Data Lineage vs Data Traceability: 7 Crucial Distinctions
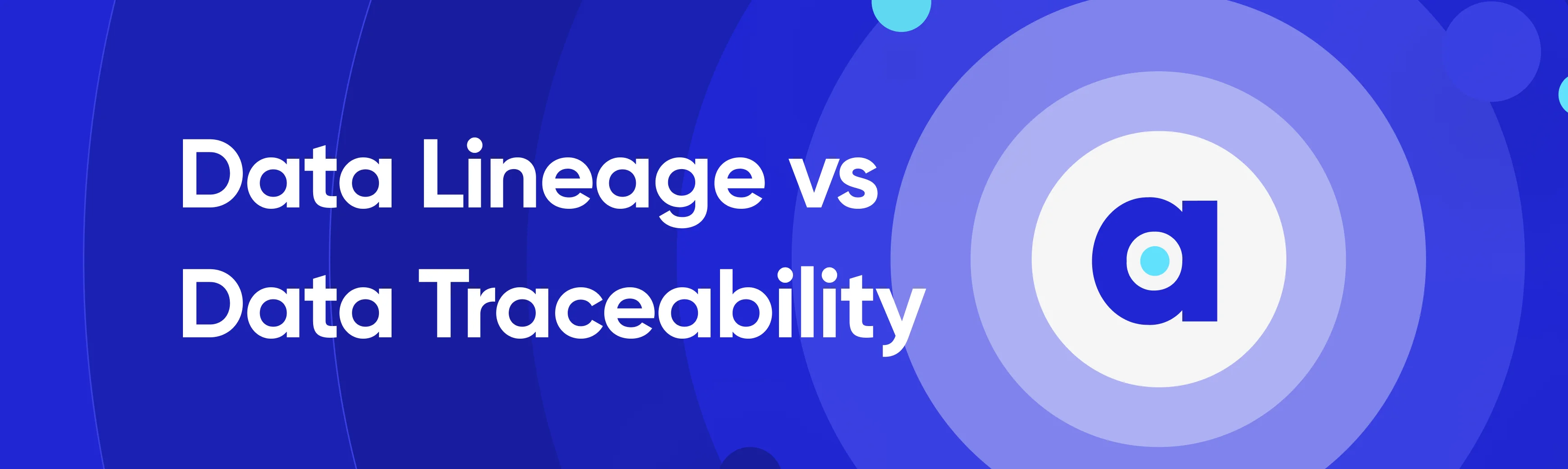
Share this article
Data lineage reveals where data has come from and how it has evolved through its lifecycle. On the other hand, data traceability is more focused on the ability to verify the accuracy, quality, and reliability of data through an audit trail of the data and metadata.
Modern data problems require modern solutions - Try Atlan, the data catalog of choice for forward-looking data teams! 👉 Book your demo today
Both are critical components of modern data management and governance strategies. In this article, we will explore:
- What are the differences between data lineage and data traceability?
- Why are data lineage and data traceability important?
- Books on data lineage and traceability
Ready? Let’s dive in!
Table of contents #
- Understanding data lineage vs. traceability: Key factors to know
- Data lineage vs data traceability: Key concepts and significance
- Data lineage vs. data traceability: A tabular view
- Books on data lineage and traceability
- Summary
- Data lineage vs data traceability: Related reads
Understanding data lineage vs data traceability: Key factors to know #
To fully appreciate the differences and relationship between data lineage and data traceability, let us understand these concepts in the context of a few additional factors:
- Data governance
- Data quality
- Data security
- Business intelligence and analytics
- Metadata management
- Regulatory compliance
- Data operations (DataOps)
Let’s look into these factors in brief:
1. Data governance #
Both data lineage and traceability are critical components of data governance, the overall management of data availability, usability, integrity, and security in an organization.
They contribute to maintaining high data quality, ensuring regulatory compliance, and making informed business decisions.
2. Data quality #
Understanding data lineage helps identify points in the data journey where errors or inconsistencies might be introduced, while data traceability verifies the correctness and integrity of data.
Together, they provide a robust mechanism to maintain and improve data quality.
3. Data security #
Traceability can aid in tracking unauthorized access or changes to data, which is important for data security.
Lineage can show where sensitive data is going and who or what processes are accessing it, which is crucial for privacy regulations like GDPR or CCPA.
4. Business intelligence and analytics #
Lineage can help understand how data is transformed before it ends up in a report or a dashboard, thus ensuring the correctness of insights.
Traceability ensures that the data used in these insights have been processed correctly and is reliable.
5. Metadata management #
Metadata provides information about other data, and managing it is integral to both data lineage and traceability.
Metadata might include information about data’s source, who has accessed it, how it has been transformed, etc.
