Predictive Data Quality: What is It & How to Go About It
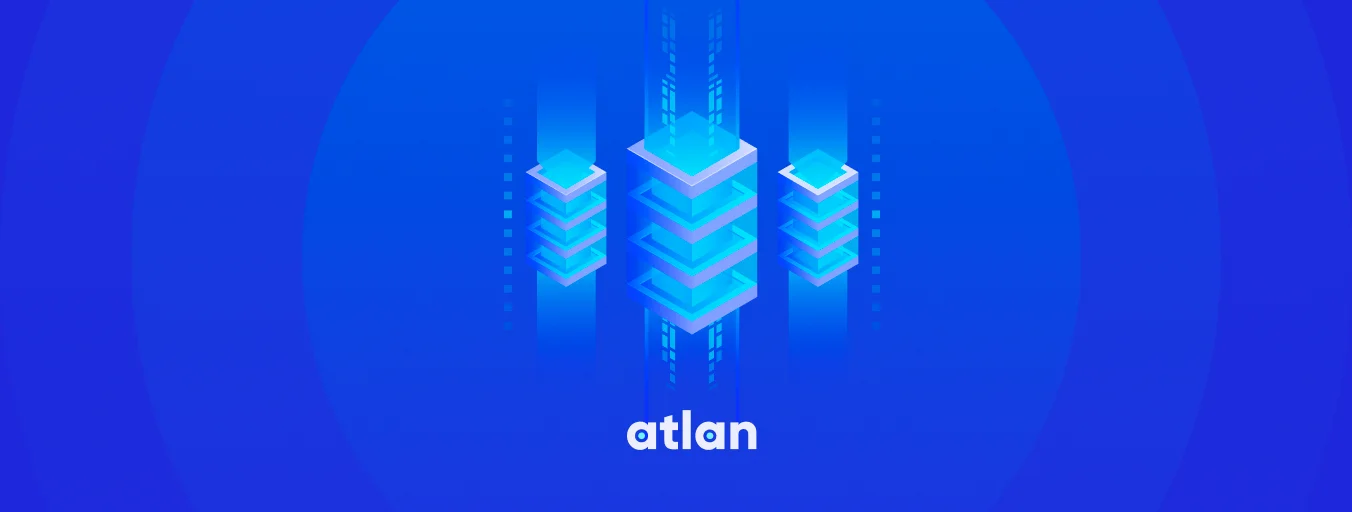
Share this article
What is predictive data quality? #
Predictive data quality refers to the assessment and improvement of data so that it is most useful and effective for predictive modeling or other types of data analytics. In other words, it is the set of practices that ensures the data you are using to make predictions is as accurate, complete, and timely as possible.
High-quality data is essential for predictive analytics because poor data quality can lead to inaccurate predictions, which in turn can lead to poor decision-making. So, in this article, we will understand what is predictive data quality, what are its pillars and benefits,
Let’s dive in!
Modern data problems require modern solutions - Try Atlan, the data catalog of choice for forward-looking data teams! 👉 Book your demo today
Table of contents #
- What is predictive data quality?
- The 7 pillars of predictive data quality
- The unignorable benefits of predictive data quality
- Predictive vs prescriptive data: What are the key differences?
- Predictive data quality in age of analytics and AI: 8 things to remember
- 9 Steps to implementing predictive data quality for data-driven businesses
- What is predictive analytics quality management and why does it matter?
- Summary
- Predictive data quality: Related reads
The 7 pillars of predictive data quality #
Predictive data quality is a set of practices and criteria aimed at ensuring that the data used in predictive modeling is of the highest quality possible. The goal is to maximize the accuracy and effectiveness of predictive analytics models by optimizing the underlying data.
The pillars of predictive data quality are:
- Data accuracy
- Data completeness
- Data consistency
- Data timeliness
- Data relevance
- Data integrity
- Data granularity
Let’s look into each of the above pillars in brief:
1. Data accuracy #
The foundational pillar of predictive data quality is data accuracy. This means ensuring that the values in your dataset genuinely represent what they are supposed to.
Incorrect or inaccurate data can be highly misleading, causing predictive models to make wrong assumptions and predictions. Data accuracy can be improved through validation techniques, error detection, and correction methods.
