Reverse ETL and Data Observability: Do They Matter in 2024?
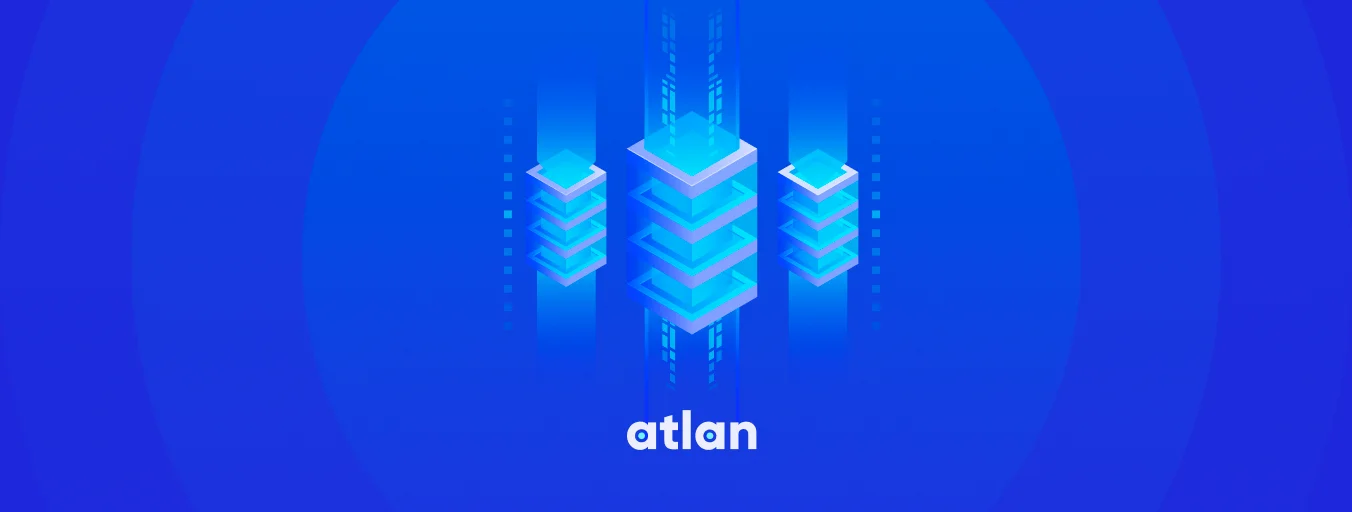
Share this article
With reverse ETL, organizations can seamlessly synchronize customer insights, analytics results, and other critical data back into their CRM, marketing automation, or other operational tools, leading to improved decision-making and enhanced customer experiences.
On the other hand, data observability alleviates the pain of data inaccuracies, incompleteness, or inconsistencies, ensuring that data-driven decisions are based on trustworthy information.
Modern data problems require modern solutions - Try Atlan, the data catalog of choice for forward-looking data teams! 👉 Book your demo today
Together, reverse ETL and data observability provide a powerful combination that streamlines data integration and ensures data integrity, ultimately driving better business outcomes and enabling organizations to stay agile and competitive in the data-centric era.
In this article we will understand how Reverse ETL and data observability complement each other in long run. Let’s dive in!
Table of contents #
- What is reverse ETL and how is it different from ETL?
- What is data observability?
- Reverse ETL vs. Data observability: How are they different?
- The Essentiality of data observability for your reverse ETL journey
- 5 Ways to harmonize data observability and reverse Etl
- Summing up
- Data observability and Reverse ETL: Related reads
What is reverse ETL and how is it different from ETL? #
Reverse ETL is an evolving technology framework that facilitates the movement of data from analytics warehouses back to operational systems. While traditional ETL (Extract, Transform, Load) moves data from source systems into data warehouses for analytics, reverse ETL takes the opposite route.
The key things to understand about reverse ETL:
- Data flow direction
- Use cases
- Components
- Benefits and challenges
1. Data flow direction #
Traditional ETL processes extract data from operational databases, transform it, and load it into a data warehouse for analysis. Reverse ETL does the opposite:
- It extracts data from analytics warehouses
- Transforms it as needed
- Sends it back to operational systems
This completes a full data loop within the organization.
2. Use cases #
The primary use case for reverse ETL is synchronizing enriched or transformed data back to operational systems.
For example, after conducting a customer segmentation analysis in a data warehouse, the enriched data can be sent back to a CRM for more targeted marketing activities.
3. Components #
Reverse ETL involves several components including the source data warehouse, transformation logic, and destination operational systems. Each component has its own set of protocols and requirements that need to be managed for successful data flow.
4. Benefits and Challenges #
The benefits of reverse ETL include better data consistency, more powerful analytics, and more effective operational strategies. However, challenges include ensuring data quality, managing data security, and overcoming integration hurdles between different systems.
