Data Warehouse Governance: Building a Strong Foundation for Quality, Security, and Compliance
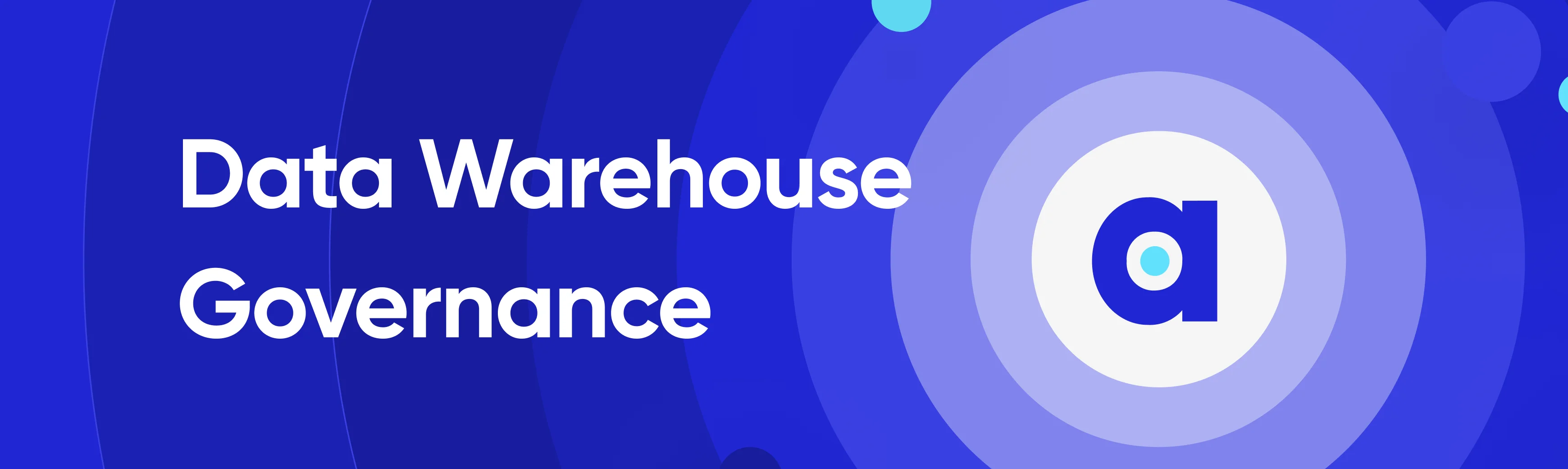
Share this article
Data warehouse governance is an essential part of maintaining data quality, security, accessibility, and regulatory compliance. It is an overarching system of practices, protocols, and technologies used to manage and ensure the effective use of data within an organization.
In this blog, we will learn why is data warehouse governance critical in ensuring data quality, security, accessibility, and regulatory compliance for your organization.
Let’s dive in!
Table of contents #
- Building a strong foundation: Key components of data warehouse governance
- What is data governance in data warehouse: Explained with examples
- How to evaluate the best data governance tool for your data warehouse integration?
- Data warehouse governance best practices
- Summary
- Related reads
Building a strong foundation: Key components of data warehouse governance #
Now that we have introduced the concept of data warehouse governance at a basic level, let us understand its key elements, which are:
- Data quality management
- Data security and privacy
- Data access and usage
- Compliance and regulation
- Metadata management
- Data lifecycle management
- Data architecture
- Change management
Let us look into each of the above key elements in brief:
1. Data quality management #
- Data quality management involves ensuring that data in the warehouse is accurate, reliable, and consistent. This includes procedures for data cleaning, de-duplication, and validation.
- It may also involve setting standards for data entry and regular audits to ensure these standards are being met.
2. Data security and privacy #
- Protecting sensitive data is a vital aspect of governance. This can involve physical security measures, like secure data centers, as well as digital measures like encryption, access control, and user authentication.
- Privacy concerns can also be addressed through de-identification or anonymization of sensitive data.
3. Data access and usage #
- Good data governance also involves controlling who has access to what data, and how they’re allowed to use it. This can be done through user permissions, roles, and access control lists (ACLs).
- It also means ensuring that users are trained in appropriate data use and ethics.
4. Compliance and regulation #
- This involves keeping the data warehouse compliant with applicable laws and regulations, such as GDPR in Europe or HIPAA in the US.
- This includes provisions for data retention, data deletion, and the rights of data subjects.
5. Metadata management #
- Metadata includes information like the data’s source, when it was last updated, who can access it, etc.
- Effective metadata management makes it easier to find, use, and manage data in the warehouse.
6. Data lifecycle management #
- This involves tracking data from its initial creation or acquisition, through its active use, to its eventual archiving or deletion.
- This includes managing data backups and ensuring data is not lost or corrupted.
7. Data architecture #
- It refers to the design of your data warehouse to meet current and future needs.
