Data Quality and Observability: Key Differences & Relationships!
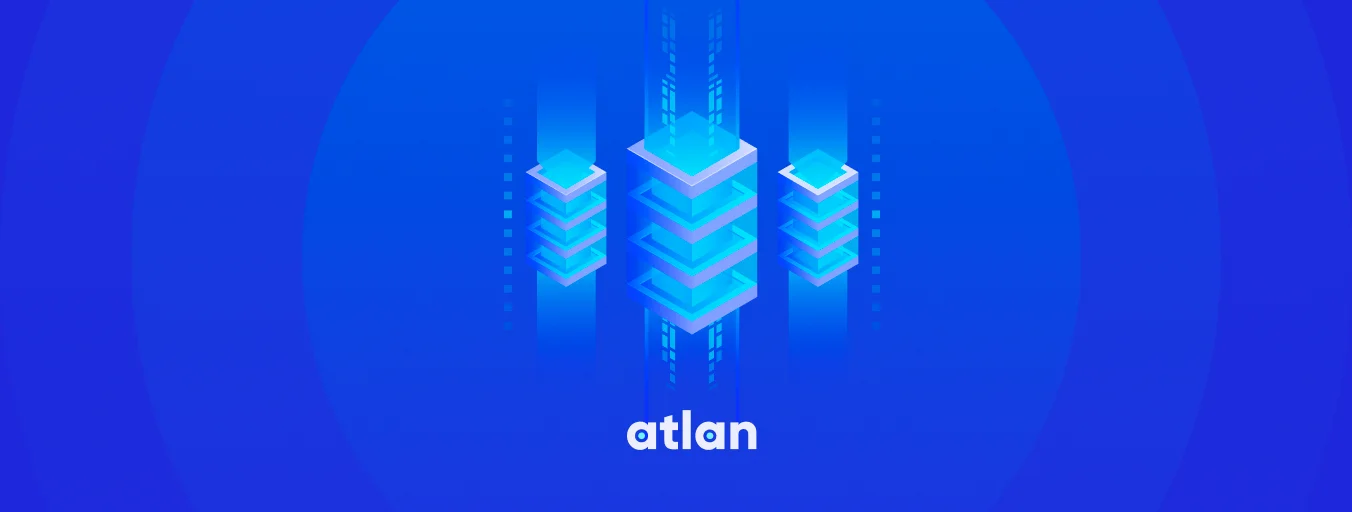
Share this article
Data quality refers to the accuracy, completeness, consistency, and timeliness of data. On the other hand, data observability enables the monitoring and investigation of systems and data pipelines to develop an understanding of data health and performance. Both work in synergy to ensure trust in data.
In today’s data-driven world, organizations rely heavily on data to make critical business decisions and operations. However, the value extracted from data is highly dependent on its quality and reliability. This is where the dual facets of data quality and observability become crucial.
Modern data problems require modern solutions - Try Atlan, the data catalog of choice for forward-looking data teams! 👉 Book your demo today
As data volumes grow exponentially, organizations must invest in both capabilities. Data quality and observability provide the checks and balances required in the modern data landscape. This discussion explores their connection and joint impact in enabling data maturity.
Let’s dive in to understand more about the differences and importance of data quality and observability.
Table of contents #
- Data quality and observability: Understanding the definitions
- Data quality and observability - How are they related?
- Data quality and observability - How are they different?
- How to implement data quality and observability in your organization in 6 steps?
- In conclusion
- Related reads
Data quality and observability: Understanding the definitions #
Data quality and data observability are two crucial concepts in the field of data management and analytics. Understanding their definitions and distinctions is essential for organizations to maintain reliable and valuable data assets. Let’s explore these concepts in detail:
1. Data quality #
Data quality refers to the measure of the reliability, accuracy, completeness, consistency, and overall trustworthiness of data. It focuses on the characteristics that determine the fitness of data for its intended use. Here are key components of data quality:
-
Accuracy: Data should be free from errors and represent the real-world entities it is supposed to describe. Inaccurate data can lead to incorrect decisions.