Difference between ETL and reverse ETL #
Aspect | ETL | Reverse ETL |
---|---|---|
Data flow direction | Operational systems to data warehouse | Data warehouse to operational systems |
Purpose | Analytics, reporting, data science | Synchronizing enriched data back to operational systems |
Source system | Operational databases, CRM, ERP, etc. | Analytics data warehouse |
Destination system | Analytics data warehouse | Operational databases, CRM, ERP, etc. |
Transformation | Data cleansing, aggregation, enrichment before loading | May involve de-aggregation, filtering, simplifying |
What is data observability? 5 Pillars to know #
Data observability is an aspect of data governance focused on monitoring and understanding the state of data in real time. It helps organizations ensure that their data is accurate, available, and actionable. This is critical for analytics, machine learning models, and operational processes that rely on high-quality data.
- Data freshness
- Data quality
- Data lineage
- Data distribution
- Data cataloging
Let us understand them in detail:
1. Data freshness #
Data freshness refers to how current the data in a system is. It’s crucial for applications requiring real-time information, such as analytics dashboards or automated trading systems. Metrics like lag time between data creation and data availability help measure freshness.
Inaccurate or outdated data can severely affect decision-making and system responses. Therefore, monitoring data freshness is essential for maintaining the integrity of real-time operations.
2. Data quality #
Data quality involves various attributes like accuracy, consistency, completeness, and reliability. It impacts analytics, reporting, and decision-making processes. Monitoring tools flag issues like missing values or inconsistent formats.
Poor data quality can cause analytical errors, mistrust in systems, and faulty business decisions. Hence, ensuring data quality is a foundational aspect of data governance.
3. Data lineage #
Data lineage is about tracking the journey of data from its source to its final destination, including all transformations it undergoes. This is vital for debugging issues, ensuring data integrity, and compliance management.
Understanding lineage helps organizations maintain accurate data, facilitate audits, and comply with regulations like GDPR. It provides transparency and trust in the data being used.
4. Data distribution #
Data distribution refers to the statistical distribution and layout of data across storage or processing nodes. It’s crucial for efficient resource utilization and meaningful analytics. Imbalanced data distribution can lead to bottlenecks or misleading analytics results.
Metrics for assessing distribution include counts, percentiles, and histograms. Proper understanding and management of data distribution are essential for both operational efficiency and analytical accuracy.
5. Data cataloging #
Data cataloging involves creating and managing a metadata repository or catalog for data assets. It facilitates easier data discovery, understanding, and management. A catalog may contain metadata, such as data types, ownership, and descriptions.
Good data cataloging practices make it easier for users to find the data they need, understand its context, and trust its quality. This leads to faster and more accurate decision-making across the organization.
Reverse ETL vs. data observability: How are they different? #
Though both Reverse ETL and Data observability play important roles in modern data governance frameworks, they serve distinct functions and focus on different aspects of data management. Understanding their differences is critical for optimizing data operations.
This table provides a concise overview of the core features and differences between Reverse ETL and Data Observability.
Feature/Aspect | Reverse ETL | Data observability |
---|---|---|
Primary focus | Data movement from analytical systems to operational systems | Monitoring and understanding data's state and quality |
Main function | Transferring enriched and analyzed data back into operational systems | Ensuring data quality, tracking, and understanding data throughout its lifecycle |
Key components | Source data warehouses, transformation logic, destination operational systems | Monitoring tools, quality assessment frameworks, real-time dashboards |
Outcome | Making analytical insights actionable in operational systems | Real-time understanding of data health and ensuring data's reliability and compliance |
Relevancy in compliance | Ensures that enriched data is compliant when moved back to operational systems | Provides data lineage and audit trails, crucial for regulatory compliance |
Operational impact | Enhances operational systems with enriched data, improving their functionality | Builds trust in data and ensures its freshness, quality, and understanding |
Tools examples | Reverse ETL platforms like High touch, Census, etc. | Data observability platforms like Monte Carlo, Data fold, etc. |
End goals | Seamless integration of analytical insights into operational systems | Improved data decision-making, compliance, and data issue resolution |
While both Reverse ETL and Data Observability are essential for modern data governance, they serve different but complementary roles. Reverse ETL is about data transportation, making analytics actionable, whereas Data Observability is about data quality and integrity, ensuring that data is reliable and compliant.
The essentiality of data observability for your reverse ETL journey #
As organizations increasingly rely on data analytics for decision-making, the role of Reverse ETL becomes crucial. It enables the flow of enriched data from analytical platforms back to operational systems. However, without Data Observability, the Reverse ETL journey can be fraught with risks such as data inconsistencies and compliance issues.
- Data quality checks
- Compliance and audit trails
- Real-time insights
- Optimization and efficiency
- Trust and accountability
Let us understand them in detail:
1. Data quality checks #
- Data quality checks in data observability ensure that the enriched data moved back to operational systems through reverse ETL is accurate and reliable.
- Without quality checks, erroneous data could disrupt business processes, leading to poor decision-making.
- By integrating data observability with reverse ETL, you can automatically flag inconsistencies, missing values, or outliers, thereby enhancing the integrity of the data being transferred.
