Self-Service Data: Definition, Benefits, and Step-by-Step Guide
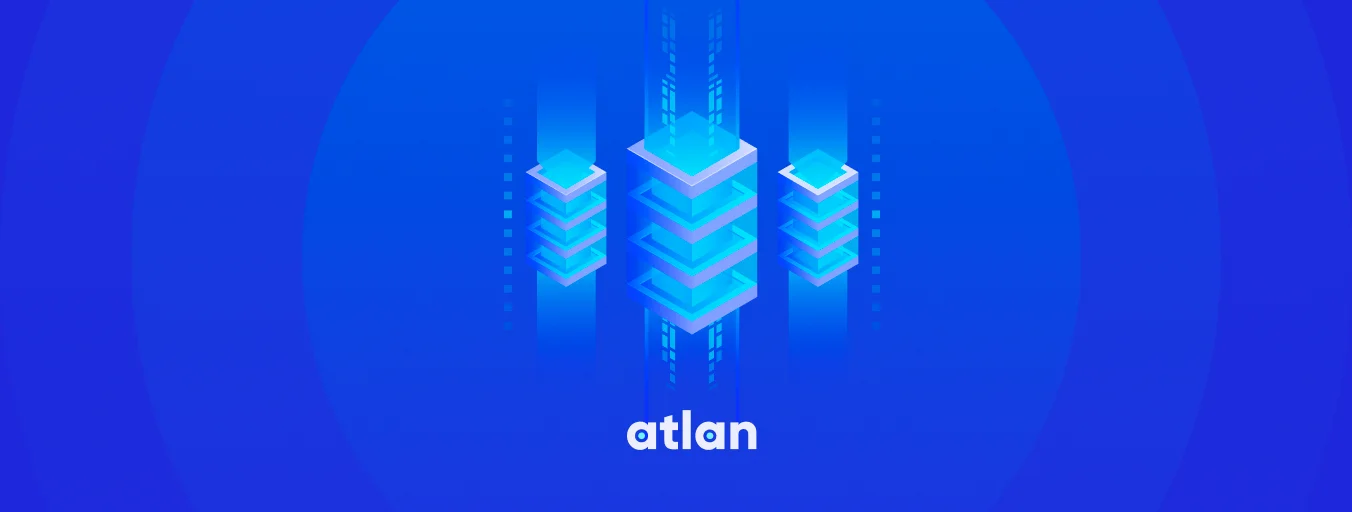
Share this article
Traditionally, when employees from various departments need data insights, they have to request these from the IT or specialized data teams. This process can be time-consuming and inefficient, leading to delays in decision-making and a lack of agility in responding to market changes.
According to Gartner Self-Service Analytics is a form of business intelligence (BI) in which line-of-business professionals are enabled and encouraged to perform queries and generate reports on their own, with nominal IT support.
The era of self-service data has arrived, promising to streamline processes, enhance decision-making, and foster a data-driven culture within organizations.
Modern data problems require modern solutions - Try Atlan, the data catalog of choice for forward-looking data teams! 👉 Book your demo today
With this article, we will understand:
- What self-service data is
- The importance of the same
- The concept of self-service data management
- Look at various self-service data tools
So, let’s dive in!
Table of contents #
- What is self-service data?
- What is self-service data preparation?
- 10 Steps roadmap for self-service data
- Importance of self-service data
- What is self-service data management?
- What are self-service data tools?
- Summing up
- Related reads
What is self-service data? What are its key characteristics? #
Self-service data refers to a set of processes, tools, and technologies that enable individuals or business users to access, analyze, and manipulate data without requiring the direct intervention of data specialists.
The goal of self-service data is to empower non-technical users to work with data and gain insights independently, reducing the dependence on data professionals and expediting decision-making processes. Key characteristics of self-service data include:
- Data accessibility
- User-friendly tools
- Data governance
- Data preparation
- Visualization
- Collaboration
- Automation
- Scalability
Now, let’s delve deeper into each of these aspects:
1. Data accessibility #
Self-service data solutions prioritize making data easily accessible to users. This means integrating data from various sources such as databases, spreadsheets, cloud services, and more.
