Data Quality Issues: Steps to Assess and Resolve Effectively
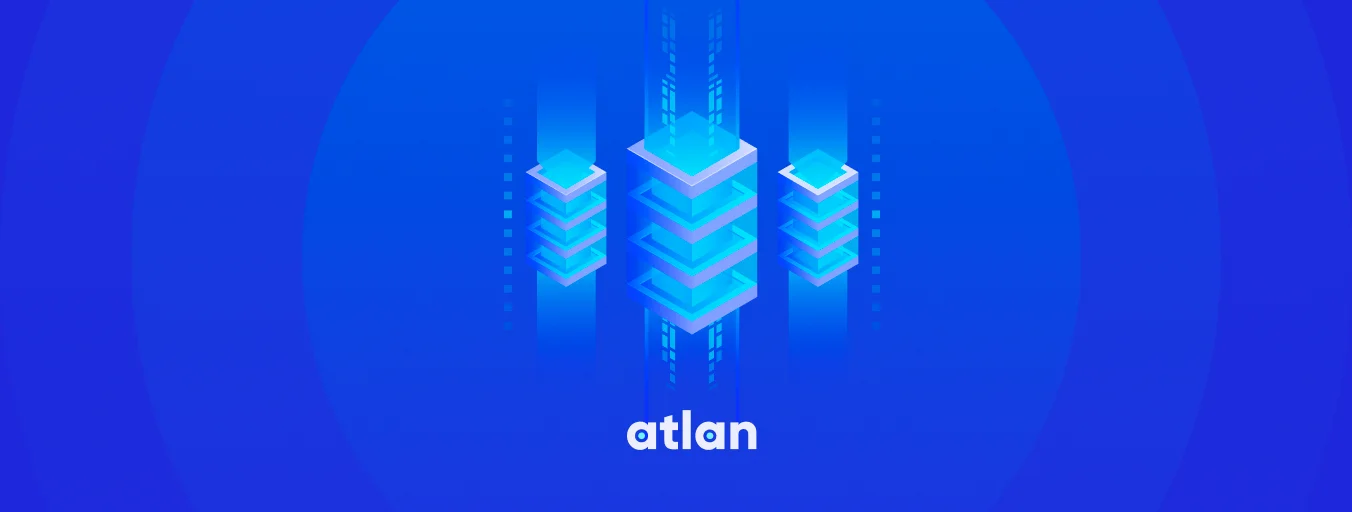
Share this article
Data has become the lifeblood of the modern digital economy, underpinning critical decisions and workflows. However, poor data quality can have severe consequences, especially for industries like banking, healthcare, and big data where flawed data can impact people’s finances, health, and privacy.
Data quality issues like inaccuracy, inconsistency, and lack of integrity of data can erode trust and impair organizations across sectors.
Modern data problems require modern solutions - Try Atlan, the data catalog of choice for forward-looking data teams! 👉 Book your demo today
This article explores the crucial relationship between data quality and key industries. We dive into the specific data challenges faced by banking, healthcare, and big data sectors.
Table of contents #
- Understanding data quality issues
- How does one assess data quality issues in 6 easy steps?
- Data quality issues in banking and their solutions
- Data quality issues in healthcare and their solutions
- What are the biggest quality issues in big data?
- Conclusion
- Related reads
Understanding data quality issues #
In today’s data-driven landscape, major data quality issues can cripple an organization’s effectiveness and decision-making processes.
Let’s delve into the key challenges that often plague various industries, including banking, healthcare, and big data.
Major data quality issues include:
- Inaccurate data entry
- Incomplete data
- Duplicate entries
- Outdated information
- Inconsistent formatting
- Lack of data governance
Let us understand them in detail:
1. Inaccurate data entry #
Inaccurate data entry is a pervasive issue stemming from human errors during manual data input. These errors can range from simple typos and incorrect values to using the wrong units of measurement.
