What is Data Scrubbing? From Basic Concepts to Techniques!
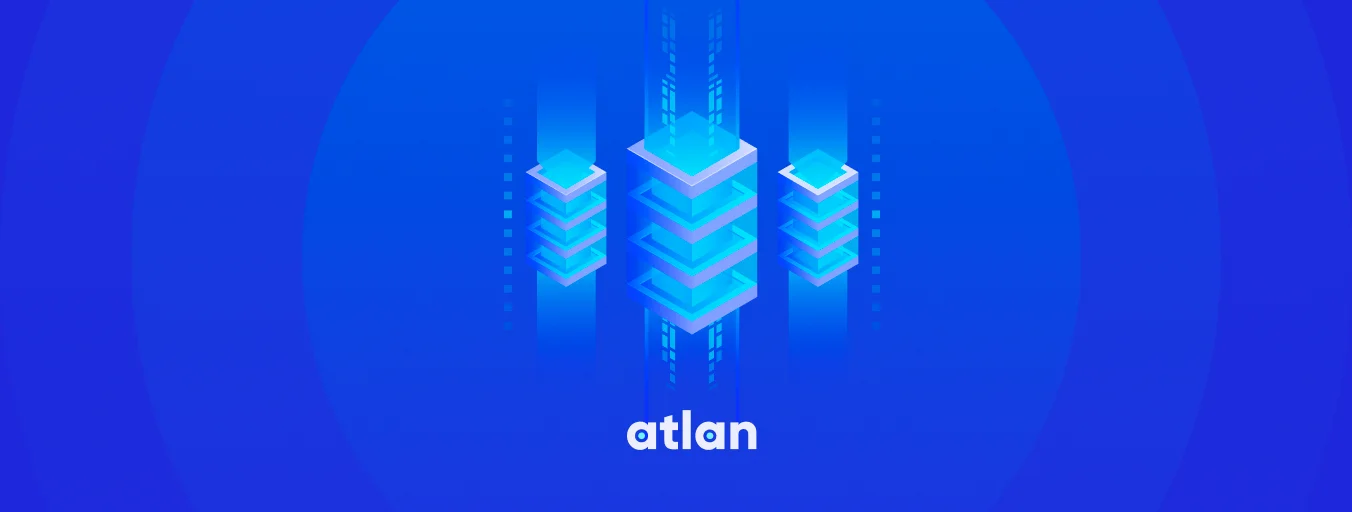
Share this article
In a world drowning in data, 73% of company data remains unanalyzed, often due to its poor quality. At this point, data scrubbing comes into play as a vital solution. It addresses the critical pain point of unreliable data which hinders informed decision-making, operational efficiency, and overall business intelligence.
Data scrubbing boosts data accuracy and consistency, enabling organizations to effectively utilize their data, turning it into a valuable strategic asset.
Data scrubbing, also known as data cleansing, is the meticulous process of detecting and correcting (or removing) corrupt or inaccurate records from a dataset. In an era where data-driven decisions are essential, the importance of data scrubbing cannot be overstated. It’s the crucial first step in ensuring that the data at your fingertips is not just abundant but accurate, reliable, and actionable.
Modern data problems require modern solutions - Try Atlan, the data catalog of choice for forward-looking data teams! 👉 Book your demo today
In this article, we will explore:
- Basic concept of data scrubbing
- An overview of data scrubbing synology
- Data cleansing, cleaning, and scrubbing
- Steps involved in the data scrubbing process
- Challenges faced during data scrubbing
- Benefits that data scrubbing brings to the table
So, let’s dive in!
Table of contents #
- What is data scrubbing?
- Data scrubbing synology
- Data cleansing vs. data cleaning vs. data scrubbing
- What are the techniques involved in data scrubbing?
- What are the steps in the data scrubbing process?
- What are the examples of data scrubbing?
- Benefits of data scrubbing
- Conclusion
- Related reads
What is data scrubbing? #
Data scrubbing is a crucial process in the realm of data management and is essentially the meticulous cleaning of data. It involves sifting through datasets to identify and rectify or remove incorrect, incomplete, or irrelevant information. The objective is to ensure the purity and accuracy of data, which is indispensable for making informed decisions.
In practice, data scrubbing utilizes a variety of techniques and tools to cleanse data. This can range from simple tasks like removing duplicates and correcting typos to more complex challenges such as resolving mismatched data from different sources.
Advanced software and algorithms are often used to automate and refine the process, handling large volumes efficiently and effectively. The importance of data scrubbing cannot be overstated, especially in a data-driven world where quality information is key to success.
