How Data Observability & Data Catalog Are Better Together
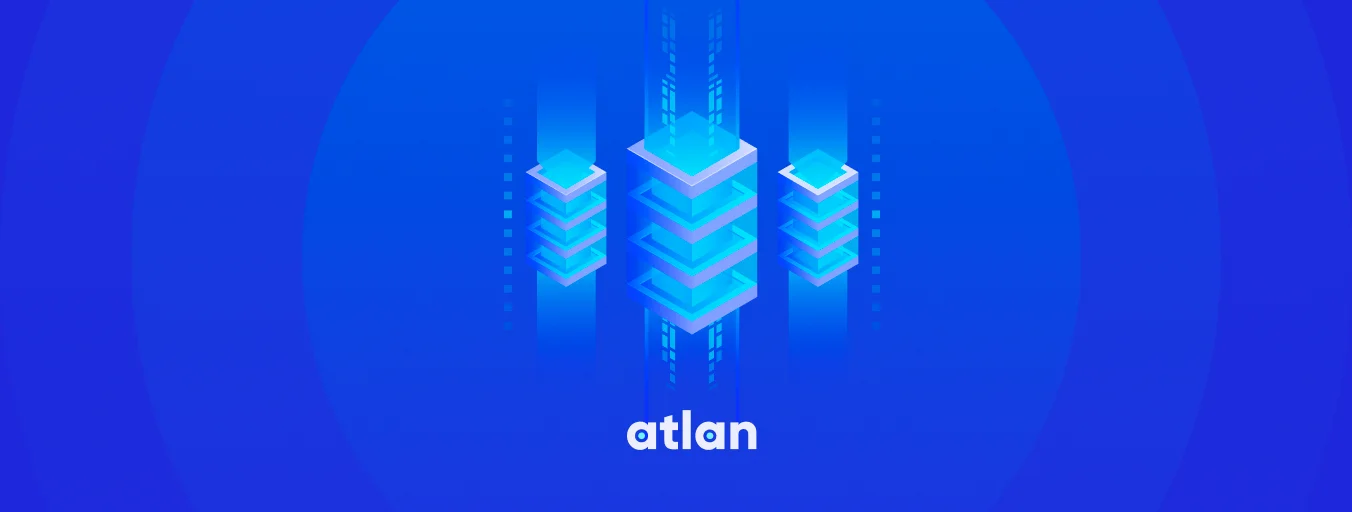
Share this article
The concepts of data observability and data catalog have emerged as twin pillars of efficient data management. While individually powerful, their true potential shines when these two concepts are seamlessly intertwined.
In this article, we delve into the compelling partnership between data observability and data catalog. Let us unravel the synergies between data observability and data catalog.
Table of contents #
- Data observability and data catalog: Understanding the basics
- How is data observability improving data cataloging?
- Data observability and data catalog: Which is more critical?
- Data observability and data catalog: Explained with example
- Summary
- Related reads
Data observability and data catalog: Understanding the basics #
As organizations continue to grapple with the complexities of harnessing data for decision-making and innovation, understanding the fundamentals of these concepts becomes paramount.
In this section, we delve into the core principles of data observability and data catalog, uncovering their distinct roles and synergies. Let us start with data observability:
What is data observability? #
Data observability refers to the practice of monitoring, measuring, and gaining insights into the behaviour, performance, and quality of data as it flows through various processes, pipelines, and systems.
It ensures that data pipelines are reliable, efficient, and meet the organization’s expectations. Data observability is particularly important in data-intensive environments where multiple data sources, transformations, and destinations are involved.
What are the key features of data observability? #
- Real-time monitoring
Data observability provides real-time insights into the health and performance of data pipelines, helping organizations identify and address issues as they occur.
- Anomaly detection
Observability tools can identify anomalies, errors, and discrepancies in data flows, allowing for proactive troubleshooting.
- Data lineage tracking
Data observability solutions can track data lineage in real time, enabling a comprehensive understanding of how data moves through the organization.
- Quality assurance
By monitoring data quality metrics, organizations can ensure that data meets predefined quality standards and rectify deviations promptly.
- Performance optimization
Data observability helps identify bottlenecks and performance issues in data pipelines, enabling optimization for better efficiency.
- Compliance and security
Observability tools can monitor data access patterns, ensuring that data usage adheres to compliance requirements and security policies.
What is a data catalog? #
A data catalog is a centralized repository that serves as a comprehensive inventory of an organization’s data assets. It provides a structured and organized way to document, manage, and discover various data sources, datasets, and metadata.
The primary purpose of a data catalog is to make data more accessible, understandable, and usable for individuals across the organization. It helps users, such as data analysts, scientists, and business stakeholders, find relevant data quickly and understand its context, quality, and usage.