2. Data completeness #
A dataset should not have missing or incomplete information. Incomplete data can cause gaps in analysis and lead to incorrect or ineffective predictive models.
Imputation methods may be used to fill in missing values, but care must be taken to ensure that the imputed values do not distort the dataset’s underlying characteristics.
3. Data consistency #
Consistency in data is crucial when pulling information from multiple sources or when the data is stored in different formats. Inconsistent data can give mixed signals to the predictive model, reducing its effectiveness and accuracy.
Consistency checks, data harmonization, and maintaining a single source of truth are approaches to ensure data consistency.
4. Data timeliness #
Data timeliness refers to how current the data is. Using outdated or stale data for predictive analytics can lead to irrelevant or inaccurate results.
Scheduled data updates and real-time data integration techniques can help in maintaining the timeliness of the data.
5. Data relevance #
Not all data is relevant for every predictive model. Irrelevant features can introduce noise and lead to overfitting, where the model performs well on the training data but poorly on new, unseen data.
Feature selection techniques can help identify the most relevant variables for a specific predictive model.
6. Data integrity #
Data integrity involves the overall reliability, security, and governance of the data. This means that the data should not only be accurate but also trustworthy and protected from unauthorized access or manipulation.
Data governance policies and secure data storage solutions can help maintain data integrity.
7. Data granularity #
This refers to the level of detail in the dataset. Too much granularity might cause the model to catch random noise, leading to overfitting.
On the other hand, too little detail can lead to underfitting, where the model is too simplistic to capture the underlying trends. The right level of granularity is crucial for making accurate predictions and can be determined through techniques like dimensionality reduction.
By giving attention to these pillars, organizations can improve the quality of their data, thereby enhancing the performance and reliability of their predictive analytics models.
The unignorable benefits of predictive data quality #
The benefits of predictive data quality are numerous, extending not just to the accuracy of predictive models but also to an organization’s overall decision-making process.
Ensuring that your data is accurate, complete, timely, and relevant can have a significant impact on various aspects of your business.
The major benefits of predictive data quality include:
- Improved model accuracy
- Reduced risk of bad decisions
- Enhanced business operations
- Cost savings
- Better customer insights
- Compliance and risk management
- Competitive advantage
Let’s look into each of the above benefits in brief:
1. Improved model accuracy #
One of the most immediate benefits of predictive data quality is the improvement in the accuracy of predictive models. High-quality data eliminates a lot of the noise that can distort predictions, thereby increasing the likelihood that the model will generate reliable results.
This enables businesses to make more informed decisions based on insights that are truly reflective of their current situation or future prospects.
2. Reduced risk of bad decisions #
Poor-quality data can lead to incorrect analyses, which in turn can result in poor decision-making.
By ensuring predictive data quality, organizations can significantly reduce the risk of making bad decisions. This has the effect of enhancing business strategies and reducing the chances of financial or reputational damage.
For example, a retail business using high-quality data can more accurately forecast sales, allowing for better inventory management and marketing strategies.
3. Enhanced business operations #
High-quality data allows for more accurate and timely decision-making, which can enhance business operations in various ways, from supply chain optimization to customer relationship management.
For example, accurate predictive models can help in optimizing inventory levels, thereby reducing carrying costs and improving service levels.
4. Cost savings #
Poor data quality often results in redundant efforts to clean and validate data, which can be both time-consuming and costly.
Ensuring predictive data quality upfront can lead to significant cost savings by reducing the need for repetitive data cleansing processes and by avoiding the costs associated with bad decision-making.
For instance, an energy company can more accurately predict equipment failures, scheduling maintenance only when necessary, thus saving on operational costs.
5. Better customer insights #
High-quality predictive data can provide a more accurate understanding of customer behavior, needs, and preferences. This, in turn, enables better customer segmentation and targeting, which can lead to more effective marketing strategies and improved customer satisfaction.
For example, a streaming service could offer more accurate recommendations, increasing user engagement and satisfaction.
6. Compliance and risk management #
Poor data quality can result in compliance issues, especially in sectors like healthcare and finance where data integrity is highly regulated. Ensuring high predictive data quality can thus be essential in risk management and in avoiding costly regulatory penalties.