By ensuring the integrity of data, it not only enhances the reliability of analytics and reporting but also safeguards against potential errors that could lead to misguided decisions or non-compliance issues. In essence, data scrubbing is the unsung hero ensuring that the foundation of our data-driven actions is solid and trustworthy.
An overview of the data scrubbing synology #
In the complex world of data management, ensuring the integrity and reliability of stored information is essential. This is where data scrubbing synology comes into play.
It’s a sophisticated process utilized in synology NAS (Network Attached Storage) systems, aiming to maintain data accuracy and prevent corruption.
Let’s look into each of the above nuances in brief:
1. Understanding the basics of data scrubbing synology #
Data scrubbing synology refers to the process implemented in synology NAS systems to detect and correct data corruption.
This proactive approach is designed to safeguard data integrity by continuously scanning and repairing corrupted data, ensuring that the information stored remains accurate and reliable.
2. The role of RAID in data scrubbing synology #
RAID (Redundant Array of Independent Disks) plays a crucial role in data scrubbing synology. It provides redundancy, which is essential for data recovery in case of disk failure.
The data scrubbing process works hand in hand with RAID configurations to detect and correct silent data errors, thereby enhancing the resilience of the data storage system.
3. Automated data integrity checks #
One of the standout features of data scrubbing synology is its automated data integrity checks.
The system regularly performs background scans to identify and rectify any discrepancies in the stored data. This automation ensures continuous protection without the need for manual intervention.
4. Impact on performance and how synology balances it #
While data scrubbing is beneficial for data integrity, it can impact system performance. Synology addresses this by optimizing the scrubbing process to minimize performance degradation.
The process is designed to run with low priority, ensuring that the system’s regular operations remain largely unaffected.
5. User-friendly interface and control #
Synology NAS systems are renowned for their user-friendly interface, and this extends to the data scrubbing feature as well.
Users have the flexibility to schedule data scrubbing tasks and monitor the process, making it accessible even for those with limited technical expertise.
6. Addressing silent data corruption #
Silent data corruption, where errors go undetected until it’s too late, is a significant concern in data storage.
Data scrubbing synology tackles this by proactively identifying and rectifying such errors, thereby preventing potential data loss or system failures.
7. Enhancing data longevity and reliability #
By regularly correcting errors and maintaining data integrity, data scrubbing synology plays a vital role in enhancing the longevity and reliability of the stored information.
This is particularly crucial for businesses and individuals who rely heavily on data accuracy for their operations.
8. Scalability and adaptability of the process #
As data storage needs grow, the data scrubbing process in synology NAS systems scales accordingly.
This adaptability ensures that irrespective of the amount of data or the number of disks in use, the data integrity is consistently maintained.
9. Data scrubbing synology as a preventive measure #
In essence, data scrubbing synology serves as a preventive measure, mitigating risks before they escalate into major issues. It’s a testament to the proactive approach in data management, emphasizing prevention over cure.
Data scrubbing synology is more than just a feature; it’s a safeguard, a commitment to data integrity. In an age where data is similar to currency, ensuring its accuracy and reliability is not just an option but a necessity.
Through its automated checks, user-friendly interface, and scalability, data scrubbing synology stands as a guiding light of data reliability, silently working behind the scenes to uphold the sanctity of our digital assets.
Data cleansing vs. data cleaning vs. data scrubbing: 10 Differences between them #
In the complicated world of data management, terms like data cleansing, data cleaning, and data scrubbing are often used interchangeably, leading to confusion. While they share similarities, there are subtle differences that set them apart.
Understanding these distinctions is crucial for any data professional looking to employ the right processes for maintaining data quality.