-
Completeness: Data should be comprehensive and not have missing values or gaps. Incomplete data can hinder analysis and decision-making.
-
Consistency: Data should be internally consistent, meaning that it doesn’t contradict itself within the same dataset. Inconsistencies can arise from data integration or collection issues.
-
Timeliness: Data should be up-to-date and relevant for the intended purpose. Stale data can result in outdated insights.
-
Relevance: Data should be pertinent to the questions or problems being addressed. Irrelevant data adds noise and reduces the usefulness of the dataset.
-
Reliability: Data should be trustworthy and sourced from credible and authoritative sources. Unreliable data can lead to misinformed decisions.
-
Validity: Data should conform to the defined data schema, structure, and constraints. Invalid data may not fit the expected format.
-
Integrity: Data should maintain its integrity, meaning it should not be altered or tampered with without proper authorization.
Ensuring data quality often involves data profiling, cleansing, validation, and monitoring processes. Data quality analysts and data stewards are responsible for maintaining and improving data quality within organizations.
2. Data observability #
Data observability is a relatively newer concept that focuses on real-time monitoring and understanding of data pipelines and workflows. It is often associated with the management of data in complex, distributed systems, such as data lakes, data warehouses, and cloud-based data platforms. Here are key components of data observability:
-
Monitoring: Data observability involves continuous monitoring of data pipelines, data transformations, and data flows. It tracks data lineage, data dependencies, and data transformations in real-time.
-
Alerting: Data observability tools and systems generate alerts and notifications when data issues or anomalies are detected. These alerts help data engineers and data operators quickly respond to problems.
-
Visibility: It provides visibility into the entire data ecosystem, allowing organizations to understand how data moves and transforms within their infrastructure. This visibility aids in troubleshooting and optimization.
-
Metadata: Metadata is crucial in data observability. It includes information about data sources, data schemas, transformations, and lineage. Metadata helps in understanding and managing data better.
-
Drift detection: Data observability can detect data drift, which is a significant concern when dealing with machine learning models and data pipelines. Drift detection ensures that model inputs and outputs remain consistent over time.
-
Performance metrics: Data observability tools often capture performance metrics related to data processing and data pipeline execution. These metrics help in optimizing data workflows for efficiency.
-
Root cause analysis: When issues arise, data observability tools can assist in identifying the root causes of problems, enabling faster resolution.
In summary, data quality focuses on the intrinsic characteristics of data, ensuring it is accurate, complete, and reliable.
Data observability, on the other hand, focuses on monitoring and understanding data pipelines and workflows in real-time, allowing organizations to detect and respond to issues promptly.
Both concepts are vital for maintaining high-quality and trustworthy data assets in today’s data-driven organizations.
To know more on data observability, check out the article -> Data Observability: Definition, Key Elements, and Business Benefits
Data quality and observability - How are they related? #
The realms of data quality and observability converge to create a comprehensive framework for ensuring the reliability, accuracy, and effectiveness of an organization’s data-driven initiatives. Understanding the synergy between these two concepts is pivotal in navigating the complexities of modern data landscapes.
The following factors are when both data quality and data observability work together for optimal results:
- Shared focus on accuracy
- Real-time monitoring for quality assurance
- Proactive Issue detection enhancing quality
- Root cause analysis and data Integrity
- Holistic data excellence through collaboration
Let us understand these points in detail.
1. Shared focus on accuracy #
Both data quality and data observability center on the accuracy and trustworthiness of data. Data quality addresses the intrinsic attributes of data, ensuring that it is consistent, complete, and accurate.
On the other hand, data observability complements this by actively monitoring data flows and processes, maintaining data accuracy in real time. Together, they uphold the sanctity of data across its lifecycle.
2. Real-time monitoring for quality assurance #
Data quality is often assessed through batch-based processes, which might not catch immediate issues. Data observability fills this gap by introducing real-time monitoring, enabling organizations to identify discrepancies or anomalies as soon as they emerge.