For example, healthcare organizations must adhere to strict data quality standards when predicting patient outcomes to comply with regulations like HIPAA in the United States.
7. Competitive advantage #
In today’s data-driven world, the quality of data can be a significant differentiator between competitors. Organizations that invest in predictive data quality have a better chance of developing more accurate and insightful models, giving them a competitive edge in the market.
For instance, an e-commerce company that can more accurately predict consumer buying habits can tailor marketing strategies that are more effective, thereby gaining market share.
By focusing on predictive data quality, organizations can unlock these benefits, driving not just better predictions but also better decision-making across the board.
Predictive vs prescriptive data: What are the key differences? #
The main difference between predictive and prescriptive data is in the type of insights they provide: Predictive data focuses on forecasting future outcomes based on historical data, while prescriptive data provides specific recommendations on ways to handle potential future scenarios.
Predictive data #
Predictive data is used primarily for forecasting future outcomes based on historical data and statistical algorithms. This can involve a variety of techniques including regression models, time-series analysis, and machine learning.
For example, a business may use predictive analytics to forecast sales for the next quarter based on past sales data.
Prescriptive data #
Prescriptive data goes a step further by not only forecasting outcomes but also providing specific recommendations for ways to handle potential future situations. This typically involves more complex analyses that take into account a variety of constraints and decision rules.
For example, in a supply chain setting, prescriptive analytics might recommend specific inventory levels for each product to minimize costs while meeting customer demand.
Let us look at the key differences between predictive data and prescriptive data in a tabular format:
Attribute | Predictive data | Prescriptive data |
---|---|---|
Objective | Forecasts what is likely to happen | Advises on how to best handle what is likely to happen |
Type of Insight | Descriptive and Inferential | Decision-oriented |
Complexity | Generally less complex | More complex due to inclusion of decision-making |
Data Sources | Historical data, current trends | Predictive data plus decision rules, constraints |
Real-time Adaptability | May or may not adapt in real-time | Often designed to adapt in real-time |
Use Case Example | Sales forecasting | Inventory optimization |
Analytical Techniques | Regression, time-series analysis, machine learning | Optimization algorithms, heuristics, decision-tree analysis |
Decision-making | Does not offer specific actions | Recommends specific actions |
Business Impact | Helps in planning and preparation | Directly influences actions and outcomes |
While both predictive and prescriptive data are integral to data-driven decision-making, they serve different roles. Predictive data provides forecasts that help with planning, whereas prescriptive data offers actionable recommendations that directly influence business outcomes.
Predictive data quality in age of analytics and AI: 8 things to remember #
Predictive data quality serves as the foundation for building reliable and effective analytics and AI models. When the data used for training and validating these models is of high quality, the resulting insights and predictions are more likely to be accurate and actionable.
Here’s how predictive data quality ensures trusted analytics and AI:
- Enhanced accuracy
- Reduced bias
- Improved generalization
- Increased consistency
- Robustness to noise
- Facilitates real-time decision-making
- Better compliance and governance
- Enhances risk management
Let’s look into each of the above aspects in brief:
1. Enhanced accuracy #
Predictive data quality helps in ensuring that the data fed into models is accurate, which directly affects the quality of the predictions. Accurate data leads to more reliable forecasts, increasing the trust stakeholders have in analytics and AI solutions.
2. Reduced bias #
High-quality, well-curated data can help in reducing the biases that can be inherently present in datasets. This is crucial for AI models, especially those used in sensitive applications like healthcare or criminal justice, where bias can have significant implications.
3. Improved generalization #
Quality data ensures that models are trained on a comprehensive and representative sample. This enhances the model’s ability to generalize well to new, unseen data, which is vital for the application of AI in real-world scenarios.
4. Increased consistency #
Consistency in data quality ensures that predictive analytics and AI models provide steady and reliable outcomes over time. This is particularly important for automated decision-making systems, which need to perform reliably under varying conditions.
5. Robustness to noise #
High-quality data is often cleaned to remove noise and outliers, making the analytics and AI models more robust. This is particularly important in environments where data can be noisy or when dealing with large datasets with diverse types of data.