Accessibility ensures that users can obtain the data they need for analysis without technical barriers.
2. User-friendly tools #
The tools provided in self-service data platforms are designed with a focus on user experience. They are intuitive, with interfaces that allow users to perform tasks like querying, filtering, and transforming data using simple, drag-and-drop functionalities.
These tools minimize the learning curve, enabling users to quickly grasp how to manipulate data.
3. Data governance #
While self-service data emphasizes user independence, it must also maintain data governance standards. This includes ensuring data quality, security, and compliance with regulations.
Administrators often set up protocols to manage access permissions, ensuring that sensitive data is protected and that users are working with accurate and reliable information.
4. Data preparation #
Data is rarely in a perfect state for analysis. Self-service data solutions provide tools for data cleaning, transformation, and integration. Users can preprocess data, handling tasks like dealing with missing values, standardizing formats, and combining datasets.
These features empower users to work with high-quality data, essential for meaningful analysis.
5. Visualization #
Visual representation of data is a powerful way to convey insights. Self-service data platforms offer visualization tools that enable users to create interactive charts, graphs, and dashboards.
Visualization not only simplifies complex data but also aids in understanding trends and patterns, making it easier for non-technical stakeholders to comprehend the significance of the data.
6. Collaboration #
Collaboration features encourage teamwork and knowledge sharing. Multiple users can work on the same dataset simultaneously, facilitating collaborative analysis.
Additionally, these platforms often allow users to share their visualizations and insights with others, fostering a collaborative environment within teams and organizations.
7. Automation #
Automation is a key aspect of advanced self-service data solutions. Machine learning algorithms and automation tools can assist users by suggesting insights, detecting patterns, and even predicting future trends based on historical data.
Automation streamlines the analysis process, making it more efficient and allowing users to focus on interpreting results rather than performing repetitive tasks.
8. Scalability #
Scalability refers to the ability of self-service data platforms to handle large volumes of data and a growing number of users.
These platforms are designed to scale horizontally, accommodating increasing data loads and user demands without sacrificing performance. Scalability ensures that self-service data solutions remain effective as an organization’s data requirements expand.
Self-service data empowers business users, analysts, and domain experts to explore and analyze data, which can lead to more agile decision-making and a better understanding of an organization’s operations, customers, and markets. It can help bridge the gap between technical experts and non-technical stakeholders, promoting a data-driven culture within an organization.
What is self-service data preparation? #
Self-service data preparation is a critical component of modern data analytics, designed to empower business users to access, clean, integrate, and prepare data for analysis without relying on specialized IT or data professionals.
This approach democratizes data handling, making it more accessible and efficient for users across various roles in an organization. Here’s a detailed breakdown of self-service data preparation:
1. Accessing data #
- Sources: Users can pull data from a variety of sources such as databases, cloud services, spreadsheets, and even unstructured data like text files.
- Connectivity: Self-service tools provide connectors or interfaces to easily link these disparate data sources.
2. Cleaning and standardizing data #
- Data Cleansing: Involves correcting or removing inaccurate, corrupted, incorrectly formatted, duplicate, or incomplete data within a dataset.
- Normalization: Standardizing formats to ensure data consistency, like converting date formats, standardizing text entries (e.g., ‘U.S.A’ to ‘USA’), and addressing null values.
3. Data integration #
- Combining data: Merging data from different sources to create a cohesive dataset. This could involve joining tables or appending data.
- De-duplication: Removing duplicate records to ensure the uniqueness of data.
4. Data transformation #
- Reshaping data: This includes pivoting, aggregating, or splitting data to fit the needs of analysis.
- Creating calculated fields: Users can add new data fields derived from existing data, like calculating the total sales from unit price and quantity.
5. Ensuring data quality #
- Validation: Applying rules to ensure data meets quality standards (e.g., no negative values for certain numeric fields).
- Anomaly detection: Identifying outliers or unusual patterns which might indicate data issues.
6. User-friendly interface #
- Drag-and-drop tools: These allow users to perform complex operations without coding.
- Visualizations: Visual interfaces to understand data transformations and relationships.
7. Data enrichment #
- Adding context: Integrating external data (like demographic information) to enrich the analysis.