This instantaneous vigilance enhances data quality by swiftly addressing issues before they escalate.
3. Proactive issue detection enhancing quality #
Data observability’s proactive issue detection aligns seamlessly with data quality goals. By detecting anomalies and deviations early, organizations can prevent data quality degradation.
Addressing data issues before they impact decision-making or downstream processes ensures that data remains trustworthy and fit for use.
4. Root cause analysis and data integrity #
Both data quality and data observability incorporate root cause analysis as a critical practice. In the realm of data quality, root cause analysis helps identify the reasons behind inconsistencies or inaccuracies.
Data observability’s root cause analysis aids in pinpointing the sources of anomalies, contributing to maintaining the integrity of data streams and processes.
5. Holistic data excellence through collaboration #
The convergence of data quality and observability underscores the importance of cross-functional collaboration.
Data engineers, data scientists, analysts, and business leaders collaborate to define data quality standards, monitor data health, and ensure adherence to best practices. This collaborative effort ensures that data is not only accurate but also aligned with business objectives.
Data quality theory provides a robust framework that enables organizations to harness the power of accurate and reliable data. By understanding and implementing the dimensions of data quality, ensuring data’s fitness for specific purposes, utilizing data profiling and cleansing techniques, and committing to continuous improvement, organizations can establish data as a strategic asset.
In a world where data drives innovation and decision-making, adhering to data quality theory empowers organizations to navigate the complexities of the digital age with confidence and foresight.
Data quality and observability - How are they different? #
In the landscape of data management, two critical concepts, data observability, and data quality, play distinctive roles in ensuring accurate, reliable, and valuable data.
Below is a comparative analysis that illuminates the differences between data observability and data quality. Understanding these distinctions is essential for organizations aiming to optimize their data strategies and operations.
Aspect | Data Observability | Data Quality |
---|---|---|
Focus | Real-time monitoring of data pipelines and processes. | Intrinsic attributes and standards of data. |
Objective | Proactively detect anomalies and deviations. | Enhance and maintain data's reliability and attributes. |
Execution Timing | Continuous monitoring throughout the data lifecycle. | Occurs during data profiling, validation, and transformation. |
Methodology | Monitoring for real-time insights. | Assessment and improvement of data attributes. |
Primary Benefit | Early issue detection to maintain data health. | Ensures data is trustworthy, accurate, and fit for purpose. |
Scope | Monitoring the entire data pipeline and processes. | Focuses on data attributes like accuracy, completeness, consistency. |
Impact | Minimizes operational and decision-making disruptions. | Ensures accurate analysis, informed decisions, and reliable insights. |
Dynamic Nature | Real-time insights into data behavior and health. | Enhances data's value by maintaining its intrinsic attributes. |
In the pursuit of leveraging data as a strategic asset, organizations deploy methodologies to ensure data’s reliability and utility. Data quality and observability are two such methodologies, each addressing different dimensions of data management.
In essence, while data quality concentrates on maintaining data’s inherent attributes, data observability emphasizes real-time vigilance and proactive detection of issues within data processes.
Both practices are essential for comprehensive data management, but they address different facets of data reliability and effectiveness. Recognizing their differences empowers organizations to optimize their data strategies and uphold the data’s strategic value.
How to implement data quality and observability in your organization in 6 steps? #
Implementing data quality and observability practices within your organization requires a systematic approach that combines technical expertise, strategic alignment, and cross-functional collaboration.
Below is a comprehensive guide outlining the steps to successfully implement data quality and observability initiatives:
- Assessment and strategy
- Technical infrastructure
- Data profiling and cleansing
- Real-time monitoring and alert
- Collaboration and training
- Continuous improvement
Let us understand these steps in detail:
Step 1: Initiating data quality and observability implementation #
Understanding organizational goals: Begin by aligning data quality and observability initiatives with your organization’s strategic objectives. Recognize how these practices contribute to informed decision-making, operational efficiency, and innovation.
