8 Ways to Tackle Data Governance Adoption Challenges
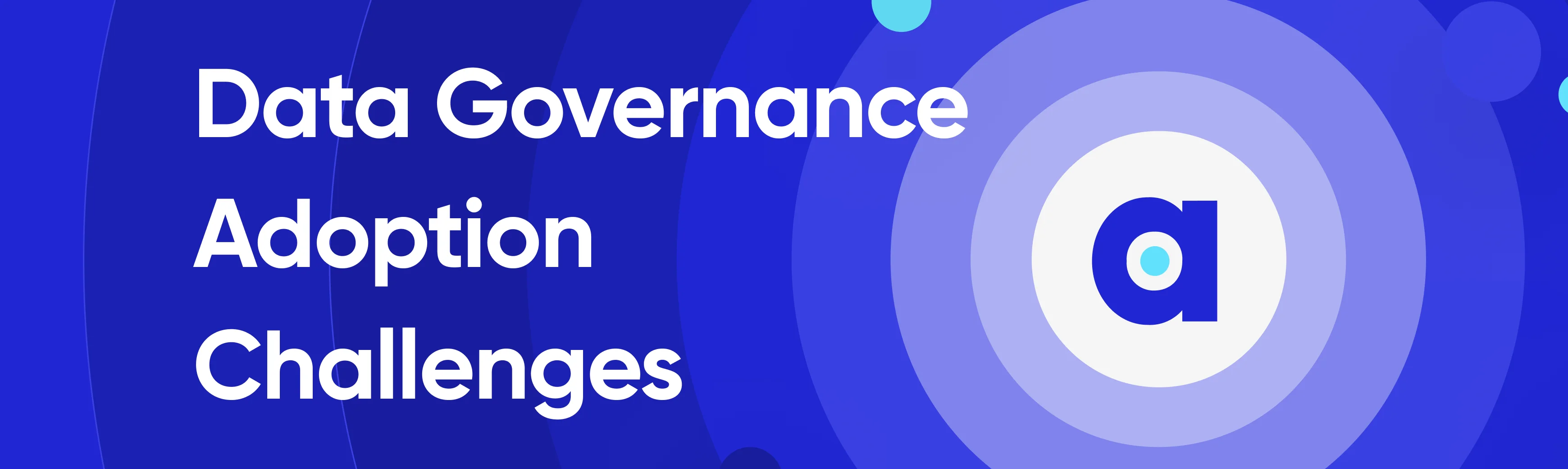
Share this article
Data governance adoption plays a pivotal role in ensuring the effective management, security, and utilization of critical information. However, despite conventional efforts, many organizations encounter hurdles that hinder the successful adoption of data governance methodologies.
These challenges can lead data governance teams on a long, bumpy, and less-than-enjoyable journey, jeopardizing the long-term success of their programs.
At the heart of this complex issue lie the diverse and unique barriers that impede adoption, making it a formidable challenge to overcome.
In this article, we delve into the essence of data governance adoption, identify the challenges faced by data-driven companies, and present valuable insights on how to overcome these obstacles. This will help you realize the true potential of data governance and unlock new vistas of growth and efficiency.
Let’s dive in!
Table of contents #
- Data governance adoption challenges and implications
- Why is data governance adoption essential for businesses?
- How to overcome data governance adoption challenges?
- Data governance adoption challenges and approaches
- Summarizing it all together
- Related reads
10 Data governance adoption challenges and implications: What modern businesses struggle against #
Data governance adoption challenges can be multifaceted and vary from one organization to another. However, some common obstacles and their impacts that many companies encounter include:
- Lack of data governance culture
- Unclear data ownership and accountability
- Inadequate leadership support
- Insufficient resources and budget
- Complex regulatory environment
- Data quality issues
- Resistance to change
- Lack of awareness and education
- Technical integration challenges
- Measuring success and ROI
Let us understand each of the above challenges in detail:
1. Lack of data governance culture #
Building a data-driven culture is essential for successful data governance adoption. However, organizations often struggle to instill a culture where data is considered a strategic asset, and its management and governance are prioritized.
Implication: Fragmented data management practices hinder collaboration and data sharing among different departments. This can result in duplicated efforts, conflicting data, and inefficiencies, leading to suboptimal decision-making.
2. Unclear data ownership and accountability #
Without clear data ownership and accountability, it becomes challenging to define who is responsible for data quality, security, and compliance.
Implication: Inadequate data security measures can result in data breaches, leaks, and cyber-attacks, compromising sensitive information. The consequences include financial losses, legal liabilities, and significant harm to the organization’s reputation.