6. Regulatory compliance #
Many industries are governed by regulations that require clear documentation of data lineage and traceability.
For instance, financial services firms must comply with BCBS 239, which stipulates risk data aggregation capabilities and risk reporting practices, needing clear data lineage.
7. Data operations (DataOps) #
Both data lineage and traceability are essential for smooth DataOps, enhancing the speed, quality, and value of data analytics.
Understanding these factors can help you appreciate the importance of data lineage and traceability in data management and governance, and how they work together to support data integrity, security, and compliance within an organization.
Data lineage vs. data traceability: Key concepts and significance #
Let us look at a brief explanation of data lineage and data traceability.
What is data lineage? #
Data lineage refers to the lifecycle of data, including its origins, where it moves over time, and what happens to it. It’s about understanding the journey that data has taken from its source to its current state.
This can include transformations, processes, systems, and people that have interacted with the data. Data lineage is often visualized in a lineage diagram, which provides a clear view of the data’s history and context.
It is particularly important in:
- Troubleshooting (finding the root cause of data quality issues)
- Impact analysis (understanding the potential impact of changes in data), and
- Meeting regulatory compliance requirements.
Why is data lineage important? #
Data lineage is a critical concept in data management and analytics that refers to the tracking and visualization of the flow and transformation of data as it moves through various stages of its lifecycle within an organization. It is important for several reasons:
- Data quality assurance
- Compliance and regulatory requirements
- Data governance
- Data exploration and discovery
- Impact analysis
- Resource optimization
Let’s look at them in detail:
1. Data quality assurance #
Data lineage helps ensure data quality by providing a clear understanding of how data is sourced, transformed, and used.
When issues with data quality arise, lineage information can be used to trace back to the source of the problem, facilitating faster identification and resolution of data quality issues.
2. Compliance and regulatory requirements #
Many industries are subject to regulatory requirements and compliance standards that demand data traceability and auditing.
Data lineage provides a documented record of data transformations and usage, helping organizations demonstrate compliance with data-related regulations such as GDPR, HIPAA, or SOX.
3. Data governance #
Data governance involves establishing policies, standards, and processes for managing data across an organization.
Data lineage is a fundamental component of data governance as it enables organizations to understand how data is handled and ensures that data is used in accordance with established governance policies.
4. Data exploration and discovery #
Data scientists, analysts, and business users often need to explore and understand data to derive insights or make informed decisions.
Data lineage helps them trace the origins of datasets, understand the transformations applied, and assess the suitability of data for specific analysis tasks.
5. Impact analysis #
When changes are proposed to data sources or data transformation processes, it is essential to understand the potential impact of those changes on downstream systems and reports.
Data lineage allows organizations to perform impact analysis, helping them assess the consequences of changes before they are implemented.
6. Resource optimization #
Data lineage can uncover redundancies and inefficiencies in data processes.
By visualizing how data flows and where transformations occur, organizations can identify opportunities to optimize data pipelines and reduce resource consumption.
In summary, data lineage is important because it enhances data governance, quality, compliance, and decision-making processes while also facilitating collaboration, optimizing resources, and mitigating risks. It empowers organizations to manage their data effectively and make informed data-related decisions, ultimately driving better business outcomes.
What is data traceability? #
Data traceability, on the other hand, is more focused on the ability to verify the accuracy, quality, and reliability of data through an audit trail of the data and metadata.
Traceability allows organizations to follow the data and transformations back to its origin, verifying the authenticity and integrity of the data.
It’s more about proving that the data has been processed correctly and hasn’t been tampered with.
It’s crucial for regulatory compliance, especially in industries where data accuracy is paramount, like healthcare or finance.
Why is data traceability important? #
Let us look into each of the above reasons in detail:
- Transparency and accountability
- Compliance and governance
- Data integrity and quality
- Operational efficiency
- Risk management
- Enhanced customer trust
Let’s look at them in detail:
1. Transparency and accountability #
Data traceability can provide a complete record of who has accessed data, when, and why. This can help ensure accountability for data handling and usage, and aid in detecting any unauthorized access or suspicious activity.
2. Compliance and governance #
Data traceability supports compliance with various data protection and privacy regulations, such as GDPR. By maintaining a record of data usage and processing activities, organizations can demonstrate that they handle data in line with regulatory requirements.
This can also facilitate data governance by helping organizations manage and control how data is used and ensure it is used ethically and responsibly.
3. Data integrity and quality #
Traceability can help organizations maintain and improve data quality and integrity. By understanding where data has come from, how it has been used, and how it has been transformed, organizations can detect and address issues that might affect data quality.
This helps ensure that the data can be trusted and that decisions based on the data are sound.
4. Operational efficiency #
In complex data environments, understanding data’s journey from source to usage can facilitate operational efficiency.
