Functions of a Data Catalog: Explained With Examples, Challenges and What the Future Promises
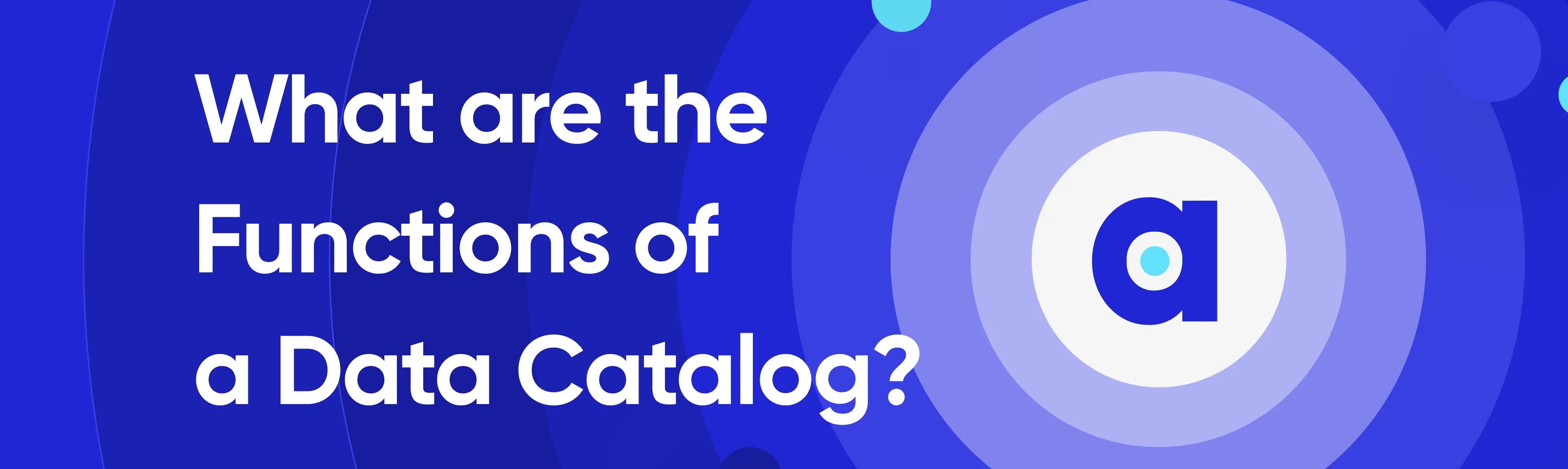
Share this article
A data catalog serves multiple functions, each playing a crucial role in unlocking the full potential of data assets in an organization. From managing diverse data assets to promoting data literacy, a data catalog acts as a centralized hub that empowers users to efficiently discover, understand, and utilize their data assets.
In this article, we will dive deep into the functions of a data catalog with examples, the challenges in its adoption, and the promise it holds in the near future.
Let’s dive in!
Table of contents #
- Exploring the functions of a data catalog
- Functions of a data catalog: Explained with examples
- Overcoming obstacles: Navigating challenges in implementing a data catalog
- What does the future of the data catalog look like?
- Bringing it all together
- Functions of a data catalog: Related reads
Exploring the functions of a data catalog #
In this section, we will delve into the various functions of a data catalog, exploring how it transforms the way organizations handle their data.
- Manage diverse data assets
- End-to-end data visibility
- Handle large scale metadata
- Facilitate embedded collaboration
- Contextualize data
- Data discovery
- Data governance
- Data security and privacy
- Promote data literacy
- Efficient data utilization
Let us look into each of the above functions of a data catalog in brief:
1. Manage diverse data assets #
- It goes beyond just managing tables, by intelligently storing and linking various types of data assets.
- This includes not just traditional data tables, but also BI dashboards, code snippets, SQL queries, models, features, and Jupyter notebooks.
2. End-to-end data visibility #
- The catalog provides a “single source of truth” about every data asset in the organization.
- It consolidates information typically spread across various tools (like data lineage tools, data quality tools, and data prep tools) into a unified, easy-to-access interface.
3. Handle large scale metadata #
- The catalog leverages the cloud to manage and process a vast amount of metadata. It essentially treats metadata as a form of data that can be searched, analyzed, and maintained.
- This includes:
- Parsing through query logs to generate insights such as column-level lineage
- Popularity scores for data assets, and
- Identification of potential owners or experts for each asset.
4. Facilitate embedded collaboration #
- The catalog enhances collaborative work by integrating with daily workflows, reducing friction, and enhancing efficiency.