3. Inadequate leadership support #
Strong leadership support is crucial for driving data governance initiatives forward.
Implication: Without top-level executive support, data governance initiatives may lack sufficient funding, authority, and organizational focus. This can lead to incomplete or ineffective implementation of data governance policies and procedures.
4. Insufficient resources and budget #
Data governance implementation demands adequate resources, including skilled personnel, technology, and financial investments.
Implication: Insufficient resources, such as skilled data professionals and modern data infrastructure, can limit the scope and effectiveness of data governance efforts. This may lead to compromised data security, quality, and compliance adherence.
5. Complex regulatory environment #
Complying with data protection regulations, such as GDPR or CCPA, can be challenging and time-consuming. Organizations need to navigate a complex regulatory landscape, which might differ across regions and industries, adding further complexities to data governance adoption.
Implication: Failure to comply with data privacy regulations can result in severe financial penalties, legal consequences, and reputational damage. Non-compliance also erodes customer trust and loyalty, impacting the organization’s bottom line.
6. Data quality issues #
Poor data quality can undermine trust in data and hinder decision-making processes. Ensuring data accuracy, completeness, and consistency is critical, but data governance teams often face challenges in identifying and rectifying data quality issues.
Implication: Poor data quality undermines the reliability of insights derived from the data. This can lead to faulty analyses, incorrect conclusions, and misguided business strategies, potentially harming the organization’s competitiveness.
7. Resistance to change #
Employees may resist adopting new data governance policies, practices, and tools due to fear of increased workloads, unfamiliarity, or lack of understanding about the benefits. Overcoming resistance to change requires effective communication and training.
Implication: Employee resistance to data governance initiatives slows down adoption and implementation. This resistance may stem from fear of change, lack of understanding, or concerns about job roles. This prevents the organization from harnessing the full potential of data-driven insights.
8. Lack of awareness and education #
Many stakeholders may not fully grasp the significance of data governance or understand how it impacts their day-to-day tasks and overall business goals. Raising awareness and providing education about the importance of data governance is essential to foster its adoption.
Implication: Low data literacy levels across the organization impede the ability to leverage data for decision-making. Employees may struggle to interpret data accurately, leading to missed insights and subpar strategic planning.
9. Technical integration challenges #
Integrating data governance into existing IT infrastructure can be complicated, especially in organizations with legacy systems and a fragmented data landscape. Compatibility issues and the need for seamless integration can slow down the adoption process.
Implication: Inflexible data governance frameworks can hinder the organization’s ability to adapt to changing business needs, expanding datasets, and emerging technologies. This may result in data management bottlenecks and missed growth opportunities.
10. Measuring success and ROI #
Quantifying the return on investment (ROI) of data governance initiatives can be challenging. Without tangible metrics to measure success, it becomes difficult to demonstrate the value of data governance to key stakeholders.
Implication: Difficulty in quantifying the direct ROI of data governance initiatives may lead some stakeholders to question the value of investing in data governance. This skepticism can hinder long-term commitment and support for data governance efforts.
But, why is data governance adoption essential for businesses? #
Data governance adoption is essential in a business because it provides the framework and guidelines for effectively managing, securing, and utilizing the organization’s data assets. In today’s data-driven world, businesses generate and collect massive volumes of data from various sources, including customers, operations, and external environments. Without proper governance, this data can become fragmented, unreliable, and vulnerable to security breaches, leading to suboptimal decision-making and increased risks.
By fostering a culture of data literacy and collaboration, data governance encourages employees to embrace data-driven practices, enabling them to extract valuable insights and drive innovation. It also promotes scalability and adaptability, allowing businesses to efficiently handle expanding datasets and adapt to technological advancements.
Overall, data governance adoption is crucial for modern businesses as it :
- Instills confidence in data quality
- Enhances data security
- Ensures regulatory compliance
- Drives strategic decision-making
With an effective data governance framework in place, businesses can unlock the full potential of their data assets, gain a competitive edge in the market, and thrive in an increasingly data-centric landscape.
How to overcome data governance adoption challenges? #
Overcoming the challenges faced in data governance adoption requires a comprehensive and proactive approach.
Here are eight ways to tackle data governance adoption challenges:
- Cultivate a data governance culture
- Clarify data ownership and accountability
- Address resistance to change
- Improve data quality
- Navigate the complex regulatory environment
- Increase awareness and education
- Overcome technical integration challenges
- Measure success and ROI
Let’s explore each one in detail.
1. Cultivate a data governance culture #
- Lead by example: Encourage leadership to demonstrate their commitment to data governance practices and set a precedent for others to follow.
