Self Service Analytics: What is It and Why is It Important?
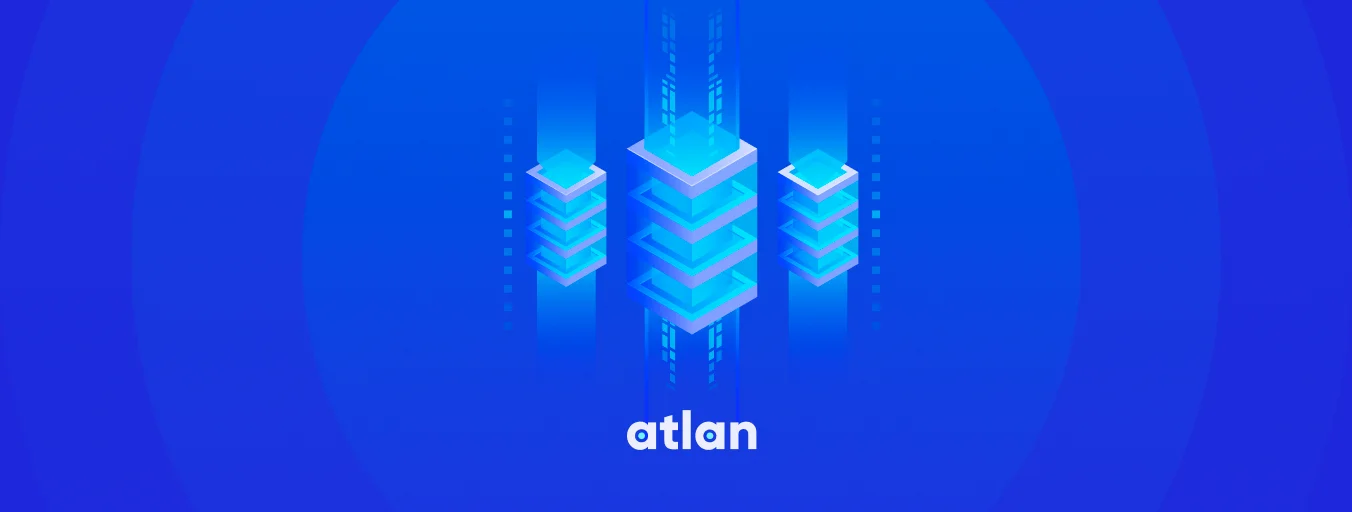
Share this article
According to Gartner “Self-Service Analytics is a form of business intelligence (BI) in which line-of-business professionals are enabled and encouraged to perform queries and generate reports on their own, with nominal IT support.”
Imagine this: every employee in your organization, from the marketing whiz to the finance guru, armed with the ability to access, analyze, and transform data into actionable insights, all at their fingertips.
Self service analytics stands as a beacon of empowerment placing the tools of data exploration, analysis, and visualization squarely into the hands of non-technical users.
This liberates organizations from the confines of traditional data silos and technical dependencies.
Modern data problems require modern solutions - Try Atlan, the data catalog of choice for forward-looking data teams! 👉 Book your demo today
In this article, we will explore:
- What is self service analytics?
- How does self service analytics work?
- What are the core components of self service analytics?
- What is the purpose of self service analytics?
- Self service analytics tools
Ready? Let’s dive in!
Table of contents #
- What is self service analytics?
- Self service analytics strategy: How does it work?
- Self-service analytics: Purpose and advantages
- Self service analytics tools
- Self service analytics platforms
- Frameworks for self service analytics
- In summary
- Self service analytics: Related reads
What is self service analytics? #
Self service analytics is a paradigm shift in the way organizations approach data analysis. It’s the practice of enabling business users, regardless of their technical background, to independently access and manipulate data to generate insights and make informed decisions.
Traditional analytics often required specialized skills, leading to bottlenecks in data accessibility and analysis. Self service analytics democratizes data, making it readily available to those who need it, when they need it.
4 Core components of self service analytics #
In the age of data-driven decision-making, self service analytics has emerged as a transformative force, revolutionizing the way organizations interact with their data. At its core are powerful components that empower users across all departments to access, analyze, and visualize data with ease.
In this exploration, we dive deep into the essential building blocks of self service analytics, unveiling the user-friendly tools that make data analysis accessible, the data preparation and integration processes that ensure data quality, the captivating world of visualizations, and the democratization of data access.
