10 Steps to Improve Data Quality in Healthcare
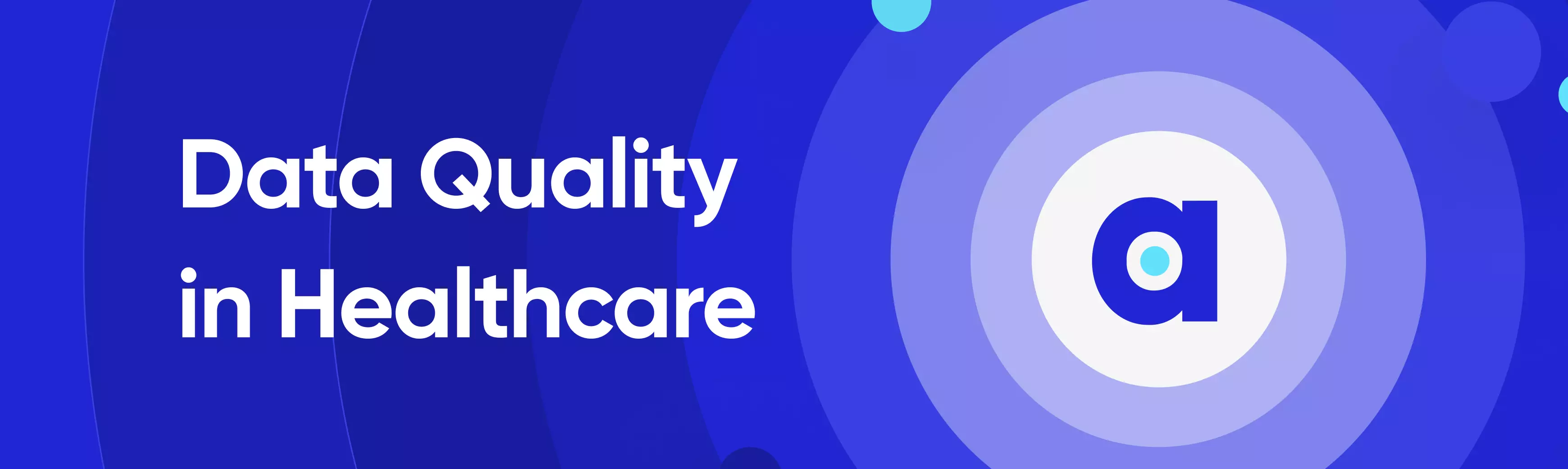
Share this article
Data quality is of paramount importance in the healthcare sector for several reasons. High-quality data enables healthcare organizations to make informed decisions, improve patient outcomes, streamline operations, and maintain regulatory compliance.
Modern data problems require modern solutions - Try Atlan, the data catalog of choice for forward-looking data teams! 👉 Book your demo today
In this article, we will explore the importance of data quality in healthcare, and the best practices to improve it.
Let’s dive in!
Table of contents
- What is the role of data quality in healthcare: 6 Key aspects to consider
- The importance of data quality in healthcare
- How to ensure data quality in healthcare: 10 Steps to follow
- 8 Key considerations for effective data quality management in healthcare
- Rounding it all up
- Data quality in healthcare: Related reads
What is the role of data quality in healthcare data: 6 Key aspects to consider
Here is why data quality is essential in healthcare:
- Patient safety and care
- Interoperability
- Regulatory compliance
- Operational efficiency
- Research and innovation
- Decision-making and strategic planning
Let’s look into each of these aspects in detail:
1. Patient safety and care
Accurate, complete, and consistent data is crucial for proper diagnosis and treatment of patients. High-quality data ensures that healthcare professionals have the right information at the right time to make informed decisions about patient care, reducing the risk of errors and improving overall patient outcomes.
2. Interoperability
Healthcare organizations need to exchange data with various entities such as other hospitals, clinics, insurance providers, and government agencies. Ensuring data quality is vital to maintain seamless interoperability and effective communication among these entities, ultimately improving care coordination and patient experiences.
3. Regulatory compliance
Healthcare organizations must adhere to strict regulations regarding patient privacy and data security, such as the Health Insurance Portability and Accountability Act (HIPAA) in the United States. High-quality data is necessary to maintain compliance with these regulations, as inaccurate or inconsistent data could lead to breaches or penalties.
4. Operational efficiency
Accurate and reliable data is crucial for streamlining operations and reducing costs. High-quality data allows healthcare organizations to optimize resource allocation, better manage their supply chain, and identify opportunities for process improvements.