- Communicate the importance: Educate employees about the significance of data governance in achieving business objectives and how it contributes to their individual roles and responsibilities.
- Establish rewards and recognition: Incentivize data governance adherence by recognizing and rewarding teams and individuals who actively participate in data governance initiatives.
2. Clarify data ownership and accountability #
- Define roles and responsibilities: Clearly outline the roles and responsibilities of data owners, stewards, and custodians to establish accountability and streamline decision-making processes.
- Encourage collaboration: Foster a culture of teamwork and collaboration to ensure data governance responsibilities are shared and understood across teams and departments.
3. Address resistance to change #
- Engage stakeholders early: Involve key stakeholders from the beginning of the data governance implementation to gain their buy-in and address concerns proactively.
- Highlight benefits: Emphasize the positive impact of data governance on efficiency, decision-making, and compliance to alleviate fears of increased workload.
4. Improve data quality #
- Implement data quality controls: Set up data validation, cleansing, and enrichment processes to maintain high data quality standards.
- Conduct regular audits: Periodically audit data sources and records to identify and resolve data quality issues promptly.
5. Navigate the complex regulatory environment #
- Establish a compliance framework: Develop policies and procedures that align with relevant data protection and privacy regulations, ensuring the organization’s practices remain up-to-date and compliant.
- Engage legal experts: Work with legal professionals who specialize in data protection to interpret and implement regulations effectively.
6. Increase awareness and education #
- Conduct training sessions: Host regular workshops and training sessions to educate employees about data governance principles, best practices, and the potential impact on their work.
- Promote data literacy: Foster a data-literate workforce by encouraging employees to understand and interpret data effectively.
7. Overcome technical integration challenges #
- Invest in data integration tools: Utilize data integration platforms that can harmonize data from various sources and provide a unified view for data governance efforts.
- Standardize data formats: Implement standardized data formats and definitions to streamline integration and improve data consistency.
8. Measure success and ROI #
- Define clear KPIs: Identify key performance indicators that align with data governance objectives and demonstrate the value it brings to the organization.
- Monitor progress: Continuously track and analyze data governance initiatives’ outcomes to evaluate their effectiveness and make data-driven improvements.
By adopting these strategies, organizations can effectively address the challenges associated with data governance adoption. Moreover, it is essential to remember that data governance is an ongoing process that requires continuous monitoring, evaluation, and improvement to ensure its long-term success and contribution to organizational growth and success.
Data governance adoption challenges and approaches: Explained with examples #
Data governance is essential for ensuring data quality, security, compliance, and maximizing the value of data assets. However, the road to successful data governance implementation is often fraught with obstacles that can impede progress and hinder data-driven decision-making.
To shed light on these challenges and demonstrate how real-life organizations have tackled them, we delve into some compelling examples of data governance adoption. These real-life cases offer valuable insights into the approaches used to overcome common hurdles, ensuring data governance success.
#1 Data governance adoption challenges in banks #
One example of a data governance adoption challenge in the banking industry is “Data privacy compliance”
Banks are custodians of vast amounts of personal and financial data of their customers. Ensuring compliance with data privacy regulations is critical to protect customer information and maintain their trust.
However, banks have often struggled to implement a cohesive data governance framework that addressed data privacy compliance effectively. This has been mainly due to the complexity of managing data across various systems and departments, each with its own set of data practices.
Here’s how banks could solve this challenge:
- Cross-functional collaboration: Banks need to recognize the need for cross-functional collaboration among IT, legal, compliance, and business departments to develop a comprehensive data governance strategy. Regular meetings and workshops need to happen to identify potential privacy risks and compliance gaps.
- Data mapping and inventory: Banks need to conduct a thorough data mapping exercise to identify all the data elements and their sources, including customer data collected and processed across different systems. This helps in understanding the data flow and the areas that required special attention for privacy compliance.
- Data classification and access controls: A bank’s data can be classified based on sensitivity levels and implemented access controls accordingly. This approach restricts access to sensitive data only to authorized personnel, reducing the risk of data breaches and unauthorized disclosures.
- Data privacy training: To promote data literacy and awareness, banks can conduct data privacy training for all employees. This training should cover the principles of data privacy, the importance of compliance, and the consequences of data mishandling.
- Privacy impact assessments: Banks need to perform privacy impact assessments (PIAs) on new projects and data processing activities to evaluate potential privacy risks. PIAs helped identify and mitigate risks at an early stage, ensuring compliance throughout the project lifecycle.
- Data retention and deletion policies: Banks can establish clear data retention and deletion policies aligned with regulatory requirements. This helps manage the data lifecycle effectively, reducing the risk of retaining data longer than necessary.