The four key components of self service analytics are:
- User-friendly tools
- Data preparation and integration
- Visualizations
- Data accessibility
Let’s discuss them in detail:
1. User-friendly tools #
- Drag-and-drop interfaces: Self service analytics tools are designed with user-friendliness in mind. They often feature drag-and-drop interfaces, allowing users to select and arrange data elements, charts, and visualizations intuitively. This eliminates the need for complex coding or scripting, making data analysis accessible to non-technical users.
- Pre-built templates: Many self service analytics tools offer pre-built templates for common data analysis tasks. These templates provide a starting point for users, streamlining the process of creating reports, dashboards, and visualizations. Users can customize these templates to suit their specific needs.
- WYSIWYG (What you see is what you get) editing: WYSIWYG editing allows users to see the visual representation of their data as they build reports and dashboards. This real-time feedback enables users to make instant adjustments and fine-tune their visualizations for better insights.
- Interactive dashboards: Self service analytics platforms often include interactive dashboard creation tools. Users can design dashboards that allow for drill-downs, filtering, and dynamic interactions with data. This interactivity enhances the depth of analysis and exploration.
2. Data preparation and integration #
- Data wrangling: Data preparation tools within self service analytics platforms enable users to perform data wrangling tasks. This includes cleaning, transforming, and reshaping data to make it suitable for analysis. Users can handle tasks like removing duplicates, filling missing values, and creating calculated fields.
- Data blending: Self service analytics tools allow users to combine data from multiple sources seamlessly. Users can integrate data from databases, spreadsheets, cloud storage, web services, and more. Data blending ensures a holistic view, enabling users to analyze data from various angles.
- Automated ETL (Extract, transform, load): Some self service analytics platforms offer automated ETL processes. These processes streamline data extraction, transformation, and loading tasks. Automated ETL reduces manual data preparation efforts and ensures data consistency and accuracy.
- Data profiling: Data profiling features assist users in understanding the characteristics of their data. This includes data distribution, data types, and summary statistics. Data profiling helps users identify data quality issues and make informed decisions about data cleaning and transformation.
3. Visualizations #
- Chart and graph options: Self service analytics tools provide a wide range of chart and graph options. Users can choose from bar charts, line graphs, pie charts, scatter plots, heatmaps, and more. The availability of diverse visualization types allows users to select the most appropriate format for presenting their data.
- Customization: Users have the flexibility to customize the appearance of visualizations. They can adjust colors, fonts, labels, legends, and other design elements to align with their branding or reporting standards. Customization options enhance the aesthetics and readability of reports and dashboards.
- Interactive elements: Interactive elements like tooltips, filters, and parameters make visualizations more engaging and informative. Users can hover over data points to view additional details, apply filters to specific data subsets, and change visualization settings on the fly.
- Real-time updates: Visualizations in self service analytics dashboards often support real-time or near-real-time data updates. This ensures that users always have access to the most current information, critical for dynamic decision-making.
4. Data accessibility #
- Data connectors: Self service analytics tools provide connectors to a wide range of data sources. These connectors allow users to establish connections to databases, cloud storage, spreadsheets, web services, and other data repositories. The availability of connectors simplifies data access.
- Data exploration: Users can explore data from various angles and perspectives. Self service analytics platforms enable users to slice and dice data, apply filters, sort data, and group information to gain deeper insights. The ability to explore data interactively facilitates hypothesis testing and discovery.
- Collaboration features: Collaboration features within self service analytics tools enable users to share their analyses, reports, and dashboards with colleagues. Collaborators can comment, provide feedback, and collaborate on data-driven decision-making, fostering teamwork and knowledge sharing.
These core components collectively empower users to unlock the potential of their data and make data-driven decisions with confidence. Self service analytics tools put the power of data analysis directly into the hands of those who understand the domain and context, resulting in more informed and agile organizations.
Self service analytics strategy: How does it work? #
To harness the full potential of self service analytics, organizations need more than just tools; they need a strategy of how is this working. In this section, we’ll guide you through the essential steps to get started with self service analytics.
- Getting started with self service analytics
- User training and support
- Data assessment and cleanup
- Data governance committee
Now, let’s understand them in detail:
1. Getting started with self service analytics #
Implementing self service analytics requires a well-thought-out strategy. Organizations should begin by identifying their goals, data sources, and key stakeholders.
Creating a roadmap that outlines the steps involved, from tool selection to user training, is essential. Addressing potential challenges, such as data quality and governance, should be part of the strategy.
