Top 6 Data Challenges and Solutions in 2024
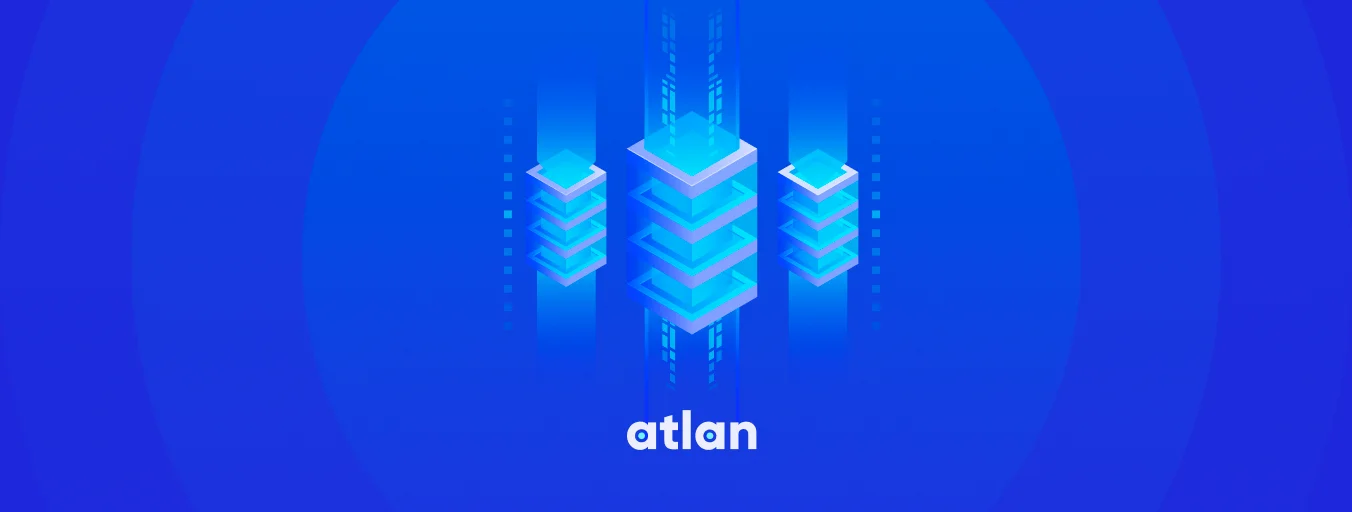
Share this article
Data challenges refer to the obstacles and complexities that organizations encounter while collecting, processing, managing, and deriving insights from data.
From privacy concerns to technological advancements, these challenges demand innovative solutions that not only ensure compliance but also unlock the full potential of data.
In this article, we will learn about the top six data challenges of 2024 and delve into actionable solutions for success in a data-driven world.
Let us dive in!
Table of contents
Data challenges: Top 6 obstacles you should be aware of
Data challenges are the intricate hurdles that organizations encounter when dealing with data throughout its lifecycle.
These challenges encompass a range of complexities, from collecting and storing data to extracting valuable insights and making informed decisions.
Let us see the common data challenges:
- Data silos and inaccessibility
- Data quality and cleansing
- Data privacy and compliance
- Data exploration and feature engineering
- Data imbalance and bias
- Predictive model deployment
Let us dive into each of the common data challenges in more detail:
1. Data silos and inaccessibility
- Data silos are caused by data stored in isolated systems or departments, that prevent a holistic view of the organization’s operations, hindering effective decision-making.
- Different teams using separate systems lead to redundant data entry, inconsistent records, and fragmented insights.
- This makes it difficult to analyze data comprehensively and understand relationships between different aspects of the business.
2. Data quality and cleansing
- Poor data quality, including duplicate, inaccurate, or incomplete information, undermines the reliability of analysis and insights.
- Data might be entered with errors, outdated, or duplicated across systems.