- For instance, it may allow users to request access to a data asset seamlessly, or report issues that automatically trigger support requests integrated with existing workflows.
5. Contextualize data #
- It gives data context, making it easier for users to understand what the data represents and how it can be used.
- This includes providing information such as data definitions, relationships, origins, and transformations.
6. Data discovery #
- A significant function of the catalog is to facilitate data discovery, helping users find the data they need for analysis and decision-making.
- It accomplishes this through intelligent search capabilities and detailed metadata.
7. Data governance #
- The catalog enables better data governance, allowing for the implementation and maintenance of rules, policies, and standards that ensure the data is used appropriately and responsibly.
- It also contributes to better data quality management.
8. Data security and privacy #
- By keeping track of data assets and their use, the catalog helps ensure that data security and privacy rules are followed.
- It may also support access control, ensuring that only authorized individuals can access specific data assets.
9. Promote data literacy #
- The catalog provides a platform for users to learn more about the data assets available to them, promoting data literacy across the organization.
- This includes explaining complex data concepts in understandable terms and helping users get the most from the data assets.
10. Efficient data utilization #
- Lastly, the catalog aids in:
- Making data more accessible and usable
- Reducing the time and effort needed to understand and work with data, thereby increasing overall productivity and data utilization efficiency.
Functions of a data catalog: Explained with examples #
Now that we know the main functions of a data catalog, let us understand them better with examples.
1. Manage diverse data assets #
- For instance, a data catalog could store and interlink an SQL query used for customer segmentation, the Python script used to process the query’s output, and a Tableau dashboard that displays the processed data.
2. End-to-end data visibility #
- A data catalog could provide information about a data asset from its inception to its current state.
- This includes its origin, transformations it underwent, who accessed it, and how it was used in various analyses and reports, all accessible through a single interface.
3. Handle large scale metadata #
- For example, a data catalog could analyze the metadata from thousands of SQL queries executed in a month.
- This helps determining the most frequently accessed tables, commonly joined columns, and deducing the potential data owners or subject-matter experts based on query patterns.
4. Facilitate embedded collaboration #
When a data analyst finds an issue with a certain data asset in the catalog, they could directly create a ticket in the organization’s JIRA system from within the catalog, attaching relevant metadata and context, thereby speeding up issue resolution.
5. Contextualize data #
A data catalog could provide a complete context of a sales data table - explaining what each column represents, how the sales figures were calculated, any adjustments made, and perhaps a glossary of sales-related terms.
6. Data discovery #
- Users can leverage the catalog’s intelligent search feature to find specific datasets.
- For instance, typing “customer” might suggest “customer demographics”, “customer transaction history” or “customer behavior analysis” based on the metadata and previous search patterns.
7. Data governance #
- The catalog could enforce a policy that all data assets must have a designated owner and documented business definitions.
- It could alert when assets do not comply, promoting adherence to data governance standards.
8. Data security and privacy #
- The catalog could maintain information about who has access to sensitive data, such as personally identifiable information (PII)
- It can even alert when such data is accessed without proper authorization.
9. Promote data literacy #
- An analyst who is unfamiliar with a particular area, say “logistics data”, could use the data catalog to learn about the datasets available, their relevance, context, and appropriate use cases.
10. Efficient data utilization #
- A data analyst looking to understand customer behavior might discover through the catalog that a colleague in another department has already created a customer segmentation model.
- They could then leverage this existing model, saving time and effort.
Overcoming obstacles: Navigating challenges in implementing a data catalog #
Several factors may hinder organizations from fully utilizing the functions of a modern data catalog, such as:
- Data silos
- Lack of data governance
- Data quality issues
- Insufficient technical capabilities
- Resource constraints
- Resistance to change
- Data privacy concerns
- Scalability issues
- The complexity of integration
- Insufficient metadata management
Let us look into each of the above challenges in brief:
1. Data silos #
Information kept in isolated systems or departments can make it challenging to achieve end-to-end visibility and management of data assets.
2. Lack of data governance #
Absence of clear data governance policies and procedures can lead to unstandardized data, which makes cataloging and understanding data assets difficult.
3. Data quality issues #
Poor quality data, including incomplete, inconsistent, or duplicate data, can undermine the reliability of the data catalog.
4. Insufficient technical capabilities #
Implementing a modern data catalog requires certain technical competencies. Not having the necessary skill sets in-house can hamper the catalog’s development and management.
