Data Validation vs Data Quality: 12 Key Differences
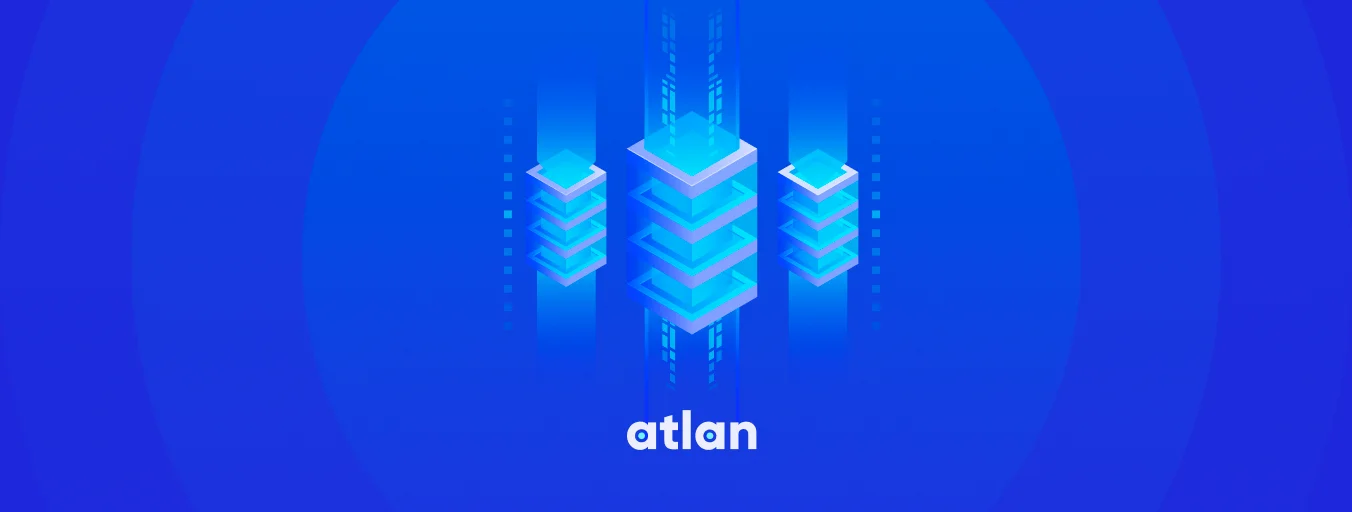
Share this article
According to Forbes, a staggering 95% of businesses cite the need to manage unstructured data as a problem for their business. In this data-driven era, the distinction between data validation and data quality is not just an academic pursuit—it’s a vital business necessity.
Data validation is the process of ensuring that data input meets predefined criteria and standards before it’s processed, acting as a crucial checkpoint for accuracy. On the other hand, data quality is a broader concept that encompasses the overall completeness, consistency, and reliability of data within a system.
Understanding the nuances of ‘data validation vs data quality’ is key to harnessing the power of your data effectively, ensuring it’s not just abundant but also actionable and reliable.
Modern data problems require modern solutions - Try Atlan, the data catalog of choice for forward-looking data teams! 👉 Book your demo today
In this article, we will look into:
- Basics of data validation and data quality
- The key differences
- Benefits
- Best practices
- Conclusion and related reads
Let’s dive in!
Table of contents #
- What is data validation?
- What is data quality?
- Data validation vs data quality: 12 Key differences
- Data validation vs data quality: 3 Real world examples
- 4 Key benefits of data validation & data quality
- Data validation vs data quality: 5 Best practices
- Summarizing it all together
- Data validation vs data quality: Related reads
What is data validation? #
Data validation is a critical process used to ensure the accuracy and quality of data. It involves the verification of data against a set of rules or parameters to confirm its correctness and appropriateness for a specific purpose.
This process is fundamental in maintaining data integrity, crucial for businesses and organizations relying on data-driven decision-making. The mechanics of data validation vary based on the data’s nature and intended use.
It ranges from basic checks, such as ensuring a field isn’t empty or confirming that entered dates are realistic, to more complex validations, like ensuring consistency across different data sets or verifying data against predefined formats or criteria. This process is key in preventing data errors and maintaining the credibility of data systems.