What are the key features of data catalog? #
- Metadata management
A data catalog stores metadata, which includes information about data assets such as their source, structure, definitions, data lineage, ownership, and usage.
- Data discovery
Users can search for and discover datasets based on keywords, attributes, and tags, making it easier to locate the data they need.
- Data lineage
Data lineage diagrams show how data flows through various stages, transformations, and processes, helping users understand how data is transformed and used.
- Collaboration
Data catalogs facilitate collaboration among data users by allowing them to comment, rate, and share insights about specific datasets.
- Data governance
Data catalogs play a role in data governance by enforcing data access policies, ensuring compliance, and maintaining data quality standards.
- Decision-making
By providing a clear view of available data assets, a data catalog enables informed decision-making based on accurate and relevant information.
In summary, a data catalog is a repository that helps organize and manage data assets, while data observability focuses on monitoring and gaining insights into the behavior and quality of data pipelines.
Both concepts are essential in a data-driven organization, as they contribute to improved data management, decision-making, and overall data reliability. Integrating data observability with a data catalog enhances data discoverability, quality assurance, governance, and agility.
How is data observability improving data cataloging? #
Data observability and data cataloging are two distinct but highly complementary concepts that together can significantly enhance an organization’s data management and decision-making capabilities.
Let’s explore how data observability is beneficial for data cataloging and how they work hand in hand:
- Enhanced data discovery and accessibility
- Data quality and reliability
- Data governance and compliance
- Continuous improvement
Let us understand each of them in detail:
1. Enhanced data discovery and accessibility #
Data cataloging:
- A data catalog acts as a centralized repository of metadata that describes various datasets, their sources, definitions, and relationships.
- It helps users discover relevant data assets and understand their context.
Data observability:
- Data observability involves monitoring and tracking the flow and behavior of data pipelines in real-time.
- It provides insights into data lineage, transformations, and usage patterns.
Synergy:
- When data observability is integrated with a data catalog, it provides users with a more complete understanding of how data moves through the organization.
- Users can trace the journey of data from its source to its destination, making data discovery and accessibility more transparent and efficient.
2. Data quality and reliability #
Data cataloging:
- A well-maintained data catalog includes information about data quality, lineage, and transformations.
- However, data may change over time, and the catalog might not always reflect the most up-to-date information.
Data observability:
- By observing data pipelines and processes in real-time, data observability can provide insights into data quality issues, anomalies, and discrepancies as they occur.
- It helps identify and rectify data quality problems promptly.
Synergy:
- When integrated, data observability enhances the accuracy and reliability of the data catalog.
- Users can trust that the information in the catalog is aligned with the current state of data, leading to more confident decision-making.
3. Data governance and compliance #
Data cataloging:
- A data catalog plays a role in data governance by documenting data ownership, access controls, and compliance requirements.
Data observability:
- Observing data pipelines allows organizations to monitor data usage, access patterns, and potential security breaches in real time.
- This would help to ensure data governance and compliance.
Synergy:
- Integrating data observability into data cataloging enhances data governance practices.
- Organizations can track and audit data usage, ensuring that data is being used according to compliance regulations and policies.
4. Continuous improvement #
Data cataloging:
- A data catalog supports the documentation of data assets.
- It might not reflect changes to data sources, transformations, and usage patterns that happen after catalog entries are created.
Data observability:
- Real-time monitoring provided by data observability allows organizations to capture changes and anomalies as they occur.
- This enables faster response and continuous improvement.
Synergy:
- When combined, data observability and data cataloging create a dynamic environment where the catalog is continuously updated with the most recent data insights and usage patterns.
- This ensures that the catalog remains relevant and useful over time.
Data observability provides real-time insights into data behavior and quality, while data cataloging offers a structured view of data assets and their metadata.
By integrating these approaches, organizations can achieve a more holistic and effective data management strategy, ensuring that data is not only accessible and discoverable but also reliable, compliant, and adaptable to changing business needs.
Data observability and data catalog: Which is more critical? #
Choosing between a data catalog and data observability depends on your organization’s specific goals, priorities, and existing data infrastructure.
These two concepts serve different purposes but can complement each other when integrated effectively. Here are some considerations to help you decide which option is best for your organization:
When to choose a data catalog? #
- Data discovery and accessibility
- If your main concern is making data easily discoverable, understandable, and accessible to various teams and stakeholders, a data catalog is an excellent choice.
- It helps users find the right data assets efficiently.
- Metadata management
- If you need to manage metadata associated with your data assets, such as data definitions, source information, lineage, and ownership.
- A data catalog is designed specifically for this purpose.