5. Resource constraints #
Building and maintaining a data catalog require time, money, and human resources. Resource constraints may limit an organization’s ability to develop a comprehensive and effective data catalog.
6. Resistance to change #
In some organizations, there might be resistance from employees who are used to certain ways of working with data. Overcoming this resistance and encouraging the adoption of a new system can be challenging.
7. Data privacy concerns #
With increasing data privacy regulations like GDPR, handling sensitive data becomes a concern. Ensuring the data catalog maintains privacy and meets regulatory compliance can be complex.
8. Scalability issues #
As the volume, variety, and velocity of data grow, keeping the data catalog updated and scalable can become increasingly challenging.
9. The complexity of integration #
Integrating the data catalog with existing systems, tools, and workflows might be technically complex and disruptive to ongoing operations.
10. Insufficient metadata management #
The inability to effectively manage large volumes of metadata, especially in organizations dealing with big data, can obstruct the full functionality of a data catalog.
What does the future of the data catalog look like? #
In this section, we will look at the potential functions of a data catalog in the imminent future. Let’s dive in!
- Improved contextual understanding
- Real-time data asset interaction
- In-app metadata enrichment
- Seamless integration with diverse tools
- Observability, cost management, and remediation
- Automated ownership attribution
- Automated pipeline management
- Data quality management
- Intelligent tuning of data systems
Let us look into each of the above aspects in detail:
1. Improved contextual understanding #
Active metadata could be utilized in real-time within a user’s workflow. For example, while using a BI tool, a user could receive immediate context about a dashboard or metric, without having to open a separate tool or search through metadata in a traditional data catalog.
2. Real-time data asset interaction #
With the implementation of active metadata, users could make real-time interactions with data assets. For instance, while browsing the lineage of a data asset, users could create a Jira ticket directly if they find an issue.
3. In-app metadata enrichment #
Active metadata could allow in-app enrichment of data assets. For example, while asking a question about a data asset in Slack, a bot could bring relevant context directly within Slack.
4. Seamless integration with diverse tools #
Active metadata could facilitate seamless integration with diverse tools like Jira, Slack, GitHub etc. enriching the user experience by providing context, lineage information, and dependency checks within these tools.
5. Observability, cost management, and remediation #
Active metadata could be used to monitor the health of data systems, manage costs by optimizing data pipelines and compute workloads, and remediate issues such as data quality or security incidents.
6. Automated ownership attribution #
Active metadata could automatically deduce the owners and experts for data tables or dashboards based on SQL query logs.
7. Automated pipeline management #
In case of a detected data quality issue, active metadata could automatically stop downstream pipelines, predict what went wrong based on past records, and fix it without human intervention.
8. Data quality management #
It could enable automated purging of low-quality or outdated data products, based on metadata information about data usage, relevance, and freshness.
9. Intelligent tuning of data systems #
Active metadata could auto-tune data pipelines and compute workloads by analyzing usage metadata, operational metadata, and end-to-end lineage information.
These advanced capabilities show the potential for active metadata to revolutionize the way data catalogs are used, making them an integral part of daily workflows rather than separate tools to be consulted when necessary. It’s a move towards a more intelligent and user-friendly data experience.
Bringing it all together #
In conclusion, a data catalog serves a wide range of functions that are essential for unlocking the full potential of data assets within an organization. It acts as a centralized hub, enabling efficient data management, discovery, governance, security, and utilization.
As businesses continue to recognize the value of data as a strategic asset, the functions of data catalogs will only become more critical. The future promises a data experience that is more intelligent, seamless, and transformative, empowering organizations to extract valuable insights and drive innovation through their data assets.
Functions of a data catalog: Related reads #
- What Is a Data Catalog? & Why Do You Need One in 2023?
- Essential Features of Data Catalogs
- Enterprise data catalog: Definition, Importance & benefits
- Data catalog benefits: 5 key reasons why you need one
- Open Source Data Catalog Software: 5 Popular Tools to Consider in 2023
- Data Catalog Platform: The Key To Future-Proofing Your Data Stack
- Top Data Catalog Use Cases Intrinsic to Data-Led Enterprises
- AI and Data Catalog: AI Data Catalog
- Benefits and Features of Snowflake: Snowflake Data Catalog
- dbt Data Catalog
- AWS Glue Data Catalog: Architecture, Components, and Crawlers
- Airbnb Data Catalog Democratizing Data With Dataportal
- Lexikon: Spotify’s Efficient Solution For Data Discovery And What You Can Learn From It
- Google Cloud Data Catalog Guide - Everything You Need to Know
Share this article