In our data-driven era, the role of data validation is more significant than ever. It not only enhances the reliability of data analysis but also plays a vital part in compliance and security, especially in industries with strict data standards.
Effective data validation helps organizations avoid costly mistakes, making it an indispensable part of modern data management strategies.
What is data quality? #
Data quality refers to the measure of data’s condition, often defined by its accuracy, completeness, reliability, and relevance. High-quality data is essential for effective decision-making and operational efficiency in various industries.
It encompasses the ability of the data to serve its intended purpose, providing a reliable foundation for analytics, reporting, and business intelligence. In essence, data quality assesses whether data is fit for use in the specific context of a business or organization.
The concept of data quality is multifaceted, encompassing several key dimensions that collectively define its effectiveness and usability. These dimensions include accuracy, completeness, consistency, and timeliness, each playing a vital role in assessing the overall quality of data.
- Accuracy: This dimension ensures that the data accurately represents real-world entities or events. Accurate data correctly mirrors what it is intended to depict, free from errors and inaccuracies that could mislead or result in incorrect conclusions.
- Completeness: Completeness is about having all necessary data present, with minimal gaps. It involves ensuring that no crucial piece of information is missing, which could otherwise compromise the data’s ability to serve its intended purpose.
- Consistency: Consistency refers to maintaining uniformity across various data sets and sources. It’s about ensuring that data remains consistent in format, structure, and value across different systems, contributing to its reliability and ease of use.
- Timeliness: This dimension deals with the data being up-to-date and relevant to the current context. Timeliness is crucial, especially in fast-paced environments where outdated information can lead to missed opportunities or erroneous decisions.
Maintaining high data quality is crucial in today’s data-centric business environment. Poor data quality can lead to incorrect decisions, inefficiency, and potential financial losses.
Therefore, businesses invest in processes and technologies to continually assess and enhance the quality of their data, ensuring it remains a valuable asset for strategic decision-making and day-to-day operations.
Data validation vs data quality: 12 Key differences #
Data validation and data quality are two critical aspects of data management, each playing a distinct role in ensuring the integrity and usefulness of data in an organization. While they are often mentioned together, their differences are significant and worth understanding.
Below is a table highlighting twelve key differences between data validation vs data quality.
Aspect | Data validation | Data quality |
---|---|---|
Definition | Process of checking data against predefined rules or criteria to ensure correctness. | Overall measurement of data's condition and suitability for use, based on specific attributes. |
Focus area | Ensuring the data format, type, and value meet specific standards or rules. | Assessing data across multiple dimensions like accuracy, completeness, and relevance. |
Scope | Often operational, focused on individual data entries or transactions. | Broader, considering the entire dataset or database's quality. |
Methodology | Involves specific checks like format validation, range checking, and data type verification. | Encompasses a wider range of evaluations like data profiling, data cleansing, and monitoring. |
Objective | To verify that data entered into a system is correct and valid. | To ensure that the overall dataset is fit for its intended purpose. |
Process stage | Typically performed at the point of data entry or acquisition. | Ongoing process, often carried out throughout the data lifecycle. |
Tools used | Form validation tools, data entry interfaces with validation rules. | Data quality software, comprehensive data management tools. |
Outcome | Clean, error-free individual data points. | A dataset that is reliable, accurate, and useful for decision-making. |
Error identification | Focuses on immediate errors in data entry or transmission. | Identifies systemic issues affecting data integrity and usability. |
Responsibility | Often lies with data entry personnel or automated data collection systems. | Involves data analysts, IT teams, and data stewards for broader oversight. |
Measurement | Based on the percentage of data passing validation rules. | Measured through data quality metrics like error rates, completeness scores. |
Impact | Prevents the entry of incorrect or irrelevant data into systems. | Influences the overall effectiveness of data in supporting business processes and decisions. |
These distinctions highlight how data validation and data quality, while interconnected, address different aspects of data management, each playing a unique role in ensuring the effectiveness of a data-driven approach in organizations.
Data validation vs data quality: 3 Real world examples #
Data validation and data quality are crucial concepts in managing information, especially in today’s data-driven world. Understanding the difference between the two and how they manifest in real-life scenarios can be enlightening.
