Data Governance vs Data Observability: An Overview, 7 Key Differences, and Case Studies
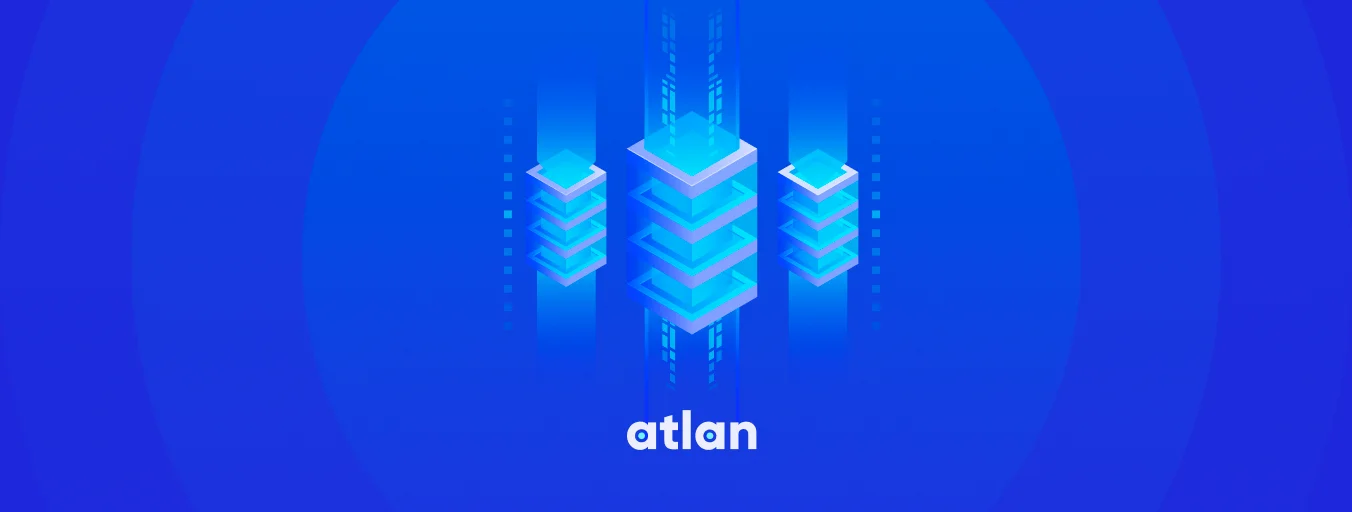
Share this article
The main difference between data governance and data observability is that data governance is an organization-wide framework for managing, organizing, and using data effectively.
Meanwhile, data observability is the real-time monitoring of your data estate to proactively detect and address data quality issues and anomalies.
This article looks into the fundamentals of data governance and data observability to explain the differences and explore their roles in the landscape of data management and the modern data ecosystem.
Want to make data governance a business priority? We can help you craft a plan that’s too good to ignore! 👉 Talk to us
Table of Contents #
- Data governance vs data observability: Concepts to understand
- Data governance vs. observability: What’s more important?
- Data governance vs observability: 7 key differences
- How does observability help with data governance
- Summing it all up
- Related reads
Data governance vs data observability: Concepts to understand #
Let’s begin by reviewing the fundamentals, starting with data governance.
Data governance: An overview #
Data governance is an organization-wide framework to ensure the accuracy, consistency, availability, security, and integrity of your data assets. This promotes responsible use and accountability of your data.
Read more → Getting started with active data governance
The core components of data governance include (but aren’t limited to):
- Data governance framework
- Roles and responsibilities
- Policies and procedures
- Data cataloging and metadata management
- Data quality, security, and privacy
- Data integration and interoperability
- Data stewardship
Read more → Data governance key components 101
Data observability: An overview #
Data observability is a holistic practice — a collection of activities and technologies — for the ongoing management of your data quality, health, and usability.
The four pillars of data observability are metrics, logs, metadata, and lineage.
The core data observability activities include:
- Data monitoring
- Data alerting
- Data tracking
- Data comparisons
- Data logging
Read more → Data observability 101
Data governance vs. observability: What’s more important? #
Data observability can enhance the effectiveness of your data governance program by ensuring that data quality is high.
That’s because proper observability can help you create a data ecosystem that prevents issues and ensures the integrity of increasingly complex data pipelines over time. This is crucial for better data quality and collaboration. Better data quality influences the effectiveness of your decisions.
As mentioned earlier, data governance is a framework for responsible use of data. The goal is to create more business value with data, while complying with external compliance regulations.
So, data observability can be seen as one of the factors or tactical practices for effectively implementing a data governance program.
Data teams can use data observability to understand how data flows and is used within their organizations, and get better at detecting data misuse, malfeasance, or potential regulatory non-compliance.
The best way forward is to integrate observability into your overall data governance and management strategy.
Also read → Data quality in data governance
Data governance vs observability: 7 key differences #
In this segment, we provide a comprehensive comparison of data governance and data observability in an easy-to-read table.
