Data Quality Problems? 5 Ways to Fix Them in 2024!
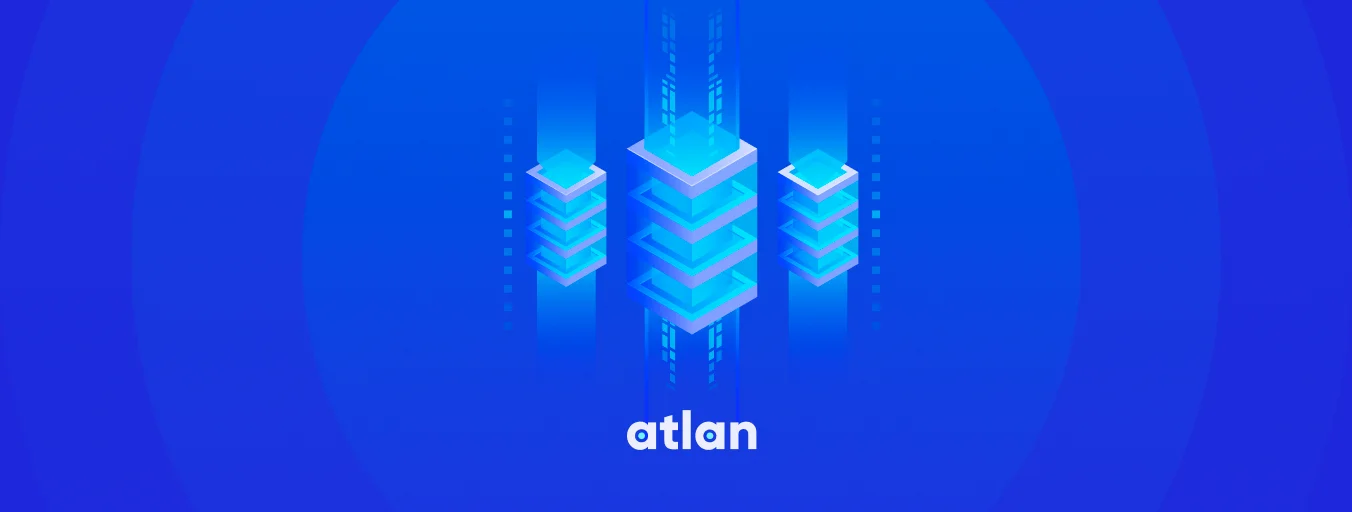
Share this article
Data quality problems are the issues and discrepancies within datasets that hinder their accuracy, completeness, consistency, and reliability. As a result, they contribute the cost of poor data.
And Gartner’s Data Quality Market Survey showed that the average annual financial cost of poor data is to the tune of $15M. So, data quality problems can disrupt operations, compromise decision-making, and erode customer trust.
Modern data problems require modern solutions - Try Atlan, the data catalog of choice for forward-looking data teams! 👉 Book your demo today
In this article, we will understand what are the common data quality problems, how to fix them, factors affecting the data quality.
Ready? Let’s dive in!
Table of contents
- What are data quality problems?
- 7 Common data quality problems
- How can you fix data quality problems?
- What are the factors affecting data quality?
- Summing up
- Related reads
What are data quality problems?
Data quality problems are the issues in the characteristics of data that negatively affect its ability to serve its intended purpose. These problems can significantly impact the accuracy, reliability, and usefulness of the data in various contexts, such as business decision-making, scientific research, and day-to-day operations.
Addressing data quality problems typically involves a multifaceted approach, including implementing data governance policies, regular data auditing, using sophisticated data cleaning tools, and fostering a culture of data awareness and responsibility within the organization.
These steps help ensure that the data is as accurate, complete, consistent, reliable, relevant, timely, usable, and has integrity as possible, thereby enhancing its value and effectiveness for its intended use. And, now let’s look at some typical data quality problems.
7 Common data quality problems
Understanding common data quality problems is paramount as it enables proactive measures to enhance data reliability, integrity, and security, fostering informed decision-making and preventing potential risks associated with poor-quality data.
And here are the seven common data quality problems:
- Incomplete data
- Inaccurate data
- Duplicate data
- Inconsistent data
- Outdated data
- Data integrity issues
- Data security and privacy concerns
Let’s understand each problem in detail.
1. Incomplete data
Incomplete data refers to the presence of missing or incomplete information within a dataset. This can occur for various reasons, such as data entry errors, system limitations, or data sources not providing certain required details.
Incomplete data can lead to inaccuracies in analysis and decision-making, as it may result in gaps or biases in the dataset. Addressing this issue involves data validation processes, data collection improvements, and ensuring that all necessary information is consistently and accurately recorded.