Data validation examples #
Here are some examples of data validations are:
- Online form submission
- E-commerce transactions
- Database importing
Let’s explore the examples of data validations in detail.
1. Online form submission #
When you fill out an online form, say for a loan application, data validation is at work when it prompts you for missing or incorrectly formatted information.
For instance, if you forget to fill in your date of birth, or if your email address is missing the ‘@’ symbol, the form won’t submit until these errors are corrected. This is data validation ensuring the correctness of data at the point of entry.
2. E-commerce transactions #
In an e-commerce platform, when you enter your credit card details, data validation checks occur. It verifies whether the card number matches the standard format and if the expiration date is valid.
These checks prevent transaction errors and potential fraud.
3. Database importing #
Suppose a company is importing customer data into their CRM system. Data validation will check if the phone numbers conform to the expected format and if email addresses are valid.
This step prevents the entry of incorrect data right from the start.
Data quality examples #
Here are some of the real world data quality examples:
- Customer relationship management system
- Healthcare records management
- Financial reporting
Let’s look into the examples of data quality in detail.
1. Customer relationship management system #
Over time, a CRM system might accumulate outdated or duplicate customer records. Data quality processes involve cleaning this data – updating records, removing duplicates, and ensuring that each customer’s information is accurate and complete.
This results in a reliable database that the sales and marketing team can use for effective customer engagement.
2. Healthcare records management #
In a hospital’s database, maintaining high data quality is crucial. It involves ensuring patient records are complete, accurate, and consistently formatted across various departments. This ensures that doctors and nurses have access to reliable information, which is vital for effective patient care.
3. Financial reporting #
For a financial analyst preparing quarterly reports, data quality is paramount. They need to ensure that the financial data from various departments is not only accurate and complete but also consistent and timely.
This involves regular checks and balances to ensure the data reflects the true financial position of the company, which is essential for stakeholders and regulatory compliance.
In summary, data validation is about making sure the data entered into a system is correct right from the start, while data quality is an ongoing process that ensures the entire database is accurate, complete, consistent, and relevant at all times. Both are integral to maintaining the integrity of an organization’s data.
4 Key benefits of data validation & data quality #
In the realm of data management, the terms ‘data validation’ and ‘data quality’ often surface. While they are interconnected, understanding their distinct benefits is crucial for any organization aiming to harness the power of accurate and reliable data.
Benefits of data validation #
Here are the benefits of data validation include:
- Error prevention at the source
- Enhanced data integrity
- Improved user experience
- Compliance and security
Let’s delve into the benefits of data validations.
1. Error prevention at the source #
Data validation acts as the first line of defense against errors. By setting rules for data entry, it ensures that incorrect or inappropriate data doesn’t enter the system in the first place. This proactive approach saves time and resources that would otherwise be spent correcting errors.
2. Enhanced data integrity #
By enforcing specific formats and criteria, data validation maintains the integrity of the data. For example, ensuring that a zip code field only accepts numbers or that an email address is in a valid format prevents inconsistencies that could disrupt data analysis.
3. Improved user experience #
When data validation is implemented in user-facing applications, it can significantly enhance user experience. Clear guidelines and immediate feedback on data entry help users correct their inputs in real-time, leading to more successful interactions with the application.
4. Compliance and security #
In industries where data accuracy is not just important but mandated by law (like healthcare or finance), data validation is a key tool for compliance. It also plays a role in security, as it can prevent malicious data from entering the system.
Benefits of data quality #
Here are the benefits of data quality include:
- Reliable decision-making
- Efficiency in operations
- Enhanced analytical power
- Customer satisfaction
Let’s look into the benefits of data quality in detail.
1. Reliable decision-making #
High-quality data is a cornerstone of informed decision-making. When data is accurate, complete, and consistent, organizations can rely on it to make strategic decisions. This trust in data quality can be a significant competitive advantage.
2. Efficiency in operations #
Good data quality means less time is spent hunting down and correcting errors, leading to more efficient operations. When data is clean and organized, employees can find what they need quickly and trust the information they are using.
3. Enhanced analytical power #
For data analytics to be effective, the underlying data must be of high quality. Clean, well-maintained data sets lead to more accurate and insightful analytics, which can uncover trends and opportunities that might otherwise remain hidden.