For instance, in the banking sector, a mistyped interest rate can lead to inaccurate calculations, affecting loan and investment decisions. In healthcare, entering incorrect patient information can result in treatment errors and patient safety concerns.
2. Incomplete data #
Incomplete data occurs when essential information is missing from datasets. In the healthcare industry, incomplete patient records can hinder accurate medical assessments, diagnoses, and treatment plans.
Incomplete financial transaction records can lead to gaps in banking analysis, impeding fraud detection and risk assessment. Addressing this issue requires ensuring that all necessary fields are captured accurately, minimizing information gaps.
3. Duplicate entries #
Duplicate entries arise when the same data is recorded more than once, either due to system errors or human oversight. This issue can inflate data volume, consume storage resources, and create confusion during analysis. In the context of banking, duplicate records might lead to inaccurate customer account balances or erroneous transaction histories.
In healthcare, duplicate patient records can result in unnecessary procedures and treatments. Implementing validation rules and automated checks can help identify and prevent duplicate entries.
4. Outdated information #
Relying on outdated data can lead to misguided decisions. In the banking sector, using outdated market trends can result in suboptimal investment strategies. Similarly, in healthcare, outdated research or medical guidelines can influence treatment choices, affecting patient outcomes.
Organizations need robust mechanisms to track data freshness and ensure that decision-makers have access to the most current and relevant information.
5. Inconsistent formatting #
Inconsistent data formatting across different sources poses challenges for data integration and analysis. In the realm of big data, where information is drawn from diverse platforms, standardization becomes crucial.
Varying date formats, units, and naming conventions can hinder effective data processing. Creating data transformation pipelines and using tools that facilitate data harmonization is vital to address this issue.
6. Lack of data governance #
A lack of data governance encompasses unclear ownership, standards, and protocols for data management. In the banking sector, this can result in inconsistent customer information across different touchpoints, leading to a fragmented customer experience.
In the healthcare sector, inadequate data governance can hamper data sharing among different medical departments, hindering holistic patient care. Establishing data ownership, defining data quality standards, and implementing data stewardship practices are essential to ensure data integrity and consistency.
Addressing these data quality issues is pivotal for organizations aiming to harness data-driven insights effectively.
How does one assess data quality issues in 6 easy steps? #
Accurately evaluating data quality issues is essential for organizations to make informed decisions and improve their operations. Let’s explore how one can effectively assess data quality issues across various sectors, including banking, healthcare, and big data.
Some of the key steps to assess data quality include:
- Data profiling
- Data auditing
- Data validation and cleansing
- Comparing data from multiple sources
- Monitoring data quality metrics
- User feedback and domain expert involvement
1. Data profiling #
Data profiling transcends mere examination—it’s an intricate process that unveils the essence of data. By meticulously dissecting datasets, professionals gain profound insights into their composition, uncovering data types, value distributions, and hidden patterns.
This forensic approach reveals anomalies, disparities, and outliers lurking within the data’s fabric. For the banking sector, data profiling becomes a detective’s tool, uncovering irregularities in transaction timestamps that might unveil potential fraudulent activities.
In healthcare, it’s a guardian of integrity, exposing incongruities between patient records and medical codes, and ensuring accurate billing and regulatory adherence.
2. Data auditing #
Data auditing, an intellectual symphony, orchestrates harmonious scrutiny of data sources, pipelines, and transformations.
The auditor’s baton guides the exploration of every note and rhythm, ensuring that the composition adheres to precision and compliance.In healthcare, data audits are akin to medical examinations, meticulously cross-referencing patient records and treatment protocols.
The harmony of accurate billing and patient safety echoes through a thorough examination, leading to improved care quality and regulatory compliance.
3. Data validation and cleansing #
Data validation is the craftsman’s practice, chiseling the raw data into a masterpiece of accuracy. Imposing predefined standards, validators scrutinize each stroke of data, ensuring its alignment with established norms.