8. Exporting and sharing #
- Output formats: Data can be exported in formats suitable for analytics tools or reporting software.
- Collaboration: Sharing capabilities to collaborate with other team members.
In essence, self-service data preparation is about putting the power of data in the hands of business users, enabling them to be more self-reliant and responsive in a data-driven business environment. It’s a key enabler in the broader movement towards democratizing data and analytics within organizations.
10 Steps roadmap for self-service data #
Creating a self-service data roadmap involves outlining a strategic plan to implement and enhance self-service data capabilities within an organization.
This roadmap serves as a guide to transition from traditional, centralized data management to a more democratized model where users across different departments can access, analyze, and visualize data on their own.
Here is a 10 step roadmap for self-service data:
- Assessment of current capabilities and needs
- Define objectives and scope
- Selecting the right tools and technologies
- Data governance and security
- Implementation plan
- Training and support
- Promotion of data culture
- Monitoring and evaluation
- Iterative enhancement
- Risk management
Here’s a detailed explanation of the key steps involved in developing a self-service data roadmap:
1. Assessment of current capabilities and needs #
- Current state analysis: Evaluate the existing data infrastructure, tools, and processes.
- Identify stakeholder needs: Understand the data requirements of different departments and users.
- Skill assessment: Assess the data literacy and technical skills of potential self-service data users.
2. Define objectives and scope #
- Business goals: Align the self-service initiative with overall business objectives.
- Scope definition: Determine which data, tools, and functionalities will be included in the self-service model.
3. Selecting the right tools and technologies #
- Tool evaluation: Choose self-service tools that are user-friendly, scalable, and secure. Consider factors like data integration capabilities, ease of use, and visualization options.
- Technology stack alignment: Ensure the selected tools integrate well with the existing technology stack.
4. Data governance and security #
- Data policies: Establish clear data governance policies that outline data access, quality, privacy, and security protocols.
- Role-based access: Implement role-based access control to ensure data security and compliance.
5. Implementation plan #
- Phased approach: Consider a phased rollout, starting with a pilot program before expanding to other departments.
- Integration: Integrate self-service tools with existing data sources and systems.
6. Training and support #
- Training programs: Provide comprehensive training to users, tailored to their skill levels and roles.
- Ongoing support: Establish support structures, like helpdesks or user communities, for continuous assistance and learning.
7. Promotion of data culture #
- Advocacy: Encourage leadership to advocate for a data-driven culture.
- Collaboration: Foster a collaborative environment where users share insights and best practices.
8. Monitoring and evaluation #
- Performance metrics: Define metrics to evaluate the success of the self-service initiative.
- Feedback mechanism: Collect regular feedback from users to identify challenges and opportunities for improvement.
9. Iterative enhancement #
- Continuous improvement: Regularly review and update the tools, processes, and training materials based on user feedback and evolving business needs.
- Scalability: Ensure the self-service model can scale with the growth of the business and data.
10. Risk management #
- Risk assessment: Identify and mitigate potential risks associated with self-service data analytics, such as data misinterpretation or misuse.
A well-crafted self-service data roadmap not only guides the implementation of self-service analytics tools but also plays a pivotal role in transforming the organizational culture to be more data-informed and agile.
What is the importance of self-service data? #
Self-service data is of paramount importance in today’s data-driven world. It offers numerous advantages that facilitate quicker decision-making, empower business users, enhance productivity, and ultimately drive organizations toward greater success.
This approach to data access and analysis has several key attributes that make it indispensable in various industries and sectors.
Key benefits of self-service data include :
- Faster decision-making
- Empowerment of business users
- Increased productivity
- Data-driven decision-making
- Scalability
1. Faster decision-making #
Self-service data empowers users to access and analyze data without delays.
Quick access to data and the ability to perform real-time analysis means that organizations can respond swiftly to market changes and emerging opportunities. This agility is essential in dynamic business environments.
2. Empowerment of business users #
User-friendly self-service data tools reduce the reliance on it or data specialists, enabling business users, domain experts, and analysts to work with data autonomously.
It fosters a data-driven culture within an organization, allowing individuals closer to business processes to harness the power of data.
