Data Quality Culture: Everything You Need to Know in 2024!
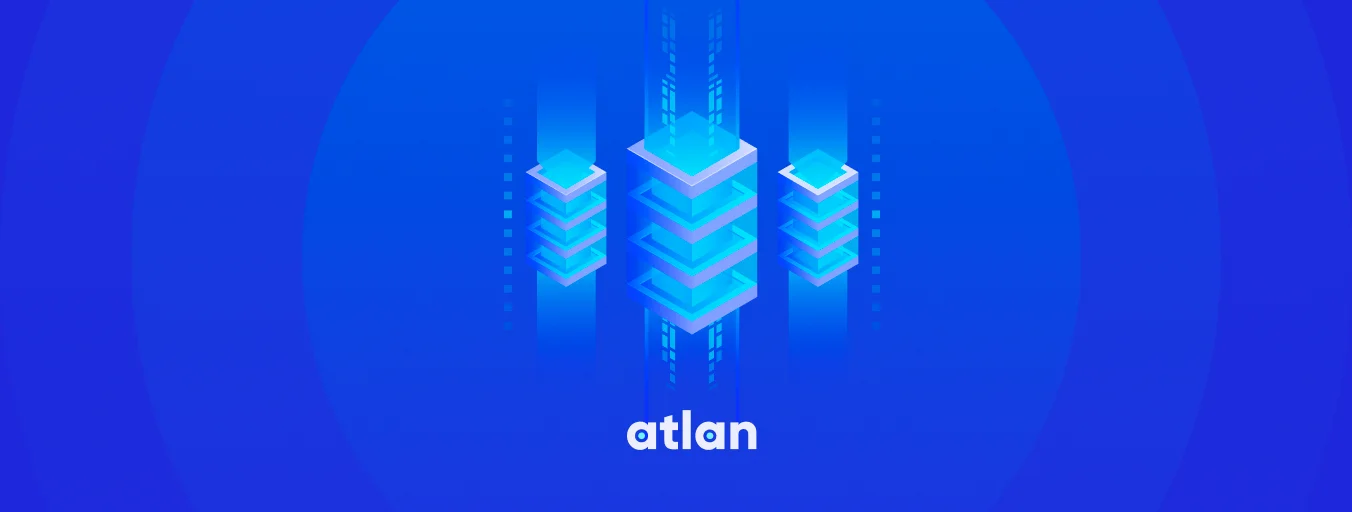
Share this article
A data quality culture refers to the collective attitudes, beliefs, and practices that prioritize the importance of maintaining high-quality data across an organization.
Investing in data quality tools is not enough, to reap the multifaceted benefits of reliable data, companies must nurture a culture that places quality at the core.
By understanding the hallmarks of a robust data quality culture, organizations can benchmark their progress and pinpoint areas for improvement. The insights can act as a roadmap for instilling an enterprise-wide recognition that reliable data is a strategic necessity, not just an it concern. Let us examine the cultural traits that separate data-driven leaders from the rest.
Modern data problems require modern solutions - Try Atlan, the data catalog of choice for forward-looking data teams! 👉 Book your demo today
In this article, we will explore:
- What is a data quality culture?
- Benefits of having a good data quality culture
- Steps to implement a data quality culture in different working environments
Let’s dive in!
Table of contents #
- What is a data quality culture?
- 5 Steps to implement a good data quality culture in your organization
- What are the signs of a good data quality culture in an organization?
- 6 Key benefits of a good data quality culture
- How does data quality culture align with other organizational cultures?
- 4 Simple steps to implement a data quality culture in a remote working environment
- Summary
- Related reads
What is a data quality culture? #
A data quality culture refers to an organizational environment where the accuracy, consistency, and reliability of data are prioritized and integrated into everyday practices.
A good data quality culture involves ensure high-quality data and fosters values, beliefs, and behaviors among all members of the organization that emphasize the importance of maintaining data quality.
The practices for good data quality culture include:
- Collective attitude
- Process standardization
- Data ownership
- Ongoing training
- Measurement and accountability
Let us understand these points in detail.
1. Collective attitude #
- Collective attitude refers to the shared mindset within the organization regarding the significance of data quality. This isn’t merely an “it issue”; it involves every department from marketing to finance.