Subsequently, data cleansing commences—a meticulous restoration process, fixing inaccuracies, bridging gaps, and expelling duplicates.
Within the realm of big data, data validation and cleansing are the alchemists’ touch, transforming the cacophony of user-generated content into the gold of reliable sentiment analysis and predictive insights.
4. Comparing data from multiple sources #
Comparing data from disparate sources is the conductor’s baton, orchestrating the symphony of consistency. Each data source is an instrument, and the conductor harmonizes their melodies to unearth discrepancies and deviations.
In banking, this harmonious pursuit illuminates inconsistent customer information across databases, highlighting variations in contact details or account balances.
The result—a more accurate, unified customer profile that resonates through every banking interaction.
5. Monitoring data quality metrics #
Monitoring data quality metrics is akin to measuring a patient’s vital signs—a constant check on the data’s pulse, ensuring its vitality over time.
For healthcare, tracking patient data completeness and accuracy metrics mirrors a physician’s diligent observation. The health of medical research and the precision of clinical decisions depend on this vigilance, ensuring that patient outcomes remain in tune with the highest standards.
6. User feedback and domain expert involvement #
Incorporating user feedback and domain experts’ insights is a symphony of collaboration. The virtuoso users, together with domain experts, compose an opus of insight, identifying nuances and subtleties that automated methods may overlook.
In the banking sector, this collaborative crescendo engages customer service representatives and enlists their expertise, spotlighting discrepancies in customer records and conducting a harmonious operation that resonates with accurate and consistent data management.
Effective assessment of data quality issues requires a multi-faceted approach, incorporating data profiling, auditing, validation, comparison, monitoring, and engagement with domain experts and users.
By implementing these strategies, organizations can uncover and address data quality challenges, enhancing their ability to make accurate and reliable decisions.
Data quality issues in banking and their solutions #
The world of banking operates on a foundation of trust and precision, driven by data-driven insights. However, within this intricate landscape, data quality issues emerge as silent disruptors, threatening to undermine operational efficiency, erode customer confidence, and sway strategic decision-making.
In this segment, we will uncover the distinctive data quality challenges that the banking industry faces, exploring their impact and unveiling strategies for resolution.
Some of the major data quality issues and solutions with examples #
Here are some of the examples of major data quality issues and solutions include:
- Inaccurate customer records
- Inconsistent reporting across departments
- Compliance risks due to inaccurate KYC data
- Delayed fraud detection
Let’s explore the examples of data quality issues and solutions in detail.
1. Inaccurate customer records #
Problem: Over time, due to manual data entry and lack of validation mechanisms, customer records in the bank’s database have become riddled with errors. These errors range from misspelled names to incorrect contact information, making it challenging to provide personalized services and accurate communication to customers.
Solution: Implement an automated data validation process at the point of entry for customer information. This process can include real-time validation of names, addresses, and contact numbers. Additionally, invest in data cleansing tools that regularly scan and rectify discrepancies in existing customer records. Regular data audits can help identify and correct inaccuracies, ensuring that customer data remains accurate and up to date.
2. Inconsistent reporting across departments #
Problem: Different departments within the bank generate reports using varying data sources, formats, and definitions. This inconsistency leads to confusion among decision-makers, impedes strategic planning, and undermines the bank’s credibility in the eyes of regulators and investors.
Solution: Implement a centralized data repository that acts as a single source of truth for all departments. Standardize data formats and definitions across the organization to ensure consistency. Introduce data integration tools that seamlessly gather data from various sources and transform it into a unified format.
Enforce data governance protocols that mandate data owners to validate data before it’s used for reporting. Regular training sessions can educate staff on proper reporting procedures and maintain uniformity.
3. Compliance risks due to inaccurate KYC data #
Problem: The bank’s Know Your Customer (KYC) data, which is crucial for regulatory compliance and risk assessment, contains inaccuracies and outdated information. This exposes the bank to non-compliance risks, potential legal actions, and regulatory penalties.