Let us look at the differences between data cleansing, data cleaning and data scrubbing in a tabular format:
Aspect | Data cleansing | Data cleaning | Data scrubbing |
---|---|---|---|
Definition | Data cleansing involves identifying and correcting inaccuracies and inconsistencies in data to improve its quality. | Data cleaning primarily focuses on removing errors and inconsistencies from data sets. | Data scrubbing goes beyond cleaning, involving validation and reconciliation processes to ensure data accuracy and consistency. |
Scope | Encompasses a broader range of activities, including standardization, validation, and enrichment. | Generally limited to error detection and removal, such as duplicates or incomplete entries. | Involves in-depth analysis, often incorporating algorithms and complex checks to validate data integrity. |
Tools used | Advanced software tools capable of handling data standardization, deduplication, and enrichment. | Basic tools for filtering, sorting, and removing unwanted data. | Sophisticated tools that can perform pattern recognition, anomaly detection, and more. |
Objective | To enhance data usability and reliability by improving overall data quality. | To clean up data sets, making them more usable for specific tasks or analyses. | To ensure the accuracy and consistency of data, especially in critical applications. |
Complexity | Often more complex, requiring a comprehensive approach to address various data quality issues. | Less complex, primarily dealing with surface-level data issues. | Highly complex, involving thorough checks and often automated processes. |
Frequency of application | Typically performed periodically to maintain ongoing data quality. | Can be a one-time process or done as needed. | Often done regularly, especially in systems where data integrity is crucial. |
Outcome | Results in a cleaner, more standardized, and enriched data set. | Produces a cleaner data set, free from obvious errors and inconsistencies. | Ensures data integrity and reliability at a deeper level. |
Importance in decision-making | Crucial for ensuring data-driven decisions are based on accurate and comprehensive data. | Important to ensure decisions are not based on flawed data. | Vital for decisions where data accuracy and consistency are non-negotiable. |
Industry applications | Widely used in marketing, finance, healthcare, and any industry relying on high-quality data. | Common in research, academia, and initial stages of data analysis. | Essential in industries where data integrity is paramount, such as finance, healthcare, and security. |
Typical challenges | Managing data from diverse sources, data standardization, and enrichment. | Identifying and removing duplicates, incomplete data, and basic errors. | Implementing complex validation rules, maintaining data consistency, and automated error correction. |
Understanding the nuances between data cleansing, data cleaning, and data scrubbing is vital for anyone navigating the world of data management. Each process serves a unique purpose, and choosing the right one depends on the specific needs of your data project.
Whether it’s the broad scope of data cleansing, the targeted approach of data cleaning, or the in-depth rigor of data scrubbing, recognizing and utilizing these processes appropriately can significantly elevate the quality and reliability of your data, ultimately leading to more informed decisions and successful outcomes.
What are the techniques involved in data scrubbing? #
Data scrubbing, also known as data cleansing, is a critical process in data management where data is cleaned to improve its quality. This involves identifying and correcting (or removing) errors and inconsistencies from data to enhance its accuracy and reliability. Here are some common techniques involved in data scrubbing:
1. Error detection and correction #
- Identification of anomalies: Recognizing irregularities or deviations in data, such as outliers or unexpected values.
- Correction of errors: Once errors are identified, they are corrected. This might involve rectifying spelling mistakes, incorrect data entries, or resolving inconsistencies.
2. Data validation #
- Rule-based validation: Applying predefined rules to ensure data conforms to specific standards or patterns, like format validations for dates or email addresses.
- Cross-reference with trusted sources: Validating data against reliable external sources or databases to confirm its accuracy.
3. Data standardization #
- Uniform formats: Converting data into a consistent format, which is crucial for fields like dates, phone numbers, and addresses.
- Normalization: Bringing data into a standard format or range, like converting all temperatures to Celsius or all currency to a single standard.
4. De-duplication #
- Identifying duplicates: Locating and removing duplicate records to ensure each data entry is unique.
- Merge or purge: Deciding whether to merge duplicate entries into a single record or purge redundant ones.
5. Data enrichment #
- Adding missing information: Filling in gaps in data, like adding a zip code to an address.
- Enhancing data with external sources: Incorporating additional information from external data sources to provide a more complete picture.
6. Data consolidation #
- Merging data from multiple sources: Combining data from various sources into a single, cohesive dataset.
- Reconciling data variations: Addressing discrepancies in data coming from different sources.
7. Data quality audit #
- Regular audits: Conducting regular audits to assess data quality.
- Monitoring and reporting: Implementing tools to monitor data quality and generating reports for analysis.
8. Missing data handling #
- Imputation: Estimating missing values based on available data.
- Removing or flagging: Deciding whether to remove records with missing data or flagging them for future reference.
9. Data parsing and transformation #
- Parsing: Breaking down data elements into structured formats.
- Data transformation: Converting data into formats suitable for analysis or business processes.
10. Data anonymization #
- Masking Personal Identifiable Information (PII): Hiding or encrypting sensitive data for privacy and compliance.
- Creating synthetic data: Generating non-identifiable data that retains statistical significance for analysis.
These techniques, when applied effectively, can significantly enhance the quality of data, making it a reliable asset for decision-making, analytics, and various business processes.
What are the steps in the data scrubbing process? #
Embarking on the data scrubbing process can be similar to navigating unfamiliar waters for many businesses. This meticulous journey is crucial for ensuring data integrity and usability.