- When everyone values data accuracy and reliability, data-driven decision-making becomes more effective. This collective attitude manifests in daily practices, where each member ensures that they contribute to maintaining data quality.
- The stronger this shared attitude, the more resilient the organization is against poor data practices.
2. Process standardization #
- Process standardization means having a set of defined procedures for how data is collected, processed, and managed. This ensures uniformity, reducing the risk of errors and inconsistencies.
- For example, a standardized data entry form can be used across different departments to maintain consistency.
- Standardized processes make it easier to audit data, ensuring that it meets quality requirements. This also speeds up data integration tasks and simplifies the work for analytics teams.
3. Data ownership #
- Data ownership assigns specific roles or teams the responsibility for particular sets of data within an organization. These data owners are accountable for the quality, privacy, and ethical use of the data they oversee.
- They are the go-to persons for any data-related queries and are responsible for data cleansing, updates, and quality assurance.
- By having a designated owner, the accountability for maintaining high-quality data is clear.
- Data ownership is often formally documented to prevent ambiguities and to align with compliance requirements.
4. Ongoing training #
- Ongoing training involves continuous education for staff on data quality principles, tools, and best practices. This is crucial because data ecosystems are dynamic and constantly evolving.
- Periodic workshops and training sessions help staff stay updated on the latest trends and technologies.
- Such training also reiterates the importance of data quality, keeping it at the forefront of employees’ minds. A well-trained team is less likely to make errors that compromise data quality, thereby maintaining the organization’s data integrity.
5. Measurement and accountability #
- Measurement and accountability ensure that data quality is not just a slogan but a quantifiable objective.
- Key performance indicators (KPIs) for data quality must be established and regularly monitored.
- Employees and teams are held accountable for these KPIs, encouraging a sense of responsibility.
- Regular audits, perhaps quarterly or bi-annually, help in checking whether the data meets the set quality standards. This fosters a culture where data quality is continually assessed and optimized, rather than being a one-off initiative.
Understanding data quality culture is the first crucial step in implementing a sustainable data governance strategy. It lays the foundation for how data is treated and valued within the organization, directly impacting the business’ overall success.
5 Steps to implement a good data quality culture in your organization #
Successfully incorporating good data quality culture within an organization is not just about implementing software solutions. It’s a multifaceted approach that involves strategy, human resources, technology, and ongoing monitoring.
The steps to a good data quality culture are as follows:
- Strategic planning
- Staff training and onboarding
- Implementing a data governance framework
- Choosing the right tools
- Monitoring and evaluating progress
Let us understand these points in detail:
1. Strategic planning #
Strategic planning is the first and most critical step. Organizations should start by defining their data quality objectives aligned with business goals.
A roadmap should be created that outlines how to achieve these objectives, which should be endorsed by leadership for maximum impact.
2. Staff training #
In staff training and onboarding, it’s crucial to ensure that everyone understands their role in maintaining data quality.
This could involve formal courses, workshops, and even periodic assessments. Employees must be trained not just on how to use data quality tools but also on why data quality is important for the organization.
3. Implementing data governance framework #
A comprehensive data governance framework needs to be in place, clearly outlining roles, responsibilities, and procedures around data.
This includes data ownership, data collection methodologies, and protocols for data security and compliance. An effective framework should be adaptable to the changing needs of the organization and its data landscape.
4. Choosing the right tools #
Choosing the right tools is pivotal for maintaining data quality. These should align with your data types, volumes, and the specific quality issues your organization faces.
The toolset could range from simple data cleaning tools to more complex data management and analytics platforms.
5. Monitoring and evaluating progress #
Finally, monitoring and evaluating progress is a continual process. Data quality should be measured against the set key performance indicators (KPIs), and regular audits should be conducted.
It’s essential to establish feedback mechanisms where employees can report issues or offer suggestions, as well as a method for implementing those changes.
Incorporating good data quality is an ongoing commitment that permeates all levels of an organization. From strategic planning to staff training, from choosing the right tools to regular monitoring, each aspect plays a crucial role.
A structured approach, backed by leadership and engaged employees, ensures that the organization moves towards a culture of data quality excellence.