2. Inaccurate data
Inaccurate data encompasses errors, discrepancies, or inconsistencies within a dataset. These inaccuracies can originate from various sources, including human errors during data entry, system malfunctions, or issues with data integration.
Inaccurate data can lead to faulty conclusions and misguided decisions. Resolving this issue often requires rigorous data validation and cleansing procedures, data quality monitoring, and implementing data entry validation rules to prevent errors at the source.
3. Duplicate data
Duplicate data arises when identical records or entries are present in a dataset. This can result from data entry errors, system glitches, or issues during data integration.
Duplicate data can lead to redundancy, increased storage costs, and misinterpretation of information if not properly identified and managed. De-duplication processes, data cleansing, and the implementation of unique identifiers can help address this issue.
4. Inconsistent data
Inconsistent data occurs when data elements within a dataset are not uniform or do not adhere to a consistent format or standard. This inconsistency can make data challenging to merge, analyze, or utilize cohesively. It often arises due to data entry variations, evolving data sources, or a lack of standardized data governance practices.
To mitigate this issue, organizations must establish clear data standards, enforce data quality guidelines, and use data transformation and cleansing techniques to ensure consistency.
5. Outdated data
Outdated data consists of information that is no longer current or relevant. This can occur over time as data ages and becomes obsolete. Outdated data can lead to misinformed decisions, as it does not accurately reflect the current state of affairs.
To address this issue, organizations should implement data update and refresh procedures, data aging policies, and regular data maintenance routines to ensure that data remains current and relevant.
6. Data integrity issues
Data integrity issues encompass a range of problems related to data accuracy, consistency, and reliability. These issues can include violations of data integrity constraints, data corruption, or unauthorized data modifications.
Data integrity problems can harm data quality and trustworthiness, and they often require strong data validation, constraints, and access controls to maintain the integrity of data.
7. Data security and privacy concerns
Data security and privacy concerns involve issues related to the protection of data against unauthorized access, breaches, or improper handling. These concerns can harm data quality and an organization’s reputation.
Addressing data security and privacy issues involves implementing robust security measures, access controls, encryption, and compliance with privacy regulations to safeguard data from unauthorized access and maintain data quality and trustworthiness.
How can you fix data quality problems? 5 Ways to get around data quality issues
Addressing data quality issues is essential to ensure the accuracy and reliability of data, which is critical for making informed decisions and maintaining trust in data-driven processes.
Here are five strategies to fix common data quality issues:
- Data validation and cleaning
- Standardization and consistency
- De-duplication
- Regular data audits and updates
- Data security and privacy measures
Let’s look at them in detail:
1. Data validation and cleaning
Data validation rules include checks for data accuracy and completeness during data entry to prevent errors at the source. Validation checks can include format validation (e.g., ensuring valid email addresses), range validation (e.g., verifying that a value falls within an expected range), and presence validation (e.g., ensuring required fields are filled).
Data cleansing procedures involve identifying and rectifying errors within the data, such as correcting misspelled names or eliminating inconsistent data formats.
2. Standardization and consistency
Clear data standards define how data should be structured, formatted, and labeled. Data quality guidelines ensure that data is maintained consistently according to these standards.
Data transformation and cleansing techniques are used to convert data into a common format or structure. For instance, converting dates into a uniform format like YYY-MM-DD.
3. De-duplication
De-duplication processes involve identifying and eliminating duplicate records within datasets. This can be done by comparing records and retaining only one instance of duplicated data.
Using unique identifiers (such as customer IDs) helps prevent the creation of new duplicates by ensuring that each data entry has a distinct identifier.
4. Regular data audits and updates
Regular data audits involve a systematic review of datasets to identify missing or outdated information. This can be done by comparing data against predefined criteria or business rules.
Data aging policies define when data becomes outdated and should be updated or archived. Regular data updates ensure that information remains current and relevant.
5. Data security and privacy measures
Data security measures, including encryption and access controls, protect data from unauthorized access or breaches. Encryption ensures that data is scrambled and can only be deciphered with the correct decryption key.
Compliance with privacy regulations (e.g., GDPR or HIPAA) ensures that sensitive data is handled according to legal and ethical standards, reducing the risk of privacy breaches and data security issues.
Implementing these strategies is essential for maintaining data integrity and ensuring that data remains a valuable asset for decision-making and analysis. Data quality is an ongoing effort, and organizations must regularly monitor, clean, and secure their data to optimize its value.