Identifying data criticality: Determine which data sets are most crucial for your organization’s operations and decision-making. Focus your efforts on these critical data assets to maximize impact.
Defining key metrics and KPIs: Establish measurable metrics and key performance indicators (KPIs) that reflect data quality and observability. These metrics will guide your implementation progress and measure the effectiveness of your initiatives.
Step 2: Building technical infrastructure #
Choosing data quality tools: Select appropriate data quality tools that align with your organization’s technology stack. These tools should facilitate data profiling, validation, cleansing, and monitoring.
Selecting observability solutions: Evaluate and choose observability solutions that provide real-time insights into data pipelines, processes, and system behavior. Consider solutions that integrate seamlessly with your existing architecture.
Integrating monitoring and testing: Integrate data quality checks and observability monitoring into your data workflows. Ensure that these checks are automated and seamlessly embedded in your data processes.
Step 3: Data profiling and cleansing #
Profiling data sources: Perform comprehensive data profiling to understand the characteristics and anomalies present in your data. This step provides insights into potential quality issues.
Identifying data anomalies: Use data profiling results to identify anomalies, inconsistencies, and inaccuracies within your data. This step lays the foundation for effective data cleansing.
Establishing cleansing workflows: Develop workflows and processes for data cleansing based on the anomalies identified. Implement automated data cleansing routines to ensure data accuracy.
Step 4: Real-time monitoring and alerts #
Defining data health indicators: Define key indicators that signify data health and quality. These indicators could include accuracy rates, completeness percentages, and consistency metrics.
Setting up real-time monitoring: Implement real-time monitoring tools that continuously track data flows, processes, and system behavior. These tools provide immediate insights into any deviations from expected data behavior.
Configuring automated alerts: Configure automated alerts that trigger when data anomalies or quality issues are detected. These alerts ensure prompt responses and proactive issue resolution.
Step 5: Collaboration and training #
Forming cross-functional teams: Establish cross-functional teams that include data engineers, data scientists, analysts, and business stakeholders. Collaborative efforts enhance the effectiveness of data quality and observability initiatives.
Providing training and awareness: Offer training sessions to educate team members about data quality and observability practices. Foster a culture of data excellence by raising awareness about the importance of these initiatives.
Establishing communication channels: Set up communication channels for reporting data quality issues and sharing observability insights. Effective communication ensures that data-related concerns are addressed promptly.
Step 6: Continuous improvement #
Regular data audits: Conduct regular data audits to assess the effectiveness of your data quality and observability initiatives. Use the insights gained to refine your strategies and processes.
Iterative refinement: Continuously refine your data quality and observability practices based on feedback and lessons learned. Adapt to evolving data challenges and business requirements.
Incorporating feedback: Incorporate feedback from various stakeholders to enhance your data quality and observability approaches. Collaboration and feedback ensure that your initiatives remain relevant and effective over time.
In conclusion #
Data quality and data observability are two sides of the same coin when it comes to achieving data excellence. While data quality focuses on the inherent attributes of data, data observability provides the real-time vigilance needed to maintain these attributes.
By embracing both concepts, organizations can elevate their data-driven strategies, making informed decisions with confidence and agility in a data-rich landscape.
Data quality and observability: Related reads #
- Data Quality Explained : Causes, Detection, and Fixes
- 9 Components to Build the Best Data Quality Framework
- How To Improve Data Quality In 12 Actionable Steps?
- Data Quality Measures : Best Practices to Implement
- 6 Popular Open Source Data Quality Tools To Know in 2023: Overview, Features & Resources
- Data Quality in Data Governance : The Crucial Link That Ensures Data Accuracy and Integrity
- Data Observability : Definition, Key Elements, and Business Benefits
- Data Observability vs Data Testing : 6 Points to Differentiate
- How Data Observability & Data Catalog Are Better Together
Share this article