Data Governance | Data Observability | |
---|---|---|
Definition | An internal strategy for data collection, storage, processing, management, and use | Ongoing management of data health and usability that offers real-time insights into data behavior and health |
Goal | Manage, maintain, and use data to create business value, while aligning with business goals and regulations. | Proactively detect anomalies and deviations |
Components | Policies and procedures, data security and privacy, metadata management, etc. | Metrics, logs, metadata, and lineage |
Activities | Establishing data policies, classification rules, access controls, data integration and interoperability infrastructure, etc. | Managing data health indicators, anomalies, real-time monitoring, logging, automated alerts, etc. |
Benefits | Improved data quality, compliance with regulations, minimized data risks, and better decisions. | Early issue detection to maintain data health and reduce data downtime as well as operational disruptions. |
Stakeholders involved | Data stewards, data owners, compliance officers, and data management teams | Data reliability engineers, DataOps teams |
Also, read → How to select the best data governance tool
How does observability help with data governance: Let’s hear from NASDAQ and Sands Capital #
The background and challenges with data quality #
For NASDAQ, reactive data monitoring was an issue. According to Michael Weiss, Senior Director of Product Management (NAM, Data Access and Analytics) at NASDAQ:
“While a pipeline might succeed, we wouldn’t know if the data was right, wrong, or indifferent.”
Meanwhile, Sands Capital wanted to tackle the issue of data quality and visibility with limited time and resources. Here’s how Adam Rosenbaum, Data Governance Analyst at Sands Capital, puts it:
“You’re going to be hard-pressed to get a Data Engineer to say, ‘Sure, here’s the username and password, take a look, take a tour.’ To give that visibility and observability to what’s in there, to actually see a column and the first 20 rows? It’s truly helpful.”
Also, read → Building a data community at Sands Capital
For both organizations, better data quality translated to better data governance, and that’s why data observability became crucial.
Solution: Data quality and governance with Atlan and Monte Carlo #
NASDAQ added Monte Carlo and Atlan to their data stack. According to Michael, this setup helped in catching data incidents early on, providing everyone with clear visibility into the current status of data accuracy.
This also helped NASDAQ automate data quality checks and testing, which supports their governance efforts.
“We can automate data quality standards while maintaining visibility into how each team follows global policies and sets local policies within the domain.” Michael Weiss, Senior Director of Product Management at NASDAQ
NASDAQ’s data teams have better visibility into data health. As a result, they can fix bugs faster and communicate about potential downstream impacts right away.
Meanwhile, Sands Capital adopted Atlan to map their data estate, improve data discovery, bring context to their data assets, and improve collaboration.
“[Previously], questions about data or broken dashboards once meant numerous messages to Data Engineering and Data Governance, and a lengthy back-and-forth and investigation. [Now, we use] Atlan to proactively communicate breakages using alerts on data assets, and messages on Slack.“ Adam Rosenbaum, Data Governance Analyst at Sands Capital
According to Akriti Shrestha, Business and Data Associate at Sands Capital, Atlan has brought a lot of light into the data. Data teams have complete visibility into issues and the fixes being implemented in real-time.
“If someone’s working on the bug, you can see it’s in progress. If it’s resolved, the communication is so fluent. That’s been amazing.” Akriti Shrestha, Business and Data Associate at Sands Capital
In both cases, data observability helped “shine the light into data”. Using Atlan helped enable automation and self-service. It also promoted better data discovery and understanding as well as collaboration. All of these factors contributed to the effectiveness of their data governance efforts.
Read more → Scaling data trust and collaboration with Monte Carlo and Atlan
Summing it all up #
Data governance is a program to ensure the accuracy, credibility, integrity, privacy, and security of your data assets. It tells you what data you have, where that data is stored, and how can you use it. The goal is to help you arrive at better business insights using your data.
Meanwhile, data observability is one of the means to ensure better data governance. It focuses on real-time data health monitoring and detecting pipeline issues proactively. Data operations become transparent and data asset discovery and exploration becomes easier. This translates to better data quality, thereby increasing your trust in it.
The NASDAQ and Sands Capital case studies establish this symbiotic relationship between data governance and data observability. Setting up a data ecosystem with adequate data observability will certainly support your data governance initiatives.
Data governance vs observability: Related reads #
- What is Data Governance? Its Importance & Principles
- Data Governance Roles and Their Responsibilities
- Data Governance and Compliance: Act of Checks & Balances
- How to implement data governance? Steps, Prerequisites, Essential Factors & Business Case
- Automated Data Governance: How Does It Help You Manage Access, Security & More at Scale?
- Data Observability 101: Everything You Need to Know
- How Data Observability & Data Catalog Are Better Together
- Data Observability vs Data Testing: 6 Points to Differentiate
- Data Lineage & Data Observability: Why Are They Important?
- Data Observability & Data Mesh: How Are They Related?
- Data Observability for Data Engineers: What, Why & How?
- Data Governance vs Data Security: 7 Key Differences in 2024
- Data Governance vs Data Analytics: Which is the best for you?
- Data Quality vs Data Governance: Learn the Differences & Relationships
Share this article