- Without proper validation and cleansing, decision-makers may rely on flawed data, resulting in inaccurate conclusions and misguided strategies.
3. Data privacy and compliance
- Ensuring that data handling practices align with privacy regulations (like GDPR or CCPA) to protect user information and avoid legal consequences.
- Privacy regulations demand user consent, data encryption, and secure storage.
- Failure to comply can lead to fines and loss of trust.
- Proper implementation of privacy measures requires meticulous planning and consistent monitoring.
4. Data exploration and feature engineering
- Extracting meaningful patterns and relationships from raw data, and crafting informative features for analysis.
- Data scientists need domain knowledge to identify relevant variables and relationships.
- Incomplete or irrelevant features can result in models that fail to capture the true underlying dynamics of the data.
5. Data imbalance and bias
- When certain outcomes are rare in the data (imbalanced), models can struggle to accurately predict these outcomes.
- Bias can emerge if training data isn’t representative of real-world scenarios.
- For instance, in fraud detection, legitimate transactions might vastly outnumber fraudulent ones.
- Biased data can lead to models that are overly cautious or blind to important patterns.
6. Predictive model deployment
- Implementing predictive models into existing business processes seamlessly and ensuring their ongoing maintenance.
- Integrating models requires coordination between data scientists, IT, and business units.
- Models also need continuous monitoring and updates to remain accurate and relevant as business dynamics change.
Addressing these challenges with thoughtful strategies and solutions can transform potential setbacks into avenues for growth and innovation.
Solutions to data challenges: 6 Things to know
Let us explore solutions for each of the data challenges in more detail:
1. Data silos and inaccessibility
- Implement a centralized data repository and integration tools.
- Integrate data from various sources into a centralized data warehouse or data lake.
- Use data integration tools and platforms to harmonize data from different systems.
- Create data pipelines that extract, transform, and load data from disparate sources into a unified format. This enables cross-functional analysis and a holistic view of operations.
2. Data quality and cleansing
- Perform data cleansing and establish data quality checks.
- Develop automated scripts to identify and remove duplicate records.
- Implement data validation rules to prevent incorrect data entry.
- Regularly audit data for accuracy and completeness.
- Consider using data profiling tools to assess data quality issues.
- Establish data stewardship roles to maintain data integrity.
3. Data privacy and compliance
- Implement robust data governance, encryption, and user consent mechanisms.
- Develop and enforce data governance policies that outline data handling practices.
- Implement encryption protocols to secure sensitive data both in transit and at rest.
- Create mechanisms for obtaining user consent for data collection and processing.
- Conduct privacy impact assessments to identify and mitigate risks.
4. Data exploration and feature engineering
- Collaborate with domain experts and data scientists for feature engineering.
- Engage domain experts to identify relevant features that capture meaningful relationships in the data.
- Experiment with different feature combinations to enhance model performance.
- Leverage machine learning techniques to automate feature selection and engineering.
- Continuously refine features based on feedback and changing business dynamics.
5. Data imbalance and bias
- Employ techniques like oversampling, undersampling, and fairness-aware algorithms.
- Balance the dataset by oversampling the minority class or undersampling the majority class.
- Use synthetic data generation techniques to create new instances of the minority class.
- Employ fairness-aware algorithms that mitigate bias by adjusting model predictions to ensure equitable outcomes across different groups.
6. Predictive model deployment
- Collaborate with IT teams for seamless model integration and monitoring.
- Work closely with IT departments to integrate predictive models into existing business processes.
- Develop APIs or microservices that allow applications to interact with the model in real time.
- Implement monitoring and logging mechanisms to track model performance and detect anomalies.
- Continuously update and retrain models to adapt to changing business conditions.
Addressing these data challenges requires a multi-faceted approach involving technological solutions, organizational alignment, and continuous improvement.
By implementing these solutions, businesses can overcome hurdles, unlock the potential of their data, and drive positive outcomes across various aspects of their operations.