4. Customer satisfaction #
High-quality data can lead to better customer experiences. For example, accurate customer contact information ensures that communications reach the intended recipient. Similarly, up-to-date product data can enhance customer satisfaction in e-commerce settings.
In the comparison of data validation vs data quality, both play vital roles in the data management ecosystem. Data validation is about preventing erroneous data at the point of entry, while data quality is an ongoing process of maintaining the utility and accuracy of the data over time.
Data validation vs data quality: 5 Best practices #
The importance of ‘data validation vs data quality’ cannot be overstated. Both are critical in ensuring that the data an organization collects and utilizes is accurate, reliable, and useful.
Let’s explore some of the best practices that can help in achieving high standards in both data validation and data quality.
Best practices for data validation #
Some of the key practices of data validation include:
- Define clear validation rules
- Implement real-time validation
- Use robust validation tools
- Regularly update validation criteria
- Educate users about validation standards
Let’s understand the best practices of data validation in detail.
1. Define clear validation rules #
Establish specific and clear rules for data entry. This includes setting the correct data type, format, and range for each data field. For instance, dates should be in a consistent format, and numerical fields should reject alphabetic characters.
2. Implement real-time validation #
Incorporate real-time feedback mechanisms in data entry systems. This helps users correct errors immediately, enhancing data accuracy at the point of entry.
3. Use robust validation tools #
Employ comprehensive data validation tools that can handle complex validation rules. These tools should be capable of validating data against not only format and type but also against business rules and logic.
4. Regularly update validation criteria #
As business needs evolve, so should your validation criteria. Regularly review and update these rules to ensure they remain relevant and effective.
5. Educate users about validation standards #
Train users entering data on the importance of validation standards. A well-informed user is less likely to make errors during data entry.
Best practices for data quality #
Some of the key practices of data quality include:
- Continuous data quality assessment
- Data cleansing
- Establish data quality metrics
- Foster a culture of data stewardship
- Leverage technology for data quality management
Let’s look into the best practices of data validation in detail.
1. Continuous data quality assessment #
Regularly audit your data for quality. This includes checking for accuracy, completeness, consistency, and relevancy. Tools and processes for these assessments should be part of the data management strategy.
2. Data cleansing #
Implement processes for correcting or removing incorrect, incomplete, or irrelevant data. Data cleansing should be an ongoing activity to maintain high data quality.
3. Establish data quality metrics #
Define clear metrics to measure data quality. These metrics should align with the organization’s goals and help in tracking the effectiveness of data quality initiatives.
4. Foster a culture of data stewardship #
Encourage a culture where data is valued and managed with care. Assign data stewards who are responsible for maintaining the quality of data in their respective areas.
5. Leverage technology for data quality management #
Utilize advanced data quality management tools that can automate many aspects of data quality maintenance, such as identifying duplicates, standardizing data formats, and validating data against external sources.
By adopting best practices in data validation, organizations can prevent erroneous data from entering their systems. Concurrently, best practices in data quality ensure that the data remains accurate, consistent, and useful over time.
Balancing these two aspects effectively results in reliable data that can drive insightful decisions and strategies, giving organizations a competitive edge in the data-driven world.
Summarizing it all together #
In conclusion, this blog has explored the critical concepts of data validation and data quality, their distinctions, and their interplay in the realm of data management. We’ve unpacked the essence of data validation as the gatekeeper of data entry, and data quality as the ongoing custodian of data’s accuracy and utility.
Through real-world examples, we’ve illustrated their applications and emphasized their individual benefits. The 12 key differences highlighted offer a clear demarcation, aiding understanding and implementation.
Lastly, the outlined best practices provide actionable insights for effectively managing both aspects. Remember, in the data-driven landscape of today, mastering both data validation and data quality is not just advantageous but essential for any organization’s success.
Data validation vs data quality: Related reads #
- Data Quality Measures: Best Practices to Implement
- Data Validation Demystified: Ensuring Accuracy in Every Byte
- Data Quality Fundamentals: Why It Matters in 2023!
- Data Quality Strategy: 10 Steps to Excellence!
- How To Improve Data Quality In 12 Actionable Steps?
- Data Quality and Observability: Key Differences & Relationships!
- What is Data Validity: 9 Reasons Why It’s Important!
Share this article