- Data protection officer (DPO): Banks need to appoint a Data Protection Officer responsible for overseeing data governance efforts and ensuring compliance with data privacy regulations. The DPO should act as a central point of contact for privacy-related queries and concerns.
By adopting these measures and building a strong data governance framework, banks can successfully address the data privacy compliance challenges. The approach facilitated a unified understanding of data practices, enhanced data security, and ensures the protection of customer data.
#2 Data governance adoption challenges in healthcare #
One example of a data governance adoption challenge in the healthcare industry is “Data Interoperability”
Healthcare organizations typically deal with a multitude of disparate systems, each collecting and storing patient data in different formats and standards. However, ensuring seamless data interoperability and integration across these systems poses a significant challenge.
Without efficient data governance practices, healthcare providers may struggle to access and share patient information accurately and securely. This leads to potential medical errors, inefficiencies, and compromised patient care.
Here’s how healthcare companies could solve this challenge:
A healthcare network might face data interoperability challenges and may seek to implement a robust data governance approach to improve the exchange of patient information among its various systems. This includes electronic health record (EHR) platforms, billing systems, and medical imaging databases.
- Data standardization: The healthcare network company needs to work on standardizing data elements and terminologies across its different systems. This involves adopting widely recognized industry standards, such as Health Level Seven (HL7) and LOINC (Logical Observation Identifiers Names and Codes), to ensure consistency and semantic interoperability.
- Data mapping and integration: The healthcare network can conduct a comprehensive data mapping exercise to identify key data elements and their respective sources in each system. Using integration tools and middleware, they can develop interfaces and APIs to enable seamless data exchange between systems.
- Data quality assessment: Data governance efforts must include implementing data quality checks and validations at various points of data integration. This ensures that the shared patient data is accurate, complete, and free from errors - thereby reducing the risk of misinformation and clinical decision errors.
- Patient consent management: The healthcare organization can implement a consent management system to ensure that patient data is only shared with other healthcare providers or entities with explicit patient consent. This approach addresses privacy concerns while enabling data exchange for improved care coordination.
- Governance committee: The organization can establish a data governance committee comprising representatives from different departments, including IT, medical, legal, and compliance. This committee must oversee the data governance strategy, addressed challenges, and make decisions regarding data-sharing policies and procedures.
- Data security measures: To maintain data security during interoperability, the healthcare network needs to implement robust encryption, access controls, and audit trails. They must also regularly conduct security assessments and vulnerability testing to identify and address potential threats.
- Training and education: To promote data literacy and compliance with data governance policies, the organization needs to provide comprehensive training to staff members involved in data handling and sharing. This training must emphasize the importance of data governance and the ethical use of patient information.
Through these data governance initiatives, the healthcare network can successfully tackle the data interoperability challenge. It can enable a seamless flow of patient information across systems, improving care coordination, and patient outcomes. Additionally, the organization’s commitment to data governance ensures compliance with data privacy regulations, safeguarding patient confidentiality and trust.
Summarizing it all together #
Data governance adoption becomes increasingly crucial for unlocking the full potential of the data assets. While challenges may arise during the implementation, organizations must approach data governance with a strategic mindset and a commitment to building a data-driven culture. Addressing the complexity and cost through careful planning and incremental implementation can pave the way for successful adoption.
The risks of not having a robust data governance framework are significant. Data quality issues, regulatory non-compliance, security breaches, and missed opportunities can undermine business performance and lead to financial and reputational damage. By proactively embracing data governance, businesses can ensure data integrity, leverage data-driven insights, and build trust with customers and stakeholders.
In the dynamic landscape of 2023, data governance emerges not only as a necessity but also as a strategic enabler. Embracing this challenge head-on will position businesses for success, empowering them to harness the full potential of their data and thrive in an increasingly data-centric world.
Data governance adoption challenges: Related reads #
- What is Data Governance? Its Importance, Principles & How to Get Started?
- Key Objectives of Data Governance: How Should You Think About Them?
- Data Governance Framework — Examples, Templates, Standards, Best Practices & How to Create One?
- Data Governance and Compliance: Act of Checks & Balances
- How to implement data governance? Steps, Prerequisites, Essential Factors & Business Case
- How to Improve Data Governance? Steps, Tips & Template
- 7 Steps to Simplify Data Governance for Your Entire Organization
- Snowflake Data Governance — Features, Frameworks & Best Practices
- Automated Data Governance: How Does It Help You Manage Access, Security & More at Scale?
- Enterprise Data Governance — Basics, Strategy, Key Challenges, Benefits & Best Practices
Share this article