- This includes considering how to structure your data for efficient querying and analysis, and how to scale your architecture as your data volume grows.
8. Change management #
- As your data needs evolve, you’ll need to make changes to your data warehouse.
- Good governance involves having a system for managing these changes to minimize disruption and maintain data quality and security.
To effectively implement data warehouse governance, it’s crucial to have a dedicated team or individual responsible for overseeing the process. This could be a data steward, a data governance committee, or a chief data officer, depending on the size and complexity of your organization.
Additionally, it’s important to communicate the importance of data governance to all users of the data warehouse and provide them with the training they need to comply with governance policies and procedures.
What is data governance in data warehouse: Explained with examples #
Data governance in the context of a data warehouse refers to the overarching framework for managing, improving, monitoring, and ensuring the quality, security, and availability of data stored in the data warehouse. Data governance helps ensure that data assets are formally managed throughout the enterprise, making it easier to share, understand, and protect information.
To illustrate that, let’s look at a few scenarios that show how data warehouse governance might work in reality:
- Data quality management
- Data security and privacy
- Compliance and regulation
- Metadata management
- Data lifecycle management
- Change Management
Let us look into each of the above scenarios in detail:
Scenario #1 - Data Quality Management #
- An e-commerce company’s data warehouse collects information from multiple sources, including customer transactions, website analytics, and third-party market research.
- The data governance team has implemented rules to ensure data quality, such as checking for duplicates and validating entries against known patterns. For example, ensuring email addresses are in the right format.
- They also routinely monitor data quality metrics and conduct periodic audits.
- When data quality issues are identified, they’re logged, investigated, and resolved according to established procedures.
Scenario #2 - Data security and privacy #
- A healthcare organization holds highly sensitive patient data in its data warehouse.
- Governance policies dictate strict role-based access control, meaning each user has access only to the data necessary for their role.
- All data is encrypted, both in transit and at rest.
- Furthermore, the data is anonymized to protect patient privacy. Regular security audits ensure these measures are effective and up to date.
Scenario #3 - Compliance and regulation #
- A multinational financial firm must comply with various regulations, including GDPR and Sarbanes-Oxley.
- They’ve designed their data warehouse governance to incorporate these rules.
- This includes features like data retention schedules, which ensure:
- Data is not kept longer than legally allowed, and
- Mechanisms for honoring data subject rights, such as data deletion or portability requests.
4. Scenario - Metadata management #
- A media streaming company uses metadata extensively to recommend content to its users.
- They have robust metadata management procedures in their data governance policy.
- This includes standards for how metadata should be collected and formatted, and procedures for updating and maintaining it.
- As a result, they can easily track the source, quality, and usability of their data.
5. Scenario - Data lifecycle management #
- An insurance company’s data warehouse holds years of claim data.
- Their data governance includes policies for how long different types of data should be retained, and when and how data should be archived or deleted.
- This includes making regular backups to prevent data loss, and running regular checks to identify and resolve data corruption.
6. Scenario - Change management #
- A rapidly growing tech startup is constantly adding new features, which often require changes to their data warehouse.
- Their governance policy includes a clear process for managing these changes.
- This involves:
- Identifying the need for change
- Evaluating potential impacts
- Testing the change in a controlled environment, and
- Finally, implementing it in the live warehouse, all while communicating clearly with all relevant stakeholders.
In each of these scenarios, the key to effective data warehouse governance is a comprehensive, well-documented set of policies and procedures, combined with regular monitoring and auditing to ensure compliance.
Data governance in a data warehouse is often part of a broader data governance strategy within an organization. It helps to maximize the value of data, minimize risks, and enable better decision-making.
How to evaluate the best data governance tool for your data warehouse integration? #
We know that data governance is evolving. It’s not just about setting and enforcing rules anymore; it’s also about fostering collaboration, facilitating understanding, and enabling teams to work better together.
To do this effectively, you’ll need a data governance tool that aligns with these goals. Here’s a framework to think about evaluating such a tool that integrates with your data warehouse:
- Integration and compatibility
- Metadata management
- Data quality management
- Security and compliance
- Collaboration and accessibility
- Data cataloging
- Automation and scalability
- Change management
- Data lineage
- Flexibility and customizability
Now, let us look at each of the above concepts in brief:
1. Integration and compatibility #
Does the tool integrate seamlessly with your data warehouse? Is it compatible with your existing tech stack? It should be able to handle data from various sources and work with the tools you use for data processing, analysis, visualization, etc.
2. Metadata management #
The tool should be capable of handling metadata effectively. This includes not just technical metadata, but also business metadata and operational metadata.
3. Data quality management #
Can the tool identify and help resolve data quality issues? It should be able to set and enforce data quality rules and highlight issues for investigation.
4. Security and compliance #
Does the tool support data privacy and compliance needs? This includes features like role-based access control, encryption, anonymization, data masking, and audit trails.