Solution: Establish a comprehensive data governance framework that includes regular audits of KYC data. Utilize data quality scorecards to monitor the accuracy and completeness of KYC records in real time.
Implement automated data validation processes for KYC information, ensuring that customer identities are accurately verified during onboarding and at periodic intervals. Collaborate with compliance experts to continuously update the bank’s data validation processes based on evolving regulatory requirements.
4. Delayed fraud detection #
Problem: The bank’s current fraud detection system relies on historical transaction data, which is updated infrequently. This delay in data updates results in delayed detection of fraudulent activities, potentially leading to financial losses and damage to customer trust.
Solution: Integrate real-time data feeds into the fraud detection system, allowing it to analyze transactions as they occur. Develop machine learning algorithms that can identify patterns indicative of fraudulent activities in real-time.
Collaborate with cybersecurity experts to implement additional layers of security, such as multi-factor authentication and real-time monitoring. Regularly update fraud detection models based on emerging fraud trends and continuously monitor their effectiveness.
These examples highlight the importance of proactive data quality management in the banking sector and the various solutions that can be employed to address these challenges effectively.
Healthy data quality practices in banking #
The path to rectifying these unique data quality challenges lies in fortified data governance. Automated data validation tools, rigorous entry protocols, and continuous monitoring are indispensable.
By instilling a culture of data quality consciousness and conducting regular audits, banks can foster customer satisfaction, mitigate risks, ensure compliance, and erect a robust foundation for making informed decisions.
As we progress, we will delve into the healthcare and big data sectors, unveiling their distinct data quality struggles and tailored strategies for overcoming them.
- Precision in regulatory reporting
- Detecting financial fraud
- Harmonizing inconsistent reporting
- Navigating regulatory compliance
- Breaking down data silos
- Seizing the timeliness of market data
Now, let us understand each of the above aspects in brief:
1. Precision in regulatory reporting #
Amidst regulatory complexities, data accuracy in reporting is paramount. Mismatched numbers or incomplete data can result in erroneous submissions, attracting regulatory scrutiny and financial penalties.
Maintaining data integrity is crucial to present a reliable financial picture, reassuring stakeholders and upholding the bank’s reputation.
2. Detecting financial fraud #
In the realm of banking, where digital interactions abound, data quality emerges as a vigilant guardian against financial fraud. However, when transaction data bears inaccuracies or omissions, fraudulent patterns can hide in plain sight.
Detecting unauthorized activities relies on high-quality data that reveals anomalies accurately, safeguarding the bank’s assets and customer trust.
3. Harmonizing inconsistent reporting #
The multifaceted landscape of banking operations often leads to discrepancies in data reporting across departments. This incongruence, fueled by varying data sources or formats, tarnishes the bank’s credibility.
Ensuring consistent and accurate data reporting is crucial to presenting a united front, both internally and to regulatory bodies.
4. Navigating regulatory compliance #
The regulatory dance within banking demands meticulous data management. Inaccurate customer information can lead to violations of anti-money laundering (AML) and know-your-customer (KYC) regulations.
Ensuring data quality becomes synonymous with adhering to these stringent rules, avoiding fines, and building a reputation for ethical business practices.
5. Breaking down data silos #
Banking operations, spanning diverse departments, often result in data fragmentation within isolated data silos. The absence of integrated data hinders a comprehensive view of customer interactions and financial trends.
The solution lies in dismantling these silos, enabling a panoramic perspective that fuels precise decision-making and enhances customer experiences.
6. Seizing the timeliness of market data #
The heartbeat of banking lies in real-time market insights. However, delayed or inaccurate market data integration can hamper timely decision-making, impacting investment opportunities and risk management.
Timely, accurate data is the currency that empowers the bank to navigate market dynamics swiftly and assertively.