If a problem arises, traceability allows for quicker identification of the source of the issue, enabling a faster resolution.
5. Risk management #
Data traceability can also help manage risks related to data handling and usage.
For instance, it can help identify potential privacy risks (like unauthorized access or usage), assess their impact, and guide mitigation efforts.
6. Enhanced customer trust #
By demonstrating robust data traceability, organizations can build customer trust. Customers can feel assured that their data is handled responsibly, enhancing their trust in the organization’s products or services.
While they seem similar, the main difference between the two lies in their purpose. Data lineage is about understanding the journey and transformation of data, while data traceability is about auditing and validating the authenticity and integrity of the data.
As a data platform architect, both data lineage and data traceability will be important for your work.
To ensure data quality and integrity, you’ll want to create a system that allows you to track the journey of data (lineage) and also verify its authenticity and correctness (traceability).
Data lineage vs. data traceability: A tabular view #
Here’s a tabular representation of the differences and relationships between data lineage and data traceability:
Aspect | Data lineage | Data traceability |
---|---|---|
Definition | The life-cycle of data including its origins, where it moves, and what happens to it. | The ability to verify the accuracy, quality, and reliability of data through an audit trail. |
Purpose | To understand the journey and transformation of data. | To audit and validate the authenticity and integrity of the data. |
Focus | Data's journey through systems, transformations, and its interactions. | Audit trail of data and metadata, confirming data's authenticity and integrity. |
Importance | Troubleshooting (finding the root cause of data issues), impact analysis, and regulatory compliance. | Regulatory compliance, and validating data accuracy and integrity. |
Visualization | Often visualized in a lineage diagram showing data's history and context. | Often represented as an audit trail documenting data's processing and integrity checks. |
Relationship to the other | Both data lineage and data traceability are closely linked. Detailed data lineage can contribute to effective data traceability, as it shows the path data takes through the system. | Data traceability can reinforce data lineage by adding a layer of validation and authenticity checking to the data's journey. |
Remember that both data lineage and data traceability are important parts of a comprehensive data governance strategy. They complement each other in maintaining and ensuring the quality and integrity of data within an organization.
Books on data lineage and traceability #
Understanding data lineage and data traceability is a key part of data management and governance, and while there might not be books dedicated solely to these two topics, they are usually covered in books on data management, data governance, and data architecture.
Here are some recommended books where these topics may be explored:
-
Data Governance: How to Design, Deploy and Sustain an Effective Data Governance Program by John Ladley
This book provides a comprehensive overview of data governance, and it includes discussions about data lineage and traceability within the context of broader governance strategies.
-
Enterprise Data Governance: Reference and Master Data Management Semantic Modeling by Pierre Bonnet
While this book specifically addresses semantic modeling, it offers insights into data governance principles, including data lineage and traceability.
-
The Data Warehouse Toolkit: The Definitive Guide to Dimensional Modeling by Ralph Kimball and Margy Ross
This book is considered a classic in the field of data warehousing. While it doesn’t focus directly on lineage and traceability, understanding dimensional modeling will contribute to a greater understanding of these concepts as they are integral to building and maintaining a data warehouse.
-
Data Governance: The Definitive Guide: People, Process, and Tools to Operationalize Data Trustworthiness by Evren Eryurek, Uri Gilad, Valliappa Lakshmanan, Anita Kibunguchy-Grant, and Jessi Ashdown
This guide offers a comprehensive overview of data governance, including the understanding of data lineage and traceability.
-
Non-Invasive Data Governance: The Path of Least Resistance and Greatest Success by Robert S. Seiner
This book discusses a non-invasive approach to implementing data governance, where concepts of data lineage and traceability play significant roles.
Summary #
In conclusion, data lineage and data traceability are key components of modern data management and governance strategies. They help organizations understand where their data comes from and how it’s used, and they can be used to improve data quality, ensure regulatory compliance, and make informed business decisions.
In short, data lineage and data traceability are essential for maintaining and ensuring the quality and integrity of data within an organization. By understanding the difference between these two concepts and implementing a solution that meets your organization’s needs, you can reap the many benefits that they have to offer.
Data lineage vs data traceability: Related reads #
- What is Data Lineage & Why is it important?
- Data Lineage 101: Importance, Use Cases, and their role in governance
- 5 Types of Data Lineage: Understand all ways to view your data
- 6 Benefits of Data Lineage with insights into how businesses are leveraging it
- Automated Data Lineage: Making lineage work for everyone
- Open Source Data Lineage Tools: 5 popular to consider in 2023
- Amundsen Data Lineage Setup with dbt
- Data lineage for Snowflake and BigQuery
- Data Catalog vs. Data Lineage: Differences, use cases, and evolution of available solutions
Share this article