What are the signs of a good data quality culture in an organization? #
The signs of a good data quality culture are multidimensional, encompassing leadership, collaboration, communication, tools, and most importantly, the engagement of each individual within the organization.
Identifying these signs helps organizations focus on areas of improvement, contributing to an enduring culture of data quality excellence.
These signs include:
- Leadership commitment
- Cross-functional collaboration
- Transparent communication
- Data quality tools and infrastructure
- Regular audits and feedback loops
- Employee engagement and accountability
Let us understand these points in detail:
1. Leadership commitment #
Leadership commitment is visible when top executives prioritize data quality in strategy and decision-making. Their support is often materialized through budget allocations for data governance initiatives.
This sets the organizational tone, making data quality a company-wide priority.
2. Cross-functional collaboration #
Cross-functional collaboration means teams from various departments work together on data quality.
This eliminates data silos, ensuring that every department follows consistent standards and practices. A unified approach to data quality is the result.
3. Transparent communication #
Transparent communication allows for an open dialogue about data quality issues within the organization. Employees feel empowered to flag discrepancies and suggest improvements.
This openness enables the early identification of problems, preventing them from escalating.
4. Data quality tools and infrastructure #
The presence of specialized data quality tools and infrastructure signals an organization’s seriousness about data quality. This could include data cleansing tools or analytics platforms.
Regular updates and maintenance of these tools signify an ongoing commitment to data quality.
5. Regular audits and feedback loops #
In organizations with good data quality culture, regular audits are a norm. Both automated and human-driven checks ensure compliance with quality standards.
Feedback loops are then utilized to make continuous improvements based on these audit results.
6. Employee engagement and accountability #
Employees who are engaged in and accountable for data quality exemplify a strong data quality culture. They take ownership of the data they handle and are held responsible for maintaining its integrity.
This often manifests through reward systems or penalties that incentivize data quality.
Recognizing the signs of a good data quality culture can help organizations benchmark their progress and set future goals. It also provides a framework for other organizations to emulate.
6 Key benefits of a good data quality culture #
A good data quality culture doesn’t just serve to keep your records neat and compliant. It serves as a cornerstone for achieving various strategic advantages that can set an organization apart from its competitor. Here are six key benefits:
- Improved decision-making
- Enhanced customer experience
- Regulatory compliance
- Operational efficiency
- Competitive advantage
- Employee satisfaction and productivity
Let us understand these benefits in detail.
1. Improved decision making #
Improved decision-making is one of the most immediate benefits. When decision-makers have access to high-quality, reliable data, they are more likely to make choices that align well with organizational objectives. This can lead to increased profitability, better resource allocation, and more effective strategies.
2. Enhanced customer experience #
Enhanced customer experience is another key advantage. High-quality data allows companies to understand customer needs, preferences, and behaviors more precisely. This understanding enables personalized marketing, better customer service, and ultimately, stronger customer relationships.
3. Regulatory compliance #
Regulatory compliance becomes significantly more straightforward in a culture that prioritizes data quality. Accurate and reliable data helps meet various industry regulations and standards, reducing the risk of legal consequences and the associated financial penalties.
4. Operational efficiency #
In terms of operational efficiency, organizations with good data quality cultures often see a reduction in errors and the amount of time spent correcting them. This results in cost savings and allows staff to focus on more value-added tasks.
5. Competitive advantage #
Competitive advantage can be garnered as high-quality data enables organizations to quickly adapt to market changes, predict consumer behavior, and make swift, informed decisions. This agility helps them stay ahead of competitors who might be relying on less accurate or timely information
6. Employee satisfaction and productivity. #
Lastly, employee satisfaction and productivity can also be improved. When employees work with high-quality data, their tasks become less burdensome and more meaningful. This leads to higher job satisfaction, which in turn can improve productivity and reduce turnover.
How does data quality culture align with other key organizational cultures? #
Data quality culture isn’t an isolated aspect of an organization’s operations. It often intersects and aligns with other key organizational cultures, such as innovation, ethics, and sustainability. Understanding this alignment can help create a holistic and integrated approach to organizational success.