Data quality issues in healthcare and their solutions #
The healthcare sector, driven by critical patient care and medical advancements, navigates a unique landscape of data quality challenges. Let’s delve into the specific data quality issues that healthcare encounters and uncover the intricacies that set them apart from other industries.
Some of the data quality issues with examples #
In the realm of healthcare, precision and accuracy are imperative, as lives hang in the balance. Unique data quality challenges arise in this sector, necessitating tailored solutions to ensure patient safety, optimize operations, and uphold compliance standards. These include:
- Patient identity management: Ensuring accurate identification
- Medical data accuracy: Safeguarding treatment decisions
- Interoperability and data sharing: Facilitating seamless exchange
- Data privacy and security: Safeguarding patient confidentiality
- Clinical decision support: Enhancing medical insights
- Regulatory compliance: Upholding legal standards
Let us understand them in detail.
1. Patient identity management: Ensuring accurate identification #
Problem: Mismatched patient records due to variations in data entry practices can lead to treatment errors and safety risks.
Solution: Implement robust patient identification protocols, such as biometric verification or unique identifiers. Utilize Master Patient Index (MPI) systems to reconcile duplicate records and maintain a single, accurate patient profile across all healthcare systems.
2. Medical data accuracy: Safeguarding treatment decisions #
Problem: Inaccuracies in medical records and test results can lead to incorrect diagnoses and compromised patient outcomes.
Solution: Integrate clinical decision support systems that cross-reference medical data with evidence-based guidelines. Implement automated data validation tools to flag inconsistencies or abnormal values in medical records for review by medical professionals.
3. Interoperability and data sharing: Facilitating seamless exchange #
Problem: Disparate data formats and interoperability challenges hinder efficient data sharing, affecting coordinated patient care and research efforts.
Solution: Adopt standardized data formats and health information exchange (HIE) protocols. Implement interoperable Electronic Health Record (EHR) systems that enable seamless data exchange across different healthcare providers while adhering to privacy regulations.
4. Data privacy and security: Safeguarding patient confidentiality #
Problem: Data breaches and unauthorized access can expose sensitive medical information, eroding patient trust and leading to legal repercussions.
Solution: Employ advanced encryption techniques to protect patient data during transmission and storage. Implement stringent access controls, requiring multi-factor authentication for medical professionals accessing patient records. Regularly audit systems for vulnerabilities and conduct staff training on data security best practices.
5. Clinical decision support: Enhancing medical insights #
Problem: Data quality impacts the accuracy of clinical decision support tools, potentially leading to suboptimal treatments.
Solution: Integrate artificial intelligence and machine learning algorithms that analyze vast medical datasets to provide evidence-based recommendations. Continuously validate and fine-tune these algorithms to ensure they reflect the latest medical knowledge and practices.
6. Regulatory compliance: Upholding legal standards #
Problem: Healthcare operates under strict regulatory frameworks like HIPAA and GDPR, demanding accurate data to ensure compliance.
Solution: Establish comprehensive data governance frameworks that outline data quality standards, ownership, and auditing procedures. Regularly conduct internal audits to identify and rectify data quality issues that could lead to regulatory non-compliance.
Healthy data quality practices in healthcare #
- Patient identity management
- Medical data accuracy
- Interoperability and data sharing
- Data privacy and security
- Clinical decision support
- Regulatory compliance
Now, let us understand each of the above aspects in brief:
1. Patient identity management #
Ensuring accurate patient identification is paramount, yet challenging due to variations in data entry practices and patient information discrepancies.
Mismatched records can lead to improper diagnoses, treatment delays, and potential safety risks.
2. Medical data accuracy #
Inaccuracies in medical records, test results, and treatment histories undermine healthcare decisions. Data entry errors or misinterpretations can lead to incorrect diagnoses, improper medications, and compromised patient outcomes.
3. Interoperability and data sharing #
Healthcare relies on seamless data exchange across systems, but inconsistent data formats and interoperability barriers hinder effective sharing. This can lead to fragmented patient records, hampering coordinated care and hindering medical research.