Now, we outline a comprehensive roadmap, broken down into clear, actionable steps. By following this guide, organizations can effectively transform their raw data into a valuable asset that drives informed decisions and strategic actions.
Here are the steps that are involved in the process of data scrubbing:
- Identifying the data sources
- Data auditing
- Defining data quality standards
- Data cleaning
- Data validation
- Data Enrichment
- Data integration
- Data monitoring
- Documentation and reporting
Let’s look into each of the above steps in brief:
1. Identifying the data sources #
The first step in the data scrubbing process is identifying all the sources from which your data is coming. This could include databases, spreadsheets, external data providers, and even manual entries.
Understanding where your data originates is crucial because it sets the stage for the kind of scrubbing techniques you’ll need to utilize. Different sources might require different approaches based on their inherent quality and structure.
2. Data auditing #
Once you’ve pinpointed the sources, the next step is to conduct a thorough data audit. This involves examining the data for errors, inconsistencies, and gaps.
Tools such as data profiling can be handy here, allowing you to assess the quality of your data and understand its current state. Think of this step as a health check-up for your data, identifying potential problems that need addressing.
3. Defining data quality standards #
Before diving into the actual cleaning process, it’s essential to define what good data looks like for your organization. Establish clear data quality standards that your data must meet.
This could include accuracy, completeness, consistency, reliability, and timeliness. These standards will act as a benchmark throughout the data-scrubbing process.
4. Data cleaning #
Here’s where the hands-on work begins. Data cleaning involves removing or correcting incorrect data that doesn’t meet your quality standards.
This might include fixing typos, aligning disparate data formats, removing duplicates, or addressing missing values. Data cleaning is a crucial step in enhancing the overall quality and usability of your data.
5. Data validation #
After cleaning, the data needs to be validated. This step ensures that the data conforms to specific rules or parameters set by your organization.
For instance, you might validate that all phone numbers have the correct number of digits or that email addresses have a valid format. Data validation is about ensuring that the data is not just clean, but also correct and useful.
6. Data Enrichment #
Sometimes, cleaning and validating data isn’t enough. Data enrichment involves adding value to your existing data by incorporating additional relevant information.
This could be appending demographic information to customer data or integrating data from external sources. Enrichment enhances the depth and context of your data, making it more valuable for analysis and decision-making.
7. Data integration #
If you’re dealing with data from multiple sources, integration is key. This step involves combining data from different sources and providing a unified view.
Proper integration ensures that your data is not just clean and enriched, but also cohesive and comprehensive.
8. Data Monitoring #
Data scrubbing isn’t a one-off process; it’s ongoing. Regular monitoring is essential to maintain the quality of your data over time.
Implement systems and protocols for continually auditing and reviewing your data. This proactive approach helps you catch and address any new issues that might arise.
9. Documentation and reporting #
Lastly, document the entire data scrubbing process and report your findings. This documentation should include the techniques used, challenges faced, and improvements made.
It serves as a valuable reference for future data scrubbing initiatives and helps in maintaining transparency and accountability within the organization.
Data scrubbing is a journey that requires thorough planning, execution, and maintenance. By following these nine steps, businesses can navigate this process with confidence, transforming their data into a powerful tool for driving success. Remember, in the world of data, quality is king, and a well-executed data-scrubbing process is your path to reigning supreme.
What are the examples of data scrubbing? #
Data scrubbing examples include:
- Correcting typographical errors: Fixing misspellings in customer names.
- Standardizing data formats: Converting all date entries to a single format (e.g., YYYY-MM-DD).
- Removing duplicates: Eliminating duplicate customer records in a database.
- Validating data entries: Ensuring all email addresses in a list follow a proper format.
- Filling missing values: Adding missing zip codes in an address list based on city and state information.
- Data De-duplication: Merging multiple entries of the same customer into one.
- Error detection and cleaning: Identifying and correcting outliers in sales data.
- Data enrichment: Adding area codes to phone numbers using external databases.
- Converting data types: Changing a text field of numerical values into a numeric data type.
- Anonymizing data: Masking personal identifiers in a dataset for privacy protection.
11 Transformative benefits of data scrubbing #
In the world of data management, data scrubbing stands out as a transformative process, turning chaotic datasets into clear, actionable insights. Beyond mere cleanliness, the benefits of data scrubbing ripple across various aspects of an organization, driving efficiency, accuracy, and informed decision-making.
Here are 11 transformative benefits of data scrubbing:
- Enhanced data accuracy
- Better decision-making
- Increased efficiency
- Compliance and risk management
- Improved customer relationships
- Enhanced data integration
- Cost savings
- Scalability
- Competitive advantage
- Enhanced data usability
- Foster a data-driven culture
Let’s look into each of the above benefits in brief.