6. Facilitates real-time decision-making #
Quality data that is timely and up-to-date is essential for real-time analytics and AI models. High-quality real-time data ensures that decisions are based on the most current information, increasing the trust in automated, real-time decision-making systems.
7. Better compliance and governance #
High predictive data quality often aligns with better data governance practices, ensuring that analytics and AI models comply with legal and ethical standards. This is essential for building trust, particularly in regulated industries.
8. Enhances risk management #
Predictive models based on high-quality data can more effectively identify potential risks, allowing for better risk management strategies. The reliability of such models is critical for building trust among stakeholders who depend on them for decision-making.
Example scenarios: #
- Healthcare: Predictive analytics can forecast patient readmissions. High-quality data ensures that these forecasts are reliable, which in turn helps hospitals allocate resources more efficiently.
- Finance: AI algorithms can predict stock market trends. If these algorithms are trained on high-quality data, investors are more likely to trust them with financial decisions.
- Retail: Personalized recommendation systems can significantly influence consumer behavior. The quality of predictions, and thereby the trust in such systems, is directly tied to the quality of the data used.
- Transportation: Predictive maintenance models can forecast when parts in vehicles are likely to fail. High-quality data ensures that these predictions are accurate, helping to avoid breakdowns and improve safety.
By adhering to the pillars of predictive data quality, organizations can build analytics and AI models that are not only accurate but also trusted by users and stakeholders, thereby enhancing their utility and impact.
9 Steps to implementing predictive data quality for data-driven businesses #
Implementing predictive data analytics is a multi-step process that requires careful planning, execution, and monitoring. Here are the key steps for data-driven businesses looking to implement predictive analytics:
- Identify business objectives
- Assemble the team
- Data collection
- Data cleaning and preprocessing
- Select the analytical model
- Train and validate the model
- Deploy the model
- Monitor and update the model
- Evaluate the ROI (Return on Investment)
Let’s look at each of the above steps in brief:
1. Identify business objectives #
The first step in implementing predictive analytics is to identify what you are trying to achieve. Are you looking to improve sales forecasting, enhance customer retention, or optimize supply chain management? Clearly defined objectives will guide the rest of the process.
2. Assemble the team #
Next, you need to assemble a team of experts who can contribute to different parts of the analytics pipeline. This usually includes data scientists, data engineers, business analysts, and domain experts. Collaboration among these specialists is crucial for the success of the project.
3. Data collection #
The quality of your analytics is directly tied to the quality of your data. Data can come from various sources such as databases, spreadsheets, external APIs, or directly from sensors. It’s crucial to ensure the data aligns with the business objectives identified earlier.
4. Data cleaning and preprocessing #
Raw data is rarely clean or in a format that’s ready for analysis. Data cleaning can involve removing duplicates, handling missing values, and converting data types. Preprocessing may include normalization, transformation, and feature selection, all aimed at improving the model’s predictive performance.
5. Select the analytical model #
Based on the business objectives and the nature of the data, choose an appropriate predictive model. This could be a linear regression model, a decision tree, a neural network, or any other predictive algorithm. Often, several models are tested to find the one that offers the best performance.
6. Train and validate the model #
Using historical data, the chosen model is trained to make predictions. It is crucial to validate the model using a separate set of data that it hasn’t seen before. Metrics like accuracy, precision, and recall can be used to evaluate the model’s performance.
7. Deploy the model #
Once validated, the model is deployed into a production environment where it can start making real-time predictions. This often involves integration with existing business systems and databases.
8. Monitor and update the model #
Predictive models are not set-it-and-forget-it solutions. They need to be regularly monitored for performance and updated as necessary. New data should be fed into the model to keep it current, and occasional retraining may be required.
9. Evaluate the ROI (Return on Investment) #
Finally, the effectiveness of the predictive analytics implementation should be evaluated in terms of ROI. This involves comparing the benefits gained against the costs incurred, often through key performance indicators (KPIs) that were identified at the outset.
Now, let us look at some practical examples where it can be implemented readily:
- Retail Business: The objective may be to improve inventory management. Data from past sales, seasons, and trends would be collected and cleaned. Various models could be tested to predict future sales, and the best-performing one would be integrated into the existing inventory management system.