2. User training and support #
User training is a critical component of a successful self service analytics strategy. Providing comprehensive training programs ensures that employees can effectively use the chosen analytics tools.
Ongoing support and resources, such as documentation and online communities, should be available to help users troubleshoot issues and expand their analytical skills.
3. Data assessment and cleanup #
Before diving into self service analytics, organizations should assess their data sources and perform data cleanup. Ensuring data quality and relevance is crucial for accurate analysis and reliable insights.
4. Data governance committee #
Establishing a data governance committee or team responsible for overseeing data quality, security, and compliance is essential. This ensures that self service analytics aligns with data governance policies and best practices.
Self-service analytics: Purpose and advantages #
Why does self service analytics matter? What’s the big fuss about empowering non-technical users with data access? In this section, we will explore the purpose and advantages of self service analytics.
- Empowering non-technical users
- Accelerated decision-making
- Cost efficiency
- Data-driven culture
- Resource efficiency
Let’s discuss them in detail:
1. Empowering non-technical users #
The primary purpose of self service analytics is to empower non-technical users, such as marketers, sales professionals, and business managers, to harness the power of data.
By providing user-friendly interfaces and intuitive tools, self service analytics platforms bridge the gap between data and decision-makers. This empowerment leads to quicker, data-driven decisions and a more agile organization.
2. Accelerated decision-making #
In the modern business landscape, speed is of the essence. Self service analytics enables organizations to make faster decisions by reducing the time it takes to access and analyze data. Real-time or near-real-time insights can be critical in responding to market changes, customer demands, and competitive pressures.
Case studies demonstrate how organizations have leveraged self service analytics to gain a competitive edge through rapid decision-making.
3. Cost efficiency #
Traditional analytics approaches often require significant investments in IT infrastructure and data analyst salaries. Self service analytics can result in cost efficiency by reducing the dependency on IT resources.
Organizations can optimize their data operations and allocate resources more strategically, ultimately saving money.
4. Data-driven culture #
Self service analytics fosters a data-driven culture within an organization. When employees at all levels have access to data and can analyze it independently, they are more likely to incorporate data into their decision-making processes, resulting in a culture that values data-driven insights.
5. Resource efficiency #
Traditional analytics often required specialized teams to handle data requests and analysis. Self service analytics reduces the burden on these teams, allowing them to focus on more complex tasks while enabling non-technical users to handle routine data analysis.
Self service analytics: 5 Essential tools to know #
Self service analytics tools are the magic wands that turn raw data into actionable insights. In this section, we’ll take you on a tour of the dynamic landscape of user-friendly interfaces and data preparation tools that make self service analytics possible.
With tools like Tableau, Power BI, and Alteryx, the world of data analysis is at your fingertips. Discover how these tools empower individuals to explore data, create stunning visualizations, and uncover the hidden gems buried within their datasets.
- Tableau
- Microsoft Power BI
- QlikView/Qlik Sense
- Google Data Studio
- Alteryx
Let’s look at them in detail:
1. Tableau #
Tableau is a renowned self service analytics tool that offers a wide range of data visualization and exploration features. It’s known for its user-friendly interface and robust capabilities for data analysis and storytelling.
Key features:
- Drag-and-drop interface: Tableau’s intuitive drag-and-drop interface allows users to create interactive dashboards and reports without coding.
- Data blending: Users can blend data from various sources, including databases, spreadsheets, and cloud storage, to create comprehensive analyses.
- Numerous visualization options: Tableau offers a rich library of chart types, maps, and graphs for creating compelling visualizations.
- Real-time data integration: Users can connect to real-time data sources to ensure they are always working with the latest information.
- Data exploration: Tableau provides in-depth data exploration tools, such as filtering, sorting, and calculated fields, to uncover insights.
Tableau is widely used in industries like finance, marketing, healthcare, and more for data analysis, reporting, and data-driven decision-making.
2. Microsoft Power BI #
Microsoft Power BI is a popular self service business intelligence tool that seamlessly integrates with other Microsoft products. It’s known for its robust reporting and data visualization capabilities.
Key features:
- Integration with Microsoft ecosystem: Power BI integrates seamlessly with Excel, SharePoint, and other Microsoft applications, making it a preferred choice for organizations using Microsoft products.
- Natural language queries: Users can ask questions in plain language and receive instant visual responses, thanks to its natural language processing (NLP) capabilities.
- Customizable dashboards: Power BI allows users to create personalized dashboards and reports with a variety of visualization options.