- Alignment with innovation culture
- Alignment with ethical culture
- Alignment with sustainability culture
Let us understand them in detail:
1. Alignment with innovation culture #
Alignment with innovation culture involves the use of high-quality data as the bedrock for research and development initiatives. Accurate and reliable data empowers teams to test new ideas, validate hypotheses, and confidently move towards innovative solutions.
In turn, a culture of innovation encourages the continuous improvement of data processes, creating a beneficial cycle.
2. Alignment with ethical culture #
Alignment with ethical culture is perhaps one of the most critical intersections. Good data quality includes ensuring data privacy and ethical sourcing and handling of data.
An organization committed to ethical behavior will find this naturally aligns with the principles of a strong data quality culture.
Ethical considerations also foster trust, both internally among employees and externally with stakeholders, enhancing the quality and reliability of data.
3. Alignment with sustainability culture #
Alignment with sustainability culture is increasingly important as organizations aim to be responsible corporate citizens.
High-quality data can provide the insights necessary for sustainable operations, such as efficient resource use or waste reduction. Conversely, a culture of sustainability encourages transparency and quality in data reporting, which are key elements in data quality culture.
When understood and executed well, a strong data quality culture can act as a foundational pillar that supports other cultural initiatives within an organization. It is not just compatible but also synergistic with cultures of innovation, ethics, and sustainability.
Aligning these cultures not only amplifies the benefits of each but also contributes to a holistic, well-rounded organizational ethos.
4 Simple steps to implement a data quality culture in a remote working environment #
In today’s increasingly remote and distributed work environments, integrating a data quality culture poses unique challenges. However, it also offers opportunities for organizations to harness the power of data across geographical and functional boundaries.
Four key pointers to remember
- Remote onboarding and training
- Data governance in the cloud
- Communication and collaboration tools
- Regular virtual audits
Let us understand them in detail.
1. Remote onboarding and training #
Remote onboarding and training are crucial first steps in embedding a data quality culture in remote teams. New hires should go through a thorough orientation that includes training modules focused on data governance policies and best practices.
Refresher courses and periodic evaluations should be made accessible online for all team members, irrespective of their location.
2. Data governance in the cloud #
Data governance in the cloud is essential for remote teams, given that most will rely on cloud-based storage and applications. Make sure your cloud solutions have robust data governance features, such as access control, encryption, and backup.
In addition, there should be clear protocols for who can access what data and how they should handle it, to ensure that quality is maintained regardless of geography.
3. Communication and collaboration tools #
Communication and collaboration tools play a significant role in maintaining data quality culture within remote or distributed teams. Tools that provide real-time data sharing, version control, and robust security features can help in maintaining data integrity.
Teams should also be trained to communicate effectively about data quality issues using these platforms.
4. Regular virtual audits #
Regular virtual audits can be particularly helpful in ensuring that remote teams are complying with data quality standards.
Scheduled virtual check-ins, audits, or inspections can help identify any disparities in data handling and rectify them before they escalate.
Integrating a data quality culture into remote or distributed teams requires a well-thought-out blend of technology, training, and continuous monitoring. By leveraging modern tools and maintaining clear channels of communication, organizations can instill a robust data quality culture across geographies, ensuring that the quality of data remains uncompromised.
Summing up #
The benefits of a good data quality culture are multi-faceted, impacting almost every area of business operations. From making informed decisions to keeping customers happy, from ensuring compliance to boosting employee morale, the advantages are both immediate and long-term.
Investing in data quality culture is not just a technical necessity but a strategic imperative for sustained organizational success.
Data quality culture: Related reads #
- Data Quality Measures: A Step-by-Step Implementation Guide
- How to Improve Data Quality: Strategies and Techniques to Make Your Organization’s Data Pipeline Effective
- How to Ensure Data Quality in Healthcare Data: Best Practices and Key Considerations
- Data Quality in Data Governance
- 6 Popular Open Source Data Quality Tools in 2024: Overview, Features & Resources
- Automated quality control of data pipelines
- What Is a Data Catalog? & Why Do You Need One in 2024?
- Data Governance 101: Principles, Examples, Strategy & Programs
- Data Governance Committee 101: When Do You Need One?
Share this article