3. Increased productivity #
Self-service data platforms streamline data-related tasks. Users can efficiently perform data preparation, analysis, and visualization, saving time and effort.
Automation and collaboration features also contribute to increased productivity by reducing manual work and encouraging teamwork.
4. Data-driven decision-making #
By making data more accessible and understandable, self-service data solutions promote data-driven decision-making at all organizational levels.
Informed decisions based on data insights tend to be more accurate and less reliant on intuition or guesswork.
5. Scalability #
Self-service data platforms are designed to scale with an organization’s needs. They can accommodate growing data volumes and user demands while maintaining performance and responsiveness.
This scalability ensures that the organization’s data capabilities can evolve alongside its data requirements.
The benefits of self-service data extend beyond convenience; they drive innovation, foster a data-driven culture, and ultimately contribute to an organization’s success in an ever-evolving landscape.
What is self-service data management? #
Self-service data management is a concept and set of practices that extend the principles of self-service data access and analysis to the broader data management process within an organization. It involves giving business users and data stakeholders more control and autonomy over data-related tasks beyond just analysis.
Self-service data management aims to streamline and simplify various data management activities, such as data integration, data quality, data cataloging, and data governance, by allowing non-technical users to participate in these processes. Here are key aspects of self-service data management:
Key aspects include:
- Data integration
- Data quality
- Data cataloging
- Data governance
- Data lifecycle management
- Data collaboration
- Data discovery
Now, let’s delve deeper into each of these aspects:
1. Data integration #
Self-service data management solutions often include data integration capabilities. This means that business users and data stakeholders can bring data from various sources together without extensive technical skills.
These tools typically provide a user-friendly interface for mapping, transforming, and loading data, making the process of data integration more accessible. Users can create data pipelines that connect to databases, APIs, cloud services, and more.
This not only speeds up the data preparation process but also reduces the reliance on IT teams for routine data integration tasks.
2. Data quality #
Data quality is crucial for making informed decisions. Self-service data management empowers users to participate in data quality improvement. These tools may include data profiling, cleansing, and enrichment features that help users identify and rectify data issues.
Users can clean and standardize data, detect and handle duplicates, and enrich data with additional information, all without requiring deep technical expertise. This ensures that the data used for analysis and reporting is accurate and reliable.
3. Data cataloging #
A well-organized data catalog is essential for data discoverability and understanding. Self-service data management solutions typically include data cataloging and metadata management features.
Users can add descriptions, tags, and annotations to datasets, making it easier for others in the organization to discover, comprehend, and trust the data assets available. This democratizes data documentation and cataloging, reducing the burden on data professionals.
4. Data governance #
While encouraging user autonomy in data management, self-service data management also enforces data governance policies.
Administrators can set up rules, access controls, and data lineage tracking to ensure data security, compliance with regulations, and accountability.
Users can participate in adhering to these policies, which is particularly important when handling sensitive or regulated data. This combination of autonomy and governance helps strike a balance between flexibility and data protection.
5. Data lifecycle management #
Data doesn’t remain static; it evolves over time. Self-service data management solutions allow users to participate in the data lifecycle. They can archive, purge, or move data as needed, ensuring that the organization is efficiently managing data storage costs and complying with data retention policies.
Users play an active role in deciding what data is archived or deleted, reducing the storage overhead and keeping the data environment efficient.
6. Data collaboration #
Collaboration features within self-service data management tools facilitate teamwork on data-related tasks. This includes jointly defining data standards, maintaining data quality, and ensuring consistent data definitions across the organization.
Users can collaborate on data documentation, data transformation logic, and data governance policies. Collaboration fosters a sense of ownership and shared responsibility for data assets, leading to better data management practices.
7. Data discovery #
Self-service data management solutions make it easier for users to discover and access relevant data assets. They provide search and data lineage features, allowing users to find the data they need for analysis or reporting more efficiently.
Data discovery empowers users to explore the organization’s data landscape and locate the datasets that are most relevant to their specific needs, which ultimately improves their productivity and decision-making capabilities.