- Healthcare: The goal might be to predict patient readmissions. The team would collect historical data on patient records, treatments, and previous admissions. A predictive model could then be trained and validated to forecast the likelihood of readmission for new patients.
- Finance: For credit scoring, historical financial data would be collected and cleaned. Various algorithms could be used to predict the creditworthiness of new applicants. The model would then be deployed and regularly updated to reflect economic conditions and customer behavior.
By following this step-by-step approach, data-driven businesses can implement predictive analytics effectively, ensuring that they derive actionable insights that align with their strategic objectives.
What is predictive analytics quality management and why does it matter? #
Predictive analytics quality management focuses on ensuring that the analytics processes and models produce reliable, accurate, and actionable results. This involves multiple steps, from data gathering to model deployment, all aimed at ensuring the integrity and efficacy of predictive analytics efforts.
Here’re the key points:
- Data quality assessment
- Model selection and validation
- Interpretability and explainability
- Scalability and performance
- Monitoring and maintenance
- Compliance and governance
- User adoption and training
Let’s look into each of the above points in brief:
1. Data quality assessment #
The first step in predictive analytics quality management is assessing the quality of the data. This involves checking for completeness, consistency, and accuracy. Without high-quality data, even the most sophisticated models will yield unreliable results. Businesses should also check for biases in the data that may lead to unfair or skewed predictions.
2. Model selection and validation #
Choosing the right model for the predictive analytics task is crucial for obtaining accurate results. Models should be validated using a separate dataset to check for overfitting and to ensure they generalize well to new, unseen data.
3. Interpretability and explainability #
In many domains, it’s not enough for a model to make accurate predictions; it also needs to be understandable by human users. Interpretability and explainability become critical factors in the quality management of predictive analytics, especially in regulated industries like healthcare and finance.
4. Scalability and performance #
As a business grows, its predictive analytics solutions must be able to scale with it. Quality management involves assessing the performance and scalability of the model to ensure it can handle increasing data volumes and computational loads.
5. Monitoring and maintenance #
A predictive model’s performance can degrade over time if it’s not regularly updated with new data. Continuous monitoring and maintenance are crucial for ensuring that the model remains accurate and relevant.
6. Compliance and governance #
In regulated industries, predictive analytics models must comply with legal and ethical standards. Quality management thus includes ensuring that data usage and model decisions meet all governance and compliance requirements.
7. User adoption and training #
For predictive analytics to be effective, it must be adopted and used correctly by the intended users. Quality management includes training for users and stakeholders and checking that the system is accessible and easy to use.
Predictive analytics quality management is a comprehensive approach to ensuring that predictive models are reliable, scalable, and actionable. It helps organizations derive the most value from their predictive analytics initiatives, ensuring they make more accurate decisions, stay compliant, and remain competitive in a data-driven world.
Summary #
Predictive data quality is crucial for ensuring the accuracy and reliability of analytics and AI models. High-quality data enhances decision-making, reduces costs, and builds stakeholder trust. It’s essential for minimizing bias, improving generalization, and adhering to compliance standards. Investing in predictive data quality elevates the impact of analytics, making organizations more informed, agile, and competitive.
Predictive data quality: Related reads #
- Data Quality Measures: Best Practices to Implement
- Data Quality Explained: Causes, Detection, and Fixes
- Data Quality Fundamentals: Why It Matters in 2023!
- A Blueprint for Bulletproof Data Quality Management
- Data Quality Issues? Here Are 6 Ways to Fix Them!
- How to Improve Data Quality: Strategies and Techniques to Make Your Organization’s Data Pipeline Effective
- How to Ensure Data Quality in Healthcare Data: Best Practices and Key Considerations
- Data Quality in Data Governance
- 6 Popular Open Source Data Quality Tools in 2023: Overview, Features & Resources
- Automated quality control of data pipelines
- Resolving Data Quality Issues in the Biggest Markets
- Bad Data: How It Sneaks in & How to Manage It in 2023
- 6 Reasons Why Data Quality Needs a Data Catalog
Share this article