- Collaboration and sharing
- If you want to promote collaboration among teams by allowing users to comment, rate, and share insights about specific datasets, a data catalog provides these features.
- Data governance
- If ensuring compliance with data governance policies, managing access controls, and maintaining data quality standards are critical.
- A data catalog can help enforce these practices.
- Decision-making
- If you want to enable data-driven decision-making by providing a clear view of available data assets and their context, a data catalog facilitates informed choices.
When to choose data observability? #
- Real-time monitoring:
- If your primary concern is ensuring the reliability, performance, and health of your data pipelines.
- It processes in real-time, data observability is a strong choice.
- Anomaly detection:
- If detecting anomalies, errors, and discrepancies in data flows as they happen is crucial for your data operations, data observability tools provide the necessary insights.
- Performance optimization:
- If optimizing data pipelines for better efficiency, identifying bottlenecks.
- It is addressing performance issues are your priorities, data observability can help you achieve these goals.
- Data lineage tracking:
- If you need to understand how data moves through your organization’s processes and transformations in real time, data observability solutions can provide dynamic data lineage.
- Compliance and security:
- If monitoring data access patterns, ensuring compliance with regulations, and maintaining data security are top concerns, data observability tools offer features for these purposes.
Integration for maximum benefit #
In many cases, choosing one over the other might not be necessary. Integrating data observability with a data catalog can yield significant benefits by combining the strengths of both approaches.
This integration allows you to enhance data discovery with real-time insights into data behavior and quality. It also ensures that your data catalog remains up-to-date and aligned with the current state of your data ecosystem.
Ultimately, the decision between a data catalog and data observability depends on your organization’s priorities and challenges. In many cases, a comprehensive data strategy will involve elements of both to create a holistic approach to managing, discovering, and ensuring the reliability of your data assets.
Data observability and data catalog: Explained with example #
The integration of data catalog and data observability creates a dynamic synergy that enhances data management, accessibility, and reliability. Let’s explore this integration with an example scenario:
Example scenario: E-commerce data pipeline #
Imagine an e-commerce company with a complex data pipeline that handles customer orders, inventory management, and sales analytics. The organization wants to ensure that data flows smoothly, anomalies are detected in real-time, and users can easily discover relevant datasets for analysis.
Data catalog contribution #
The data team starts by setting up a data Catalog to document various datasets, their sources, transformations, and attributes. For instance:
- Dataset: “Order Data”
- Source: Online Store Database
- Attributes: Order ID, Customer ID, Product ID, Quantity, Price
In this example, the data catalog provides a structured view of the “Order Data” dataset, offering information about its source, attributes, and its relevance within the organization’s operations.
Data observability contribution #
Data observability involves real-time monitoring and insights into the behavior of the data pipeline. For example:
- Data flow monitoring: Data observability tools continuously track the flow of data through various stages of the pipeline, from the online store’s database to inventory management and sales analytics.
- Anomaly detection: Let’s say that on a particular day, the quantity of products ordered is abnormally high, triggering an anomaly alert from the data observability system.
Summarizing it all together #
Data observability’s real-time insights into data behavior, lineage, and quality combine seamlessly with the structured repository of metadata that a Data Catalog offers.
This alliance enhances data discovery, ensuring that the right data is accessible at the right time. The tandem empowers collaboration, enabling teams to make more informed decisions based on accurate, up-to-date information.
As we conclude this exploration, it’s evident that the partnership of data catalog and data observability is not merely a trend but a forward-looking strategy. By embracing this alliance, organizations can usher in an era of data management excellence, where the sum is truly greater than its parts.
Data observability and data catalog: Related reads #
- AI Data Catalog: Exploring the Possibilities That Artificial Intelligence Brings to Your Metadata Applications & Data Interactions
- How AI Data Governance Shows Potential To Help You Scale Data Security, Integrity, Privacy, and Compliance
- 8 Ways AI-Powered Data Catalogs Save Time Spent on Documentation, Tagging, Querying & More
- Data Catalog: Does Your Business Really Need One?
- Open Source Data Catalog Software: 5 Popular Tools to Consider in 2023
- Enterprise data catalog: Definition, Importance & benefits
- Google Cloud Data Catalog Guide - Everything You Need to Know
- AWS Glue Data Catalog: Architecture, Components, and Crawlers
- Data Catalog Platform: The Key To Future-Proofing Your Data Stack
- Data Observability: Definition, Key Elements, and Business Benefits
- Data Lineage & Data Observability: Why Are They Important?
- Data Observability & Data Mesh: How Are They Related?
- Data Observability for Data Engineers: What, Why & How?
- 12 Popular Observability Tools: An overview
- Open Source Data Observability Tools: 7 Popular Picks
Share this article