What are the factors affecting data quality? 10 factors to look out for
Data quality is crucial for accurate decision-making and meaningful analysis. Several factors can affect data quality, and understanding these factors is essential for maintaining and improving data integrity.
Here are the key factors that can impact data quality:
- Data governance practices
- Data validation and cleansing processes
- Data integration challenges
- Data migration and transfer errors
- Data storage and retrieval efficiency
- Data privacy and security concerns
- Data ownership and accountability
- Data handling and access controls
- Data source reliability
- Data standardization and consistency
Let’s look at them in detail:
1. Data governance practices
Effective data governance involves establishing policies and procedures to manage data throughout its lifecycle. This includes defining data ownership, classifying data, and protecting it according to organizational standards.
Data governance encompasses data stewardship, data quality rules, and the development of data dictionaries. It also involves setting access controls and establishing clear roles and responsibilities for data management to maintain data quality and protect against misuse.
2. Data validation and cleansing processes
Data validation focuses on checking data during data entry to prevent errors and ensure data accuracy and completeness. Data cleansing involves identifying and correcting inaccuracies in existing datasets, such as correcting misspelled names or eliminating inconsistent data formats.
3. Data integration challenges
Data integration challenges arise when combining data from various sources with differing formats and structures, requiring solutions for harmonizing and unifying the data effectively.
This often involves data mapping, transformation, and reconciliation to ensure data consistency and compatibility. Addressing integration challenges may involve the use of Extract, Transform, Load (ETL) processes and data integration platforms.
4. Data migration and transfer errors
Data migration and transfer errors can occur during the movement of data between systems, potentially leading to data loss or corruption if not managed properly.
These errors often result from mismatches in data formats, incomplete transfers, or issues during the data transfer process. Implementing data migration best practices and thorough testing is essential to mitigate these errors.
5. Data storage and retrieval efficiency
Data storage and retrieval efficiency relates to the speed and reliability of storing and retrieving data, significantly impacting an organization’s ability to access and utilize data promptly.
Efficient data storage solutions, including optimized databases and storage systems, are essential to maintain data quality and accessibility.
6. Data privacy and security concerns
Data privacy and security concerns involve safeguarding data against unauthorized access, and breaches, and ensuring compliance with privacy regulations to protect sensitive information.
This includes encrypting sensitive data, implementing access controls, and adhering to data protection laws to mitigate privacy and security risks.
7. Data ownership and accountability
Data ownership and accountability entail defining clear roles and responsibilities for data management. It ensures that individuals or teams take ownership of data quality and integrity.
This factor emphasizes the importance of having responsible stewards who oversee data-related activities and maintain data quality.
8. Data handling and access controls
Data handling and access controls involve the implementation of measures that regulate who can access and manipulate data.
Access controls define who has permission to view, edit, or delete data, preventing unauthorized access or inappropriate use. Proper data handling practices are crucial for maintaining data quality and security.
9. Data source reliability
Data source reliability concerns the trustworthiness and accuracy of the data sources utilized. Reliable data sources provide high-quality data, while unreliable sources can introduce inaccuracies and inconsistencies.
Organizations must carefully evaluate and verify the credibility of data sources to maintain data quality.
10. Data standardization and consistency
Data standardization and consistency are essential for ensuring data is uniformly structured, labeled, and formatted. This factor involves creating and adhering to data standards and guidelines and minimizing variations in data presentation.
Standardized and consistent data facilitates cohesive analysis and decision-making while reducing errors resulting from data discrepancies.
Summing up
In a nutshell, incomplete data, inaccurate data, duplicate data, inconsistent data, outdated data, data integrity issues, data security and privacy concerns are some of the typical data quality problems.
They are pervasive and can significantly hinder an organization’s operations and decision-making. Recognizing and addressing these issues is crucial for maintaining data integrity.
By implementing best practices for data validation, cleansing, governance, integration, and migration, organizations can secure their data’s reliability and ensure its value in data-driven environments.
Data Quality Problems: Related reads
- Data Quality Issues: Steps to Assess and Resolve Effectively
- How to Fix Your Data Quality Issues: 6 Proven Solutions!
- Data Quality Measures: Best Practices to Implement
- Data Quality Explained: Causes, Detection, and Fixes
- Is Atlan compatible with data quality tools?
- A Blueprint for Bulletproof Data Quality Management
- Data Quality Strategy: 10 Steps to Excellence!
- Data Quality Fundamentals: Why It Matters in 2024!
- Data Quality Testing: Key to Ensuring Accurate Insights!
- Data Quality is Everyone’s Problem, but Who is Responsible?
- Data Quality and Observability - Why Are They Important?
Share this article