resolving Data Quality Issues in the Biggest Markets
In summary, high data quality is not merely a technical requirement but a business imperative that affects virtually all aspects of an organization.
How to ensure high-quality data during cloud migration? #
Ensuring high-quality data during cloud migration is a multi-step, iterative process that involves planning, execution, and monitoring. Here’s how to go about it :
Planning phase #
-
Conduct a data audit
- Evaluate your current data landscape.
- Identify the types of data you have, where they reside, and their current quality levels in terms of accuracy, completeness, and consistency.
-
Define data quality metrics
- Define what high-quality data means for your organization.
- Decide on the KPIs (Key Performance Indicators) to measure data quality such as error rates, duplication rates, completeness, etc.
-
Choose the right tools and technology
- Decide on the tools and technology stack for data migration and quality control.
- This could include ETL (Extract, Transform, Load) tools, data quality software, and cloud management tools.
Pre-migration phase #
-
Data cleansing
- Correct erroneous records, remove duplicates, and fill in missing values.
- Validate the integrity of data relationships and hierarchies.
-
Data mapping and transformation
- Create a data mapping document to serve as a guide for how data fields will transfer from the source to the target system.
- Plan any data transformations that may be required.
-
Test migration
- Conduct a test migration with a small subset of data.
- Validate that the data quality metrics defined earlier are met.
Migration phase #
-
Execute migration
- Migrate the data in batches or phases, as planned.
-
Real-time monitoring
- Use real-time monitoring tools to keep track of the migration process.
- Pay attention to the data quality KPIs, intervening if any issues arise.
-
Validation and verification
- After each migration batch or phase, validate that the data meets the quality standards.
- This involves checking that data is accurate, complete, and consistent.
Post-migration phase #
-
Audit and review
- Conduct a thorough review of the migrated data to ensure that it meets all quality metrics.
- Document any lessons learned for future migrations.
-
Ongoing quality monitoring
- Implement data governance and quality assurance mechanisms.
- Use automated tools to continuously monitor data quality, including setting up alerts for anomalies.
-
User training
- Train end-users and data managers on best practices for maintaining data quality in the new cloud environment.
-
Regular updates and maintenance
- Keep your data quality tools up-to-date, and regularly review your quality metrics and KPIs to ensure they are aligned with business goals.
Best practices #
- Involve stakeholders: Keep key stakeholders in the loop during the entire process, from planning to post-migration.
- Documentation: Maintain comprehensive documentation of all processes, from the data audit to the lessons learned post-migration, to serve as a knowledge base for future migrations.
- Automate where possible: Automation can make data quality checks faster, more efficient, and less prone to human error.
- Quality over quantity: Don’t rush the migration process. Take the time to ensure that each phase is completed to the highest quality standards.
By following these detailed steps and best practices, organizations can significantly improve the chances of a successful cloud migration with high-quality data.
10 Challenges in maintaining data quality in cloud migration #
Maintaining data quality during cloud migration poses a number of challenges that can seem overwhelming due to the complexity, scale, and dynamic nature of the process.
Here are some of the most common challenges and reasons why it seems difficult:
1. Heterogeneous data sources #
- Organizations often have data in various formats and structures, stored across multiple platforms.
- Migrating this heterogeneous data into a unified cloud-based system requires complex mapping and transformation, which increases the likelihood of errors and inconsistencies.
2. Data volume and scalability #
- The sheer volume of data to be migrated can be overwhelming.
- Managing large-scale migrations without impacting data quality requires robust infrastructure and meticulous planning. Any oversight can result in significant data quality issues.
3. Complexity of data relationships #
- Enterprise data often has intricate relationships and dependencies that need to be maintained.
- Failure to accurately map and maintain these relationships during migration can result in broken data links, affecting the integrity and reliability of the migrated data.
4. Real-time data changes #
- In many organizations, data is constantly changing due to ongoing business operations.
- Migrating a live system without freezing operations is complex. Capturing changes in real time and reflecting them in the migrated data without errors is a challenging task.
5. Data privacy and security #
- Ensuring data privacy and compliance with regulations like GDPR, HIPAA, etc.
- The migration process may expose sensitive data to vulnerabilities, and the challenge of maintaining compliance while ensuring quality adds another layer of complexity.
6. Skills gap #
- Lack of expertise in cloud migration and data quality management.
- Cloud migration requires specialized knowledge and skills. A lack of expertise can result in mistakes that compromise data quality.
7. Inconsistent data quality metrics #
- Different departments within an organization may have varying definitions and metrics for data quality.
- A unified data quality standard is essential for successful migration. Reconciling these inconsistencies during migration is challenging.
8. Time and resource constraints #
- Data migration is often time-sensitive, and organizations may not have adequate resources dedicated to quality assurance.
- The rush to meet deadlines can result in cutting corners, leading to compromised data quality.
9. Legacy systems #
- Older systems may contain data that is not well-documented or standardized.
- Migrating such data to a cloud environment is challenging because of the lack of metadata and documentation, which are critical for maintaining data quality.
10. Lack of stakeholder alignment #
- Stakeholders across different business units might have varying interests and goals related to the migration.
- Aligning everyone to prioritize data quality can be a complex political and organizational challenge, affecting the overall quality of the migrated data.
Maintaining high data quality during cloud migration is undoubtedly challenging, but the risks of not doing so can have far-reaching impacts on business operations, decision-making, and compliance.
Therefore, it’s crucial to approach these challenges with comprehensive planning, the right tools, and a skilled team.
Summarizing it all together #
Maintaining high data quality during cloud data migrations is not just a recommendation; it’s a necessity for the success of any modern business.
As technology continues to evolve, it’s crucial for organizations to stay ahead of the curve. Adhering to high data quality standards during cloud data migrations is an investment that ensures your data assets remain reliable, secure, and valuable for the long term.
High data quality during cloud data migrations: Related reads #
- Cloud Data Migration: A 10-Step Ultimate Guide
- Data Migration: On-Premise to Cloud - The 10 Steps To Success
- Cloud Migration Data Security Challenges and How To Address Them
- 15 Cloud Migration Challenges to Overcome in 2024
- 8 Essential Strategies to Fast-Track Your Cloud Migration Journey!
- Data Warehouse Migration: The Ultimate Guide in 2024
- Data Quality Measures: Best Practices to Implement
- Data Quality Explained: Causes, Detection, and Fixes
- Data Quality Fundamentals: Why It Matters in 2024!
- A Blueprint for Bulletproof Data Quality Management
- Data Quality Issues? Here Are 6 Ways to Fix Them!
- How to Improve Data Quality
Share this article