5. Collaboration and accessibility #
Can it facilitate collaboration between different roles in your organization? This might include shared data dictionaries, discussion threads, and tools for documenting and sharing knowledge.
6. Data cataloging #
The tool should have robust data cataloging capabilities. This helps users discover available data assets, understand their purpose and quality, and find out how to access them.
7. Automation and scalability #
It’s important that your governance tool can automate repetitive tasks and scale as your data and organization grow.
8. Change management #
The tool should have robust change management capabilities, allowing changes to be tracked, tested, and implemented without disrupting ongoing work.
9. Data lineage #
The tool should provide clear data lineage, making it easy to track the journey of a data element from its source through its various transformations.
10. Flexibility and customizability #
Every organization’s data needs are unique. A good data governance tool should offer flexibility and be customizable to adapt to your specific governance policies and workflows.
By evaluating potential data governance tools against these criteria, you can find one that will support not just the rules and restrictions of data governance, but also the collaboration and understanding that truly make data governance valuable.
9 Data warehouse governance best practices for your data assets: A blueprint for success #
So far, we have understood the need and criticality of data warehouse governance. Now, let us look at a blueprint for implementing data governance for your data warehouse assets:
- Decentralize data governance
- Implement a data catalog
- Define clear roles and responsibilities
- Establish data quality standards
- Automate governance processes
- Set up data access controls
- Ensure compliance with regulations
- Promote collaboration and communication
- Monitor and improve
Now, let us look into each of the above aspects in brief:
1. Decentralize data governance #
- Rather than having a top-down approach, enable your data practitioners (analysts, engineers, scientists) to be a part of the data governance process.
- They should have the ability to help define and enforce data governance policies.
2. Implement a data catalog #
- A data catalog is essential to track all the data assets in your data warehouse.
- This catalog should include metadata about each data asset, such as its source, quality, usage, and any transformations it has undergone.
- The catalog should be easily accessible and searchable by all users.
3. Define clear roles and responsibilities #
- While data governance should be decentralized, there should still be clear roles and responsibilities for various aspects of governance.
- This includes who is responsible for ensuring data quality, who can grant or revoke access to certain data assets, and who can make decisions about how data is categorized or labeled.
4. Establish data quality standards #
- Quality standards need to be set and enforced across your data assets.
- This involves both setting rules for what constitutes ‘good’ data, and implementing processes to check and maintain data quality over time.
5. Automate governance processes #
- Wherever possible, automate your data governance processes.
- This includes tasks like updating the data catalog, checking data quality, and enforcing access controls.
- Automation will make these tasks more efficient and less prone to human error.
6. Set up data access controls #
- Data security is a key part of data governance. You should have clear policies about who can access different data assets, and enforce these policies using role-based access controls.
- These controls should be flexible enough to cater to the needs of different teams and projects.
7. Ensure compliance with regulations #
- If you’re subject to any data protection regulations, make sure your data governance policies and processes are compliant.
- This might involve steps like anonymizing personal data, setting up audit trails, or implementing data retention policies.
8. Promote collaboration and communication #
- Create platforms or channels where data practitioners can share knowledge, ask questions, and collaborate on data projects.
- This can help to build a community around your data and foster a culture of data stewardship.
9. Monitor and improve #
- Lastly, keep monitoring your data governance practices and looking for ways to improve. Use feedback from your data practitioners to identify pain points or areas for improvement.
- Always be ready to evolve your data governance practices to suit the changing needs of your organization and the wider regulatory landscape.
Remember, the key to modern data governance is agility and collaboration. Your data governance framework should enable your data practitioners to work better together, rather than just imposing restrictions on them.
Summary #
In summary, effective data warehouse governance is crucial for managing the increasing volumes of data and harnessing the value of that data. However, it needs to be approached in a way that enables collaboration and agility, rather than just imposing control.
Implementing a successful data governance strategy involves finding the right balance between control, flexibility, and collaboration, and should always be geared towards empowering your data practitioners and improving your data practices.
Data warehouse governance: Related reads #
- What is Data Governance? Its Importance, Principles & How to Get Started?
- Key Objectives of Data Governance: How Should You Think About Them?
- Data Governance Framework Examples, Templates, Standards, Best Practices & How to Create One?
- Data Governance and Compliance: Act of Checks & Balances
- How to implement data governance? Steps, Prerequisites, Essential Factors & Business Case
- How to Improve Data Governance? Steps, Tips & Template
- 7 Steps to Simplify Data Governance for Your Entire Organization
- Snowflake Data Governance Features, Frameworks & Best Practices
- Automated Data Governance: How Does It Help You Manage Access, Security & More at Scale?
- Enterprise Data Governance Basics, Strategy, Key Challenges, Benefits & Best Practices
- Data Governance in Manufacturing: Steps, Challenges, and Practical Examples
- Data Governance in Retail: Best Practices, Challenges, and Viable Solutions
- Data Governance in Insurance: Why is it Important and How it Drives Positive Business Outcomes
Share this article