By democratizing data management tasks, self-service data management encourages active participation from business users, reduces reliance on it departments, and enhances the overall efficiency and effectiveness of an organization’s data-related processes. It empowers users to take control of data management while ensuring data remains accurate, secure, and valuable to the business.
What are self-service data tools? #
Self-service data tools encompass a wide range of software applications and platforms designed to facilitate data access, analysis, and management by individuals with varying levels of technical expertise.
These tools empower users to work with data independently, reducing the reliance on IT or data specialists.
Some popular self-service data tools include: #
Tableau: Tableau is a widely used data visualization tool that enables users to create interactive and shareable dashboards, reports, and charts. It provides a user-friendly interface for exploring and visualizing data from various sources.
Power BI: Microsoft Power BI is a powerful business analytics tool that allows users to connect to different data sources, transform data, and create interactive reports and dashboards. It’s popular for its ease of use and integration with other Microsoft products.
QlikView and Qlik Sense: Qlik’s suite of products offers self-service data visualization and analytics. Users can explore data, create interactive visualizations, and share their insights with others.
Looker: Looker is a data exploration and business intelligence platform that provides users with the ability to build and share data models, create dashboards, and conduct ad-hoc analyses.
Alteryx: Alteryx is a data preparation and analytics platform that empowers users to blend, cleanse, and transform data from various sources without requiring advanced technical skills.
Google Data Studio: This free tool by Google allows users to create interactive and shareable reports and dashboards. It integrates seamlessly with other Google services, like Google Analytics and Google Sheets.
Domo: Domo is a cloud-based business intelligence and data visualization platform that offers a range of self-service features for data analysis and reporting.
IBM Cognos Analytics: IBM’s self-service data tool provides features for data exploration, dashboard creation, and reporting, along with robust analytics capabilities.
Sisense: Sisense is a business intelligence platform that enables users to easily prepare, analyze, and visualize data. It offers a combination of data integration and analytics in a single platform.
Yellowfin: Yellowfin is a business intelligence and analytics platform that offers self-service data visualization and reporting tools for data exploration and decision-making.
Dundas BI: Dundas BI is a flexible business intelligence and data analytics platform that allows users to create interactive dashboards, reports, and scorecards.
TIBCO Spotfire: TIBCO Spotfire is an analytics and data visualization tool that provides advanced data exploration, interactive dashboards, and predictive analytics capabilities.
Trifacta: Trifacta is a data preparation platform that empowers users to clean, structure, and transform data for analysis using a visually interactive interface.
OpenRefine: OpenRefine (formerly Google Refine) is an open-source data cleaning and transformation tool that helps users clean, normalize, and enhance messy data.
These tools vary in terms of their features, complexity, and pricing, but they all share the goal of making data more accessible and manageable for a broader range of users, from business analysts to non-technical stakeholders. The choice of a self-service data tool depends on an organization’s specific needs and the level of technical expertise of its users.
Summing up #
Self-service data is pivotal for modern businesses, as it brings data closer to the people who need it most. It democratizes data access and analysis, empowering organizations to make quicker, more informed decisions, increase efficiency, and stay competitive in an increasingly data-centric world.
Self-service data tools are a diverse range of software applications and platforms that empower users to access, analyze, and manage data independently, reducing dependence on IT or data specialists.
Popular tools like Tableau, Power BI, and Looker provide user-friendly interfaces for data exploration, visualization, and reporting.
These tools cater to users with varying levels of technical expertise and play a crucial role in fostering a data-driven culture within organizations. The choice of a self-service data tool depends on an organization’s specific requirements and the technical skills of its users.
Self-service data: Related reads #
- What is Self-Service BI and What Are Its Benefits?
- Self Service Analytics Mastery: From Novice to Data Champion
- Traditional BI vs Self-Serve BI: Which One Suits You the most?
- Buildertrend Drives Trusted, Self-service Data with Atlan
- What Is a Data Lake? Definition, Architecture & Solutions
- What is Data Governance? Its Importance & Principles
- Data Governance Program: How to Build It in 7 Steps?
- 7 Best Practices for Data Governance
- Creating a Data Governance Strategy Mapped to Outcomes
- What is DataOps & How Does it Help Data Management?
- Modern Data Management: 8 Things You Can Gain From It
Share this article