5. Research and innovation
Healthcare research relies on large volumes of high-quality data to develop new treatments, improve existing ones, and identify patterns in disease progression. Ensuring data quality is fundamental for driving innovation and advancing the overall state of healthcare.
6. Decision-making and strategic planning
High-quality data enables healthcare organizations to make informed decisions and develop strategies for growth, resource allocation, and risk management. This includes areas such as patient population management, cost containment, and quality improvement initiatives.
In summary, ensuring data quality is critical to the success of healthcare organizations in providing safe, effective, and efficient care. It also plays a significant role in regulatory compliance, research, innovation, and overall decision-making within the organization. Investing in data quality management will help your organization to leverage data effectively, reduce risks, and improve patient outcomes.
The importance of data quality in healthcare: Hypothetical examples of why it matters
Let’s look into each of these hypothetical examples for the points mentioned above:
1. Patient safety and care
A patient is admitted to the hospital with severe chest pain. Incomplete or inaccurate medical records could lead to an incorrect diagnosis, potentially causing the healthcare provider to administer inappropriate treatment or miss a critical issue, such as an underlying heart condition.
High-quality data ensures that healthcare professionals can make informed decisions, leading to accurate diagnoses and effective treatments.
2. Interoperability
Hospital A needs to transfer a patient to Hospital B for a specialized surgery. Inaccurate or inconsistent patient data can lead to delays in care, as Hospital B may need to request additional information or perform redundant tests. High-quality data enables seamless communication and coordination between healthcare facilities, reducing delays and improving the patient experience.
3. Regulatory compliance
A healthcare organization experiences a data breach, and an investigation reveals that the cause was due to inconsistent data security practices across different departments. Ensuring high-quality data and adhering to regulatory requirements can help organizations avoid such incidents and the associated penalties.
4. Operational efficiency
A hospital frequently experiences inventory shortages of critical medical supplies, resulting in delays or suboptimal care. Accurate and up-to-date data on inventory levels, usage patterns, and supplier lead times can help the hospital optimize its supply chain management, reducing costs and ensuring the timely availability of necessary resources.
5. Research and innovation
Researchers are analyzing electronic health records to study the effectiveness of a new cancer treatment. Inaccurate or incomplete data could lead to erroneous conclusions or missed opportunities for identifying patterns and refining the treatment. High-quality data ensures that research findings are more reliable, driving innovation and improving patient outcomes.
6. Decision-making and strategic planning
A healthcare organization wants to invest in a new service line to better serve its community. High-quality data on patient demographics, health trends, and market competition can help the organization make informed decisions about which service lines to prioritize and how to allocate resources effectively.
These hypothetical examples illustrate the importance of data quality in various aspects of healthcare, emphasizing the need for healthcare organizations to invest in data quality management to achieve better patient outcomes, operational efficiency, and compliance.
How to ensure data quality in healthcare? 10 Steps to follow
Improving data quality in the healthcare industry involves a systematic approach that encompasses people, processes, and technology. Here are some steps to help you improve your organization’s data quality:
- Establish a data governance framework
- Develop data quality policies and standards
- Assess current data quality
- Implement data quality improvement initiatives
- Invest in data quality tools and technologies
- Enhance data capture and entry processes
- Monitor and measure data quality
- Foster a culture of data quality
- Continuously improve data quality processes
- Collaborate with external partners
Let’s look into each of the steps to manage data quality in healthcare in brief:
1. Establish a data governance framework
Create a data governance team that includes stakeholders from various departments, such as IT, clinical, and administrative staff. Define roles, responsibilities, and accountabilities for data quality management, ensuring clear communication and collaboration among team members. Developing a data governance framework and identifying who is responsible for data quality will help you effectively manage data quality.
2. Develop data quality policies and standards
Define data quality objectives, policies, and standards specific to your organization’s needs and the healthcare industry’s regulatory requirements. Ensure that these policies and standards are well-documented, communicated, and easily accessible for all team members.
3. Assess current data quality
Conduct an initial data quality assessment to identify gaps and areas for improvement. Use data profiling and data quality tools to evaluate the completeness, accuracy, consistency, timeliness, and uniqueness of your data.
4. Implement data quality improvement initiatives
Based on the assessment results, prioritize and implement data quality improvement initiatives. These may include data cleansing, data enrichment, data validation, and standardization, among other activities.