2. Compliance and audit trails #
- In the context of reverse ETL, compliance and audit trails are indispensable for meeting regulatory requirements.
- Data observability offers a transparent data lineage that tracks each piece of data from the analytical warehouse back to the operational systems.
- This traceability is crucial for industries that are highly regulated, like finance and healthcare, where failure to comply can result in severe penalties.
3. Real-time insights #
- Real-time Insights are essential for operational systems that require up-to-date data.
- With data observability integrated into the reverse ETL process, you can ensure the freshness of the data being moved.
- This is vital for applications like real-time dashboards, customer relationship management (CRM) systems, or automated trading platforms, which rely on the most current data for their operations.
4. Optimization and efficiency #
- Optimization and Efficiency are vital in any data operation, including reverse ETL.
- Data observability helps in understanding how the data is distributed, allowing for load balancing and resource allocation during the transfer process.
- This results in quicker data transfers reduces system latency and ensures that you’re maximizing the use of your computational resources.
5. Trust and accountability #
- When data observability is integrated into reverse ETL processes, it fosters Trust and Accountability among stakeholders.
- Observability ensures that the data being moved is reliable and its lineage is transparent, thereby allowing for greater accountability in data handling.
- This is crucial for fostering a culture of data trustworthiness within an organization, making stakeholders more confident in data-driven decisions.
Data observability is not just an added feature but an essential component that complements and enriches your Reverse ETL journey. Implementing data observability within your reverse ETL processes provides a robust, trustworthy, and efficient data pipeline, critical for modern businesses.
5 Ways to harmonize data observability and reverse ETL #
Data observability and reverse ETL, while serving different purposes, are mutually beneficial when integrated into a data pipeline. The harmony between these two frameworks can significantly enhance data governance, quality, and operational efficiency.
- Pre-transfer data quality checks
- Real-time monitoring and alerts
- Compliance auditing integration
- Centralized dashboard
- Feedback loop for continuous improvement
Let us understand them in detail:
1. Pre-transfer data quality checks #
Before the reverse ETL process begins, data observability tools can scan the data for quality issues like duplicates or missing values. This ensures that only clean, reliable data gets transferred back into operational systems, enhancing decision-making.
2. Real-time monitoring and alerts #
Data Observability tools monitor the data in real time as it traverses the reverse ETL pipeline. Immediate alerts are generated for any discrepancies or issues, allowing for swift interventions, which in turn helps maintain data integrity and trust.
3. Compliance auditing integration #
Data Observability tracks each data element’s journey, creating a thorough audit trail. This is invaluable for regulatory compliance, ensuring that the data moved via reverse ETL is both transparent and meets governance standards.
4. Centralized dashboard #
A unified dashboard integrates metrics from both reverse ETL and data observability processes. This provides a holistic view of data quality and transfer efficiency, enabling easy monitoring and quick decision-making.
5. Feedback loop for continuous improvement #
Observability metrics can identify bottlenecks or inefficiencies in the reverse ETL process. This feedback is used to refine the reverse ETL system, ensuring ongoing improvements in both data quality and transfer speed.
Each of these aspects is critical for ensuring that data observability and reverse ETL work harmoniously to maintain a high-quality, efficient, and compliant data pipeline.
Harmonizing data observability and reverse ETL is essential for creating a robust, reliable, and compliant data ecosystem. By doing so, organizations can ensure that their data is not only actionable but also trustworthy, paving the way for data-driven excellence.
Summing up #
Reverse ETL and data observability serve complementary roles in modern data ecosystems. While Reverse ETL enables analytical enrichment of operational data, Observability provides real-time visibility into data health. Integrating the two creates a robust framework for data governance.
Pre-transfer quality checks by Observability platforms ensure only trusted data flows into operations. Real-time monitoring provides alerts on any issues, allowing rapid response. Compliance integration facilitates auditing, crucial for regulated industries. The centralized dashboard offers cross-functional visibility for continuous optimization.
Their integration provides the rigorous governance and quality needed to drive innovation while also mitigating risks. For organizations aiming to be insights-driven, this is a foundational capability on which data excellence can thrive.
Data observability and Reverse ETL: Related reads #
- Data Observability: Definition, Key Elements, & Benefits
- 12 Popular Data Observability Tools in 2024: An Overview
- Observability vs. Monitoring: How Are They Different?
- Data Observability for Data Engineers: What, Why & How?
- How Data Observability & Data Catalog Are Better Together
- Data Observability vs Data Testing: 6 Points to Differentiate
- Data Quality and Observability - Why Are They Important?
- Data Observability & Data Mesh: How Are They Related?
- Data Observability vs Data Cleansing: 5 Points to Differentiate
- 12 Popular Observability Tools in 2024
- What’s the difference between Reverse ETL and Customer Data Platforms (CDP)?
- What Is Reverse ETL and How Does It Enhance the Modern Data Stack?
- Reverse ETL Use Cases: 3 Primary Applications to Consider
- The 9 Best Reverse ETL Tools Compared in 2024
Share this article