1. Enhanced data accuracy #
At its core, data scrubbing significantly improves the accuracy of data. By removing errors, inconsistencies, and inaccuracies, data scrubbing ensures that the information at hand is reliable.
This accuracy is crucial for businesses, as it forms the foundation for all subsequent data-driven decisions and strategies.
2. Better decision-making #
Accurate data leads to better decision-making. With data scrubbing, organizations can trust their datasets, making informed decisions based on precise and up-to-date information.
This can lead to more effective strategies, improved performance, and a competitive edge in the market.
3. Increased efficiency #
Data scrubbing streamlines data management processes. By eliminating redundant and incorrect data, it reduces the time and resources needed to handle datasets.
This efficiency allows teams to focus on analysis and strategic tasks rather than getting bogged down in data cleaning.
4. Compliance and risk management #
In today’s regulatory environment, compliance is key. Data scrubbing helps organizations meet legal and regulatory requirements, especially regarding data privacy and protection.
By ensuring data is accurate and handled correctly, businesses can mitigate risks and avoid potential legal issues.
5. Improved customer relationships #
Data scrubbing has a direct impact on customer relationships. Clean, accurate customer data enables businesses to better understand their clients’ needs, preferences, and behaviors.
This understanding leads to more personalized services, improved customer experiences, and, ultimately, stronger relationships.
6. Enhanced data integration #
Integrating data from multiple sources is smoother with data scrubbing. By ensuring data consistency and compatibility, scrubbed data can be seamlessly merged, providing a comprehensive view across various datasets.
This integration is crucial for holistic analysis and insights.
7. Cost savings #
Though data scrubbing requires an initial investment, it can lead to significant cost savings in the long run.
By reducing errors, businesses can avoid costly mistakes and redundant efforts. Additionally, clean data reduces the need for additional resources, leading to more efficient operations.
8. Scalability #
As organizations grow, so does their data. Data scrubbing ensures that datasets are manageable and scalable.
Clean, well-organized data can be easily expanded and adapted to changing business needs, supporting growth and evolution.
9. Competitive advantage #
In a data-driven world, having clean, reliable data can be a competitive advantage.
Data scrubbing allows businesses to gain deeper insights, identify trends, and make swift, informed decisions, keeping them ahead in the competitive landscape.
10. Enhanced data usability #
Data scrubbing enhances the overall usability of data. By ensuring data is clean, well-structured, and free from errors, it becomes more accessible and user-friendly for analysis and reporting.
This usability is key for teams across the organization to leverage data effectively.
11. Foster a data-driven culture #
Lastly, data scrubbing plays a pivotal role in fostering a data-driven culture within an organization.
When teams consistently work with high-quality data, it builds trust in data-driven processes and encourages data-centric approaches to problem-solving and innovation.
The benefits of data scrubbing are far-reaching and multifaceted. From improving accuracy and efficiency to fostering a data-driven culture, the impacts of this process are profound and pervasive.
As organizations navigate the complex seas of data management, data scrubbing stands as a beacon, guiding them towards clearer, more informed, and more successful futures. In the end, the meticulous efforts of data scrubbing pay off, not just in cleaner data, but in a transformed, more effective organization.
Conclusion #
Throughout this article, we’ve delved deep into the world of data scrubbing. We’ve uncovered its essence, explored its various facets, and unveiled the critical differences it has with similar processes.
The journey from understanding the fundamental steps to recognizing its challenges and benefits highlights the significant role data scrubbing plays in shaping data integrity.
The transformative benefits of data scrubbing, from enhanced decision-making to improved data quality, are evident. While the challenges are prominent, they are not unbeatable. With the right tools, strategies, and understanding, organizations can navigate these hurdles effectively, harnessing the full potential of their data.
As we close this chapter on data scrubbing, remember that it’s not just a process but a journey toward data excellence. Whether you are a seasoned data expert or just beginning to grapple with the complexities of data management, data scrubbing stands as a keystone in realizing the true value of data in this information-driven age.
Data scrubbing: Related reads #
- Data Cleaning, Management, and Tagging: The Best Practices
- Data Observability vs Data Cleansing: 5 Points to Differentiate
- Data Quality is Everyone’s Problem, but Who is Responsible?
- Ebook Launch: The Ultimate Guide to Basic Data Cleaning
- A Blueprint for Bulletproof Data Quality Management
- How To Improve Data Quality In 12 Actionable Steps?
Share this article