5. Invest in data quality tools and technologies
Utilize data quality tools and technologies to automate data validation, cleansing, and monitoring processes. Implement data integration platforms to facilitate seamless data exchange and ensure data consistency across systems.
6. Enhance data capture and entry processes
Streamline and standardize data capture and entry processes to minimize errors and inconsistencies. Provide training and guidelines to staff involved in data entry to ensure that they understand the importance of data quality and adhere to established standards.
7. Monitor and measure data quality
Establish key performance indicators (KPIs) to track data quality over time. Regularly monitor and measure data quality against these KPIs, and adjust your improvement initiatives as needed.
8. Foster a culture of data quality
Encourage a culture of data quality awareness and accountability across the organization. Conduct regular training sessions, workshops, and communication campaigns to emphasize the importance of data quality and its impact on patient care, operational efficiency, and compliance.
9. Continuously improve data quality processes
Regularly review and update your data quality policies, standards, and processes to keep pace with the evolving healthcare landscape and your organization’s needs. Use lessons learned from previous data quality initiatives to refine and enhance your data quality management approach.
10. Collaborate with external partners
Work closely with external partners, such as other healthcare providers, payers, and technology vendors, to ensure consistent data quality across the healthcare ecosystem. Share best practices and collaborate on data quality improvement efforts.
By following these steps, your healthcare organization can effectively improve its data quality, enabling better decision-making, improved patient outcomes, and enhanced operational efficiency.
8 Key considerations for effective data quality management in healthcare
In addition to the steps and examples mentioned earlier, there are several other factors to consider regarding data quality in healthcare, such as:
- Privacy and security
- Data lineage and provenance
- Integration of multiple data sources
- Data quality in real-time
- Patient-generated data
- Data quality in AI and machine learning
- Continuous data quality improvement
- Change management
Let us look into each of the above factors in detail:
1. Privacy and security
Ensuring data quality should go hand-in-hand with protecting patient privacy and maintaining data security. Implement strong data encryption, access controls, and monitoring systems to safeguard sensitive healthcare data from unauthorized access, breaches, and misuse.
2. Data lineage and provenance
Understanding the source, history, and transformations of your data is essential for establishing trust in the data. Maintain clear documentation of data lineage and provenance to ensure transparency and traceability in your data quality efforts.
3. Integration of multiple data sources
Healthcare organizations often need to integrate data from various sources, such as EHR systems, lab systems, imaging systems, and billing systems. Ensuring data quality across these sources can be challenging, so invest in data integration platforms that facilitate seamless data exchange and maintain consistency across systems.
4. Data quality in real-time
Healthcare organizations are increasingly leveraging real-time data for decision-making, patient monitoring, and early intervention. Ensure that your data quality efforts extend to real-time data streams, as well as batch and historical data.
5. Patient-generated data
With the increasing adoption of remote patient monitoring and wearable devices, patient-generated data is becoming more crucial for healthcare organizations. Develop processes to validate, clean, and standardize this data to ensure its quality and usability.
6. Data quality in AI and machine learning
Healthcare organizations are increasingly using AI and machine learning for various applications, such as predictive analytics, diagnosis support, and personalized care. Ensure that your data quality efforts support these advanced analytics initiatives, as poor data quality can lead to inaccurate predictions, biased results, and suboptimal care.
7. Continuous data quality improvement
Data quality management should be an ongoing process, with regular assessments, monitoring, and improvement initiatives. Develop a feedback loop to learn from past data quality issues and refine your processes to prevent future occurrences.
8. Change management
Implementing data quality initiatives in healthcare organizations can involve significant cultural and operational changes. Develop a comprehensive change management plan to engage stakeholders, address resistance, and facilitate the successful adoption of data quality practices.
By considering these additional factors, your healthcare organization can further strengthen its data quality efforts, leading to better patient care, improved operational efficiency, and more informed decision-making.
Rounding it all up
In summary, data quality is crucial for the healthcare sector as it enables informed decision-making, improved patient outcomes, and regulatory compliance. By investing in data quality management and considering the various factors discussed, healthcare organizations can better leverage their data, leading to improved patient care, operational efficiency, and informed decision-making.
Data quality in healthcare: Related reads
- Achieving HIPAA Compliance with Data Governance
- How to Improve Data Quality: Strategies and Techniques to Make Your Organization’s Data Pipeline Effective
- Automated quality control of data pipelines
- What Is a Data Catalog? & Why Do You Need One in 2023?
- Is Atlan compatible with data quality tools?
- Data Governance 101: Principles, Examples, Strategy & Programs
Share this article