- Data transformation: Users can clean, transform, and shape data using Power Query, a powerful ETL tool integrated into the platform.
- Collaboration: The tool offers collaboration features, such as sharing reports and dashboards with colleagues and stakeholders.
Microsoft Power BI is utilized across industries for data visualization, interactive reporting, and business intelligence.
3. QlikView/Qlik Sense #
QlikView and Qlik Sense are products from Qlik, known for their associative data model that enables users to explore data relationships intuitively.
Key features:
- Associative data model: Qlik’s unique associative data model allows users to explore data relationships dynamically, making it easier to discover hidden insights.
- Data integration: Users can easily integrate data from multiple sources, create data models, and build interactive dashboards.
- Responsive design: Qlik Sense offers responsive design, ensuring dashboards and reports adapt to different screen sizes and devices.
- Advanced analytics: Qlik provides advanced analytics capabilities, including predictive analytics and data storytelling.
- Enterprise-grade security: Qlik offers robust security features to protect sensitive data.
QlikView and Qlik Sense are used in industries like retail, healthcare, manufacturing, and finance for data exploration, data visualization, and predictive analytics.
4. Looker studio ( previously Google Data Studio) #
Looker studio ( previously Google Data Studio) is a free self service analytics and data visualization tool that integrates seamlessly with other Google products.
Key features:
- Google integration: Users can connect to various Google products, such as Google Analytics, Google Ads, and Google Sheets, to analyze and visualize data.
- Customizable templates: Google Data Studio offers customizable report templates and a drag-and-drop interface for creating interactive dashboards.
- Collaboration: Users can collaborate in real-time with colleagues on reports and dashboards.
- Data sharing: Reports and dashboards can be easily shared with stakeholders, making it accessible to a wider audience.
- Integration with other data sources: Besides Google products, users can connect to various data sources, including databases, CSV files, and more.
Google Data Studio is commonly used for marketing analytics, website performance reporting, and data visualization across various industries.
5. Alteryx #
Alteryx is a self service data preparation and analytics platform that enables users to automate data workflows and perform advanced analytics.
Key features:
- Data preparation: Alteryx offers a wide range of data preparation tools to clean, transform, and enrich data.
- Workflow automation: Users can create automated workflows to streamline data processing and analysis.
- Predictive analytics: Alteryx includes predictive analytics capabilities, allowing users to build and deploy machine learning models.
- Spatial analytics: The platform supports spatial analytics, making it suitable for location-based data analysis.
- Integration with BI tools: Alteryx can integrate seamlessly with BI tools like Tableau and Power BI to enhance data analysis capabilities.
Alteryx is commonly used in industries such as finance, retail, and healthcare for data blending, predictive modeling, and automation of data workflows.
These are just a few examples of self service analytics tools, each offering unique features and capabilities to cater to the diverse needs of organizations and users. The choice of the right tool depends on factors like data volume, complexity, integration requirements, and user preferences.
Self service analytics platforms #
The world of self service analytics platforms is a playground for organizations seeking to unlock the power of their data.
Whether you’re drawn to the cloud’s scalability or prefer on-premises solutions for data control, this section has it all.
The following are some of the key self service analytics platforms:
- Cloud-based platforms
- On-premise solutions
- Data integration flexibility
- Collaborative features
- Customization and branding
Let’s look at them in detail:
1. Cloud-based platforms #
Cloud-based self service analytics platforms offer scalability, flexibility, and accessibility. Services like AWS QuickSight and Google Data Studio provide users with the ability to create and share reports and dashboards in the cloud.
This approach reduces infrastructure costs and simplifies collaboration among remote teams.
2. On-premises solutions #
Some organizations prefer on-premises self service analytics solutions for data security and compliance reasons. Software such as SAP BusinessObjects and IBM Cognos can be deployed within an organization’s own infrastructure.
While this approach may require more extensive IT support, it provides greater control over data.
3. Data integration flexibility #
Cloud-based self service analytics platforms often offer extensive data integration options, allowing organizations to connect to various data sources, from cloud storage to databases and APIs.
4. Collaborative features #
These platforms frequently include collaborative features such as real-time sharing and commenting on dashboards and reports. This promotes teamwork and knowledge sharing among users.
5. Customization and branding #
Many self service analytics tools allow organizations to customize the look and feel of their dashboards, reports, and visualizations to align with branding and user preferences.
Frameworks for self service analytics #
To build a robust self service analytics ecosystem, organizations need a solid foundation. That’s where frameworks come into play. In this section, