What is Data Product Management? Skills, Roles and Importance
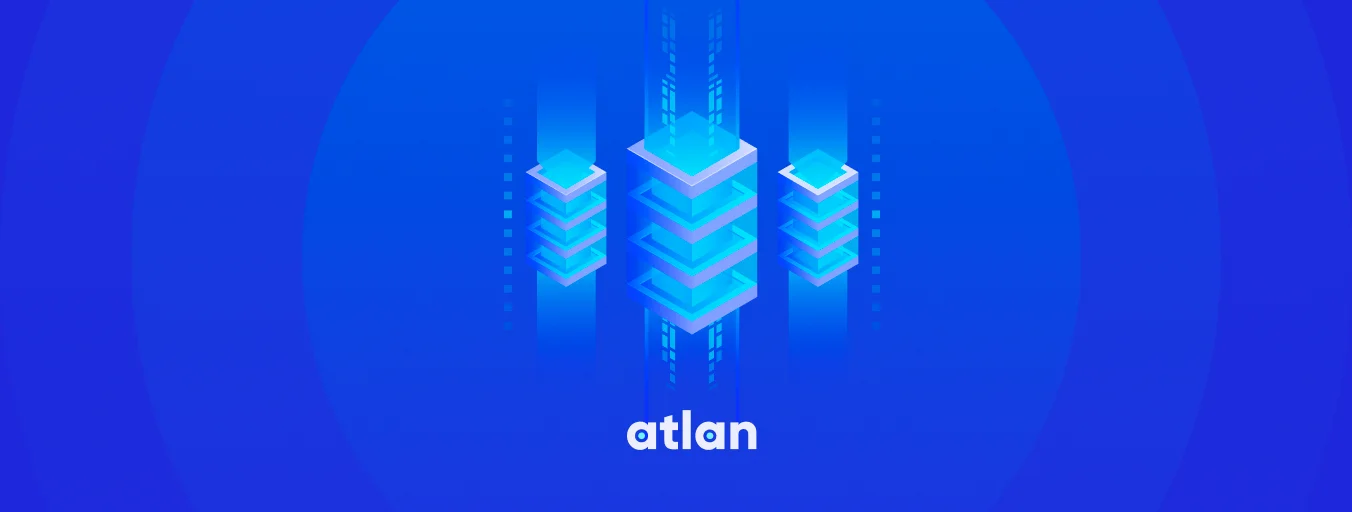
Share this article
If data products are not properly managed, they may yield inaccurate or misleading results. This can happen for a variety of reasons—ranging from poor data quality to inadequate data governance. This is where data product management helps you out!
Data product management is a field that merges the traditional principles of product management with the intricacies of data science and analytics. It is no longer just a supporting element but a full-fledged product that drives business growth. The key to unlocking this potential is data product management (DPM).
This multi-faceted role is your ticket to transitioning from raw data to actionable insights, thereby solving the very pain points that lead many businesses to failure.
Modern data problems require modern solutions - Try Atlan, the data catalog of choice for forward-looking data teams! 👉 Book your demo today
In this article, we will explore:
- What is data product management?
- Roles and responsibilities of a data product manager
- Steps to become one
- 8 Reasons why it is important
- How do data managers use data?
Ready? Let’s dive in!
Table of contents #
- What is data product management?
- Roles and responsibilities of a data product manager
- How to become a data product manager?
- Why does data product management matter? 8 Reasons to know!
- How do data managers use data?
- Unlocking the power of data product management systems for streamlined operations
- Data product management: 9 Step framework
- Product manager vs. data product manager: 10 Differences between them
- 9 Data product management courses and trainings to elevate your skill
- Conclusion
- What is data product management: Related reads
What is data product management? #
At its core, data product management focuses on creating, overseeing, and refining products powered primarily by data. Data product management is a dynamic and rapidly evolving discipline, central to the growth and innovation strategies of many modern businesses.
As organizations continue to recognize the transformative power of data, the role of data product management, act as a bridge between technical and non-technical realms, becomes ever more pivotal.
Roles and responsibilities of a data product manager #
Data product managers are instrumental in shaping the success of data-driven organizations, overseeing the development and management of data products and solutions. Their roles and responsibilities are multifaceted and require a combination of technical expertise, business acumen, and strong leadership skills. Here are key roles and responsibilities associated with this role:
- Define product strategy
- Roadmap development
- Requirements gathering
- Data governance
- Product development oversight
- User-centric focus
- Data monetization
- Performance monitoring
- Cross-functional collaboration
- Technology awareness
Now, let’s understand each of these roles and responsibilities in detail:
1. Define product strategy #
Data product managers play a critical role in defining the strategy for data products. They align these products with the overall business objectives and ensure they meet the needs of both internal and external stakeholders.
2. Roadmap development #
They create and maintain a roadmap for data product development, outlining the key milestones, features, and enhancements over time. This roadmap guides the product development team and keeps stakeholders informed.
3. Requirements gathering #
Data product managers collaborate with various teams, including data scientists, engineers, analysts, and business units, to gather and prioritize product requirements. They ensure that these requirements address business needs and align with the overarching strategy.
4. Data governance #
They establish and enforce data governance policies and standards to maintain data quality, security, and compliance. This includes defining data access controls and ensuring adherence to regulatory requirements.
5. Product development oversight #
Data product managers oversee the end-to-end development lifecycle of data products. They coordinate cross-functional teams, set project timelines, and ensure that products are delivered on time and within budget.
6. User-centric focus #
They maintain a deep understanding of user needs and continuously gather feedback to improve data product features and functionality. Prioritizing user satisfaction is essential for successful product adoption.
7. Data monetization #
Data product managers explore opportunities to generate revenue from data products. This may involve creating data licensing models, identifying new markets, or developing data-driven subscription services.
8. Performance monitoring #
After product launch, they monitor product performance using metrics and key performance indicators (KPIs). Data product managers use these insights to make data-driven decisions about product improvements and adjustments.
9. Cross-functional collaboration #
Effective communication and collaboration are vital aspects of the role. Data product managers work closely with various teams, ensuring that data product initiatives are aligned with the broader organizational goals.
10. Technology awareness #
Staying up-to-date with emerging data technologies and methodologies is crucial. Data product managers need to assess and adopt new tools and techniques to keep data products competitive and innovative.
In essence, data product managers serve as the bridge between data technology and business outcomes. They are responsible for ensuring that data products are not only technically sound but also aligned with the strategic vision of the organization, delivering value to users, and contributing to the organization’s overall success.
How to become a data product manager? #
Becoming a data product manager involves a combination of education, experience, and acquiring specific skills. Here are the steps to help you become a data product manager:
- Obtain a relevant bachelor’s degree
- Gain experience in data and product management
- Learn data technologies
- Develop business acumen
- Build project management skills
- Improve communication skills
- Pursue a master’s degree
- Network in the industry
- Apply for data product manager positions
- Continuously learn and adapt
- Develop a portfolio
- Ace interviews
Now, let’s look at each of these steps in detail:
1. Obtain a relevant bachelor’s degree #
Start with a strong educational foundation. A bachelor’s degree in a related field such as computer science, data science, business, or engineering can provide essential knowledge and skills.
2. Gain experience in data and product management #
To become a data product manager, you typically need prior experience in data-related roles, product management, or a combination of both. Begin by working in roles such as data analyst, data engineer, or product associate to gain hands-on experience.
3. Learn data technologies #
Familiarize yourself with data technologies and tools commonly used in the industry, such as data analytics platforms, data visualization tools, and data management systems. This knowledge will be valuable in your role as a data product manager.
4. Develop business acumen #
Data product managers need a strong understanding of business operations, strategy, and market dynamics. Consider taking courses in business administration or gaining experience in business-related roles.
5. Build project management skills #
Effective project management is crucial for overseeing the development of data products. Consider obtaining project management certifications such as PMP or agile/scrum certification to enhance your skills in this area.
6. Improve communication skills #
Strong communication skills are essential for conveying complex technical concepts to non-technical stakeholders and collaborating effectively with cross-functional teams. Practice written and verbal communication, including data storytelling.
7. Pursue a master’s degree (Optional) #
While not always required, pursuing a master’s degree in data science, data analytics, or business administration (MBA) can enhance your qualifications and open up more advanced opportunities.
8. Network in the industry #
Attend industry conferences, seminars, and meetups to network with professionals in the data and product management fields. Building a professional network can lead to job opportunities and valuable insights.
9. Apply for data product manager positions #
Start applying for data product manager roles as you gain relevant experience and skills. Be prepared to showcase your past achievements and demonstrate how your background aligns with the responsibilities of a data product manager.
10. Continuously learn and adapt #
The field of data and product management is constantly evolving. Stay up-to-date with industry trends, new technologies, and best practices. Consider taking online courses, attending workshops, or pursuing certifications to stay competitive in the field.
11. Develop a portfolio #
As you gain experience, create a portfolio of successful data product management projects and outcomes. This can be a powerful tool during job interviews and when seeking career advancement.
12. Ace interviews #
Prepare for interviews by studying common data product manager interview questions and practicing your responses. Showcase your problem-solving abilities, leadership skills, and data expertise.
Becoming a data product manager is a rewarding journey that requires dedication, continuous learning, and a passion for leveraging data to drive business success. Remember that each individual’s path may vary, so adapt these steps to your unique circumstances and career goals.
Why does data product management matter? 8 Reasons to know! #
Data product management (DPM) stands at the forefront of this revolution, bridging the gap between raw data and actionable insights. But what does DPM entail, and how does it transform the way organizations leverage their data? Let’s delve into the profound benefits of integrating data product management practices.
Here’s why data product management matters:
- Centralized data strategy
- Lifecycle management of data products
- Cross-functional collaboration
- Prioritization and resource allocation
- Data governance and security
- Iterative development and feedback loops
- Integration with business ecosystems
- Adherence to ethical and regulatory standards
Let us look into each of the above points in detail.
1. Centralized data strategy #
Think of DPM as the grand orchestrator of a symphony, where every instrument, or in this case, every data-driven initiative, plays in harmony. From basic analytics dashboards to intricate machine learning models, DPM ensures all these “instruments” are in tune with the broader business strategy.
The result? A unified data narrative where every project, irrespective of its scale or domain, aligns seamlessly, eliminating the chaos of disjointed data efforts. This coherence ensures clarity, prevents redundant efforts, and amplifies the potency of data-driven initiatives.
2. Lifecycle management of data products #
Just as a product manager oversees a product from its infancy to its culmination, a data product manager curates the journey of data products. Beginning from the nebulous stage of ideation, traversing through the rigors of data collection, model training, and reaching the zenith of deployment - every stage is meticulously managed.
Such diligent oversight ensures that these data products remain attuned to ever-evolving business requisites, continually delivering optimal value.
3. Cross-functional collaboration #
The realm of data isn’t an isolated fortress; it thrives on collaboration. DPM serves as a bridge connecting the technical realm of data scientists and engineers with business stakeholders and the end-users.
This symbiotic relationship ensures that the data products birthed are not just algorithmically sound but are also steeped in real-world applicability. By fostering a culture of open dialogue and collaboration, DPM ensures swift iterations and enhances the real-world efficacy of data solutions.
4. Prioritization and resource allocation #
In the vast expanse of data, not every star shines the brightest. DPM plays the critical role of the astronomer, discerning the luminous, high-impact data projects from the dim ones.
By astutely prioritizing projects and channeling resources to these pivotal endeavors, data product managers ensure maximized returns on data investments, focusing on projects that resonate with business impact.
5. Data governance and security #
With data comes power, and with power, accountability. The data sanctity, data accuracy, and data security cannot be compromised. DPM, recognizing this imperative, erects robust data governance structures.
These protocols ensure that data is ethically procured, processed, and deployed. Moreover, in an era marred by data breaches, DPM emphasizes uncompromising security measures, safeguarding an organization’s data assets and reputation.
6. Iterative development and feedback loops #
Static is antithetical to the world of data. As business landscapes pivot, so must the data products. DPM champions the ethos of agility, promoting iterative development.
This iterative lens, complemented by robust feedback mechanisms, ensures data products are continually refined, adapting to changing business terrains and user feedback, guaranteeing sustained relevance.
7. Integration with business ecosystems #
Data products, in isolation, are akin to unplayed pianos – full of potential but silent. DPM ensures these products sing by integrating them seamlessly into the broader business ecosystem.
Be it a marketing automation tool, a CRM system, or an ERP suite; DPM ensures fluid interoperability, ensuring a cohesive and streamlined business operation.
8. Adherence to ethical and regulatory standards #
In the data domain, ethics and compliance aren’t mere checkboxes; they’re pillars. DPM anchors itself in these pillars, ensuring every data product is crafted, deployed, and managed within the bounds of ethical guidelines and regulatory frameworks.
This commitment not only fosters trust but ensures businesses remain unscathed in an era of stringent data regulations.
Data product management is the unsung hero in the concert of modern businesses. It harmonizes the technical and the tactical, the algorithmic and the actionable.
How do data managers use data? #
Data Managers play a crucial role in harnessing the power of data to drive decision-making, enhance efficiency, and uncover new opportunities. Here’s how they effectively use data:
- Data collection and storage
- Data cleaning and maintenance
- Data analysis and interpretation
- Data security and compliance
- Reporting and communication
- Strategic planning
- Cross-functional collaboration
- Technology integration
- Training and development
Let us understand how data managers use data in detail.
1. Data collection and storage #
Data managers begin by establishing robust systems for data collection, ensuring the data is accurate, relevant, and comprehensive. They oversee the storage of this data in secure, accessible databases, often using cloud technologies for scalability and ease of access.
2. Data cleaning and maintenance #
A key responsibility is to maintain the quality of data. This involves regular cleaning and validation to remove inaccuracies, duplicate entries, and outdated information, thereby ensuring the data’s reliability and usefulness.
3. Data analysis and interpretation #
They analyze data to extract meaningful insights. Using various tools and techniques like statistical analysis, trend spotting, and predictive modeling, Data managers turn raw data into actionable information.
4. Data security and compliance #
Ensuring the security of data and compliance with relevant data protection laws is paramount. Data managers implement security protocols, conduct regular audits, and keep abreast of legal requirements to protect sensitive information.
5. Reporting and communication #
They transform data insights into clear, concise reports for stakeholders. By presenting data in an understandable format, they facilitate informed decision-making across the organization.
6. Strategic planning #
Data managers use data to inform strategic business decisions. They identify trends, forecast future scenarios, and provide recommendations that align with the organization’s goals.
7. Cross-functional collaboration #
They often work closely with other departments, such as marketing, finance, and operations, to ensure that data-driven strategies are implemented effectively throughout the organization.
8. Technology integration #
Staying updated with the latest data management tools and technologies, they integrate advanced systems like AI and machine learning to enhance data analysis capabilities.
9. Training and development #
They also play an educational role, training staff in data literacy and the proper use of data systems, promoting a data-driven culture within the organization.
In summary, Data managers are the linchpins in an organization’s data strategy, leveraging data to unlock potential, drive innovation, and guide the organization towards its goals.
Unlocking the power of data product management systems for streamlined operations #
In the contemporary business landscape, data isn’t just an asset—it’s a transformative force, driving innovation and charting new frontiers. Data product management, pivotal in this revolution, bridges the chasm between raw data and actionable insights. Data product management system (DPMS) is the toolkit that turbocharges DPM efforts.
The dynamic capabilities of data product management systems include:
- Customization and scalability
- Real-time monitoring and analytics
- Integration with data sources
- User access control
- Collaboration features
- Automated workflows
- Support for advanced algorithms
- Enhanced data security
Let us look into each of the above capabilities in brief:
1. Customization and scalability #
Every business is unique, presenting its own set of challenges, goals, and operational nuances. Data product management systems aren’t one-size-fits-all solutions; they are chameleons, morphing to align with a company’s bespoke requirements.
Whether it’s customizing dashboard visuals for a retail giant or tweaking algorithms for a fintech startup, DPMS adapts. As a business burgeons, the system gracefully scales, ensuring that growing data needs are effortlessly catered to, all without compromising performance.
2. Real-time monitoring and analytics #
In the fast-paced world of data, yesterday’s insights are often stale. The power lies in the ‘now.’ DPMS offers the pulse of real-time insights. This live feed enables managers to understand how their data products are being used, their current performance metrics, and potential areas begging for enhancement.
The immediacy ensures agile decision-making, pivoting strategies on the fly to harness opportunities or mitigate challenges.
3. Integration with data sources #
Data product management doesn’t operate in silos. It’s interconnected, drawing from a myriad of data sources. DPMS are akin to vast digital rivers, fed by multiple streams of data sources.
Whether it’s integrating with a CRM, an ERP, or even real-time sensors, these systems ensure a continuous and seamless inflow of data. This convergence means richer insights, holistic views, and a more integrated approach to data-driven strategies.
4. User access control #
With great data comes great responsibility. Not every stakeholder should have unrestricted access to all data facets. DPMS understands this nuanced requirement, weaving in robust access control features.
Data product managers can granularly define access parameters—deciding who gets to see what, and more importantly, who gets to modify what. This stratified access doesn’t just safeguard data but also ensures process integrity.
5. Collaboration features #
The essence of data product management is collaboration. It’s a symphony where data scientists, business analysts, and strategy heads co-create. DPMS is embedded with collaborative features that foster this co-creation.
Whether it’s a shared annotation on a data model, real-time feedback loops, or joint debugging sessions, these features ensure that teams can work in tandem, driving synergies.
6. Automated workflows #
Repetitive tasks can be a drain on resources and time. Recognizing this, DPMS brings to the table powerful automation features. Tasks that were once manual—like data ingestion, preliminary processing, or periodic reporting—are now automated.
This automation ensures not just efficiency but also consistency, eliminating the scope for human-induced errors and freeing up resources for more strategic endeavours.
7. Support for advanced algorithms #
Modern businesses aren’t content with rudimentary analytics. They’re exploring the frontiers of machine learning, deep learning, and predictive modeling.
DPMS is tailored to support these advanced explorations. They offer the infrastructure and tools to deploy, test, and monitor intricate algorithms, ensuring businesses remain at the cutting edge of data-driven innovations.
8. Enhanced data security #
Data breaches can be catastrophic, both in terms of financial repercussions and brand reputation. DPMS places an uncompromising emphasis on data security.
Through multi-layered encryption, stringent authentication protocols, and regular security audits, these systems ensure the sanctity of data, giving businesses the peace of mind to innovate without trepidation.
At the heart of every successful data product management endeavor lies a robust system, facilitating, empowering, and amplifying efforts. As businesses globally recognize the potency of data, DPMS stands as the beacon, guiding them toward data-driven excellence.
Data product management: 9 Step framework #
In an era where data drives decisions, data product management (DPM) stands out as the compass guiding businesses towards effective utilization of their data resources.
It’s not just about managing vast amounts of data, but the strategic synthesis of data science and product management.
Here are the points that you need to consider to build a solid data product management framework:
- Clear objectives
- Stakeholder collaboration
- User-centric design
- Data integration
- Iterative development
- Ethical considerations
- Scalability
- Skill development
- Future-proofing
Let us look into each of the above points in brief:
1. Clear objectives #
In the realm of data product management, clarity of purpose is paramount. Companies should not merely aim to have data products but understand why they need them. Is the objective to boost sales, enhance customer engagement, or streamline internal processes?
Or perhaps all three? Setting clear objectives ensures that the entire product development process, from data collection to product launch, aligns seamlessly with the company’s broader vision and goals.
2. Stakeholder collaboration #
Data products are not built in isolation. Their development necessitates a symphony of collaboration between different teams. Data scientists might understand the intricacies of algorithms, but marketers provide insights into customer preferences, while sales teams shed light on market dynamics.
Bringing these diverse perspectives together ensures the development of a holistic product that addresses multi-dimensional challenges.
3. User-centric design #
A product’s success is inherently tied to its user acceptance. DPM must prioritize users, delving deep into their behaviors, needs, and challenges. It’s about crafting solutions that resonate, ensuring users don’t just adopt, but advocate for the data product.
This involves iterative user testing, feedback collection, and understanding the nuances of the user journey.
4. Data integration #
In today’s digital ecosystem, data is scattered across various touchpoints. From CRMs to social media insights, integrating these disparate data sources is pivotal.
This amalgamation provides a comprehensive view, paving the way for richer insights and enabling data products to paint a holistic picture that drives informed decisions.
5. Iterative development #
The world of data is dynamic, and so should be the approach to product development. An agile methodology, characterized by short development cycles and frequent feedback loops, ensures that data products remain adaptive.
By building prototypes and beta versions, businesses can gauge user reactions and make requisite refinements, ensuring the end product is both robust and relevant.
6. Ethical considerations #
The power of data brings with it significant responsibility. In a world increasingly conscious of privacy and data rights, ensuring ethical data practices is non-negotiable.
From GDPR to CCPA, compliance with regulations is just the starting point. Businesses must champion ethical data use, ensuring transparency, consent, and respect for user data.
7. Scalability #
Today’s pilot project can be tomorrow’s enterprise solution. When building data products, scalability is crucial.
Whether it’s the ability to handle burgeoning data volumes, increased user interactions, or adding new features, data products should be architected with an eye on future growth and expansion.
8. Skill development #
The tools and technologies in the data realm are ever-evolving. To maintain a cutting-edge data product, the teams behind it need to be at the forefront of this evolution.
Investing in regular training sessions, workshops, and skill development ensures that the team remains adept, innovative, and ready to tackle new challenges.
9. Future-proofing #
The only constant in the data world is change. Whether it’s emerging tech trends, evolving user behaviors, or industry disruptions, data products must be built with resilience.
This involves keeping a pulse on industry trends, exploring next-gen technologies, and being ready to pivot when the landscape demands.
Building a data product management framework is a journey of strategic alignment, collaboration, and continuous refinement. These foundational pillars ensure that organizations can transform their vast data reservoirs into actionable, valuable, and innovative products. By focusing on these principles, businesses are poised to excel in the data-centric landscape of tomorrow.
Product manager vs. data product manager: 10 Differences between them #
While the core ethos of delivering value remains the same, product managers (PMs) and data product managers (DPMs) often tread distinct paths in their product journey. While both aim to deliver outstanding products to end-users, their focal points, skill sets, and approaches can differ significantly. As businesses increasingly rely on data-driven decision-making and products, understanding the nuances between a PM and a DPM becomes essential.
Let us delve deeper and provide a comprehensive table differentiating between product manager and data product manager:
Aspect | Product managers | Data product managers |
---|---|---|
Core focus | PMs typically look at the holistic product, balancing features, user experience, and market positioning. | DPMs, on the other hand, are laser-focused on data-driven products or features, ensuring each decision is backed by actionable insights. |
Technical depth | While PMs possess a broad spectrum of technical knowledge, they might not dive deep into any one area. | DPMs, however, immerse themselves in data science, understanding the intricacies of data handling, analytics, and deriving meaningful patterns from vast datasets. |
Stakeholder interaction | DPMs, however, immerse themselves in data science, understanding the intricacies of data handling, analytics, and deriving meaningful patterns from vast datasets. | DPMs frequently liaise with data scientists, engineers, and analysts, ensuring the data-driven aspects of the product align seamlessly with overarching objectives. |
User research | PMs concentrate on understanding user needs, preferences, and pain points in the broader context of the product. | DPMs delve deeper, focusing on data usage patterns and how users engage with data-centric features, ensuring data products are intuitive and value-laden. |
Product road mapping | PMs design roadmaps considering various factors like market trends, competition, and technological advancements. | DPMs base their roadmaps primarily on data potential, ensuring every feature or enhancement amplifies data-driven value. |
Ethical considerations | While PMs consider general ethical standards, specific data challenges might not be at the forefront. | DPMs grapple with nuanced challenges around data ethics, especially privacy, compliance, and the ethical use of data in decision-making. |
Solution design | PMs focus on a holistic product solution, considering various functionalities and user interactions. | DPMs often design solutions specifically tailored for data ingestion, processing, analysis, and visualization. |
Adaptability to change | PMs are agile and adapt based on market feedback, technological advancements, and competitive landscape shifts. | DPMs, due to the dynamic nature of data science, must continually adapt to new algorithms, tools, and evolving data trends. |
Risk management | PMs identify and mitigate risks across the entire product development lifecycle, considering factors like market acceptance, technical feasibility, and resource allocation. | DPMs face unique risks related to data quality, accuracy, and reliability, requiring them to employ rigorous validation and cleansing protocols. |
Education and training | PMs often have varied backgrounds, from business to design, and might not always possess formal technical training. | DPMs usually have a background in data science, statistics, or a related field, ensuring they are well-equipped to understand and navigate the complexities of data-driven products. |
The table above further distinguishes the subtle yet significant differences between product managers and data product managers. While both are integral to an organization’s product landscape, their specialized skills and focal areas define the unique value each brings to the table.
9 Data product management courses and training to elevate your skill #
In today’s rapidly evolving digital landscape, the role of data product managers (DPMs) has emerged as one of paramount significance. To navigate this complex intersection of data science and product strategy, continuous education becomes imperative. Fortunately, there’s an abundance of resources tailored specifically to those aspiring to excel in data product management.
The 9 Data product management courses and training include:
- University degree programs
- Online learning platforms
- Bootcamps
- Workshops and seminars
- Certifications
- Industry conferences
- On-the-job training
- Mentorship
- Collaborative communities
Let us look into each of the above courses and training in brief:
1. University degree programs #
Prestigious universities around the globe have recognized the niche yet expanding domain of data product management. As a result, many have curated specialized degree programs that seamlessly blend the principles of data science with product management.
These courses not only provide rigorous academic training but also facilitate real-world case studies, enabling students to grapple with the challenges they’ll face in their careers.
2. Online learning platforms #
With the advent of digital education, platforms such as coursera, udemy, and edX have democratized learning. These platforms feature a multitude of courses tailored to data product management, ranging from beginner overviews to advanced specializations.
The flexibility to learn at one’s own pace, combined with insights from global experts, makes these platforms an invaluable resource.
3. Bootcamps #
For those looking for a fast-paced, immersive learning experience, boot camps serve as an ideal choice. These short-term, intensive programs are meticulously designed to impart hands-on skills within a condensed timeframe.
Bootcamps often emphasize real-world projects, ensuring participants emerge job-ready.
4. Workshops and seminars #
Beyond structured courses, workshops and seminars provide opportunities for interactive learning. Participants benefit from engaging in discussions, hands-on exercises, and the chance to seek clarifications on pressing challenges.
Additionally, these events serve as excellent networking platforms, connecting novices with seasoned professionals.
5. Certifications #
In an ever-competitive job market, certifications can offer a distinct edge. Beyond mere credentials, certifications in data product management validate one’s skills, dedication, and commitment to the field.
Many reputable institutions and organizations offer such certifications, each varying in depth and specialization.
6. Industry conferences #
Events such as Productcon and Saastr have acknowledged the growing importance of data product management. These conferences often include dedicated sessions, tracks, or even full days focused on the nuances of DPM.
Beyond knowledge, these events are perfect for networking, discovering industry trends, and gaining insights from leading DPM practitioners.
7. On-the-job training #
Learning doesn’t stop upon landing a job. Many forward-thinking companies recognize the evolving nature of data product management and offer comprehensive on-the-job training programs.
Such initiatives enable DPMs to continually refine their skills, stay updated on industry advancements, and tackle real-time challenges effectively.
8. Mentorship #
The journey in data product management can be greatly enriched with guidance from a mentor. Experienced DPMs, having traversed the path, are invaluable sources of wisdom, advice, and motivation.
Beyond specific queries, mentors often provide broader career guidance, assisting protégés in navigating the complexities of the DPM world.
9. Collaborative communities #
Online communities, forums, and discussion groups cater to professionals in data product management. These platforms facilitate knowledge sharing, discussion on emerging trends, and collaborative problem-solving.
Being part of such a community ensures one remains connected, gains diverse perspectives, and can seek guidance when needed.
The realm of data product management is vast and ever-evolving. For those keen on carving a niche in this domain, the journey is one of continuous learning. With diverse avenues available, every aspiring or practicing DPM can find the resources that resonate with their learning style, ensuring they remain at the forefront of this exciting field.
Conclusion #
In an era where data is increasingly considered the lifeblood of modern business, understanding what data product management entails becomes paramount.
At its core, data product management is about harnessing the vast reservoirs of data to create products that not only serve functional requirements but also deliver profound value to users. It’s about merging the meticulous analysis of data science with the vision and strategy of product management to produce tools, services, or features that truly resonate with user needs.
As businesses march into the future, the role of data product management will only magnify. It will be the bridge between raw data and actionable insights, between user feedback and refined products, and between business goals and tangible outcomes.
Organizations that truly grasp and embrace the essence of data product management will be better positioned to innovate, adapt, and thrive in the evolving digital landscape.
What is data product management: Related reads #
- Data as a Product: Applying Product Thinking Into Data
- How to Build Data Products in 2023: A Step-by-Step Guide
- Modern Data Management: 8 Things You Can Gain From It
- Data Management 101: Four Things Every Human of Data Should Know
- 9 Reasons Why External Data Product is Critical
- Best Data Mesh Articles: The Ultimate Guide in 2023
- What is DataOps & How Does it Help Data Management? | Atlan
Share this article