4. Data privacy and security #
Protecting patient confidentiality while ensuring data availability is a delicate balance. Data breaches or unauthorized access can expose sensitive medical information, eroding patient trust and leading to legal consequences.
5. Clinical decision support #
Healthcare professionals rely on accurate data for clinical decision-making. If data quality is compromised, medical decisions may be based on flawed information, leading to suboptimal treatments and potential patient harm.
6. Regulatory compliance #
The healthcare sector operates under strict regulatory frameworks like HIPAA and GDPR. Ensuring data quality is essential to meet these regulations, as errors or inconsistencies can result in legal penalties and reputational damage.
By embracing these bespoke solutions, healthcare organizations can transcend data quality challenges, fostering patient safety, optimizing medical practices, and ensuring compliance. In the upcoming segments, we will delve into the big data sector, unearthing its unique data quality intricacies and proposing strategies to navigate this expansive landscape.
What are the biggest quality issues in big data? #
The era of big data has ushered in unprecedented opportunities and challenges. Within this expansive landscape, data quality issues emerge as complex puzzles, demanding innovative solutions to harness the potential while mitigating risks. Let’s embark on a journey through the distinctive data quality challenges that loom over the world of big data.
Some of the biggest quality issues in big data include:
- Volume overwhelm and data overload
- Variety and inconsistent data formats
- Veracity and data accuracy
- Velocity and real-time data processing
- Value extraction and relevance
- Validity and trustworthy insights
It is imperative to understand them in detail:
1. Volume overwhelm and data overload #
The sheer volume of big data can lead to challenges in data storage, management, and processing. Unfiltered data influx risks diluting meaningful insights, while storage constraints can compromise data accessibility and analytical capabilities.
2. Variety and inconsistent data formats #
Big data comprises diverse data types, including structured, unstructured, and semi-structured data. The challenge lies in harmonizing these varied formats for coherent analysis. Inconsistencies hinder effective data integration, impeding accurate decision-making.
3. Veracity and data accuracy #
Big data often originates from multiple sources, with varying degrees of accuracy. Noise, errors, and incomplete data can skew analysis outcomes, leading to misguided insights and flawed strategies
4. Velocity and real-time data processing #
The rapid pace at which big data is generated demands real-time processing. Inaccuracies introduced during high-speed data ingestion and transformation can propagate through analysis, resulting in outdated or erroneous insights.
5. Value extraction and relevance #
Amidst the abundance of data, identifying relevant information is paramount. The challenge is to sift through the noise, extract valuable insights, and discard irrelevant data to make informed decisions.
6. Validity and trustworthy insights #
The proliferation of unverified or unstructured data in big data ecosystems can lead to questionable insights. The challenge lies in ensuring the validity of data sources and the credibility of insights drawn from them.
In conclusion #
Data quality has emerged as a top strategic priority. Subpar data can result in catastrophic outcomes in these sectors, highlighting the need for robust data governance, validation, and quality control.
By examining key data quality issues and best practices tailored to these industries, organizations can define targeted solutions to manage data more effectively. Ongoing governance and using modern tools for validation, monitoring, and lineage are imperative.
Data quality issues: Related reads #
- Data Quality Measures: Best Practices to Implement
- Data Privacy vs Data Security: How & Why They Aren’t Same?
- Data Management 101: Four Things Every Human of Data Should Know
- What is Data Stewardship?
- Cloud vs On-Premise vs Hybrid: Choosing the Right Data Management Approach
- 10 Steps to Achieve HIPAA Compliance With Data Governance
- Data Quality Explained: Causes, Detection, and Fixes
- 8 Data Governance Standards Your Data Assets Need Now
- What is Data Integrity and Why Should It Be a Priority of Every Data Team?
- Modern Data Management: 8 Things You Can Gain From It
Share this article