Data challenges examples: Understanding real-world scenarios
Let us explore data challenges specific to some industries like healthcare, financial services, the insurance industry, manufacturing, banking, business, telecom industry, environmental, social, and governance (ESG):
1. Data challenges in healthcare
- Challenge: Data interoperability and integration
- Healthcare organizations often deal with diverse electronic health records (EHR) systems that don’t easily communicate with each other.
- The challenge lies in integrating data from various sources while maintaining data quality and security.
- Disparate systems can hinder a comprehensive patient view, leading to fragmented care and compromised decision-making.
2. Data challenges in financial services
- Challenge: Data privacy and security
- The financial sector handles sensitive customer financial data, making data privacy and security paramount.
- Regulatory compliance (like GDPR and CCPA) requires robust privacy practices.
- Ensuring data is encrypted, secure from breaches, and appropriately shared with user consent is critical to maintaining customer trust and avoiding regulatory fines.
3. Data challenges in insurance industry
- Challenge: Data imbalance and bias in risk assessment
- Insurers rely on historical data to assess risks and set premiums.
- If the data disproportionately represents low-risk individuals, models can underestimate risks for high-risk groups, leading to inaccurate premiums.
- Addressing data imbalance and bias is essential to ensure fair pricing and avoid regulatory scrutiny.
4. Data challenges in manufacturing
- Challenge: Predictive maintenance model deployment
- Manufacturers adopt predictive maintenance models to reduce downtime and optimize production.
- However, integrating these models into existing production systems can be complex.
- Ensuring real-time synchronization between model predictions and maintenance actions requires seamless collaboration between data scientists and operations teams.
5. Data challenges in banking
- Challenge: Customer data silos and personalization
- Banks accumulate vast customer data across multiple touchpoints like online banking, mobile apps, and in-person interactions.
- Integrating this data to create a unified customer profile is a challenge.
- Without a comprehensive view, banks struggle to offer personalized services and experiences that meet customer expectations.
6. Data challenges in telecom industry
- Challenge: Data volume and scalability
- Telecom companies manage massive amounts of data from network logs, customer interactions, and billing records.
- As the volume of data increases with expanding services, maintaining efficient data processing, storage, and analysis becomes challenging.
- Scalable data infrastructure is essential to avoid performance bottlenecks.
7. Data challenges in environmental, social, and governance (ESG)
- Challenge: Data quality and standardization
- ESG initiatives require accurate and standardized data for measuring environmental impact, social responsibility, and corporate governance.
- Aggregating data from diverse sources and ensuring its accuracy and consistency is vital to accurately assess a company’s ESG performance and make informed investment decisions.
Each industry faces its unique data challenges, which can significantly impact decision-making, operations, and customer experiences.
Addressing these challenges involves a combination of technological solutions, industry expertise, and a commitment to data-driven excellence.
Summarizing it all together
Data challenges can encompass issues related to data quality, security, privacy, integration, and more, hindering effective decision-making and efficient operations.
Addressing data challenges requires strategic planning, technological solutions, and cross-functional collaboration to unlock the value of data and drive positive outcomes.
The top data challenges of this year underscore the increasing importance of data quality, privacy, and agility. By embracing solutions that prioritize secure data practices, robust infrastructure, and adaptable strategies, businesses can overcome these challenges and transform them into opportunities.
Navigating these complexities with foresight and innovation ensures that data remains not just a challenge, but a catalyst for growth and resilience in an ever-changing digital age.
Data challenges: Related reads
- What are Data Silos and How Can You Break Them Down?
- Speed Bump! 10 Data Governance Challenges to Overcome
- 10 Data Catalog Challenges and a Step-by-step Action Plan to Tackle Them
- 8 Ways to Tackle Data Governance Adoption Challenges
- Data Cataloging Process: Challenges, Steps, and Success Factors
- What Is Dark Data and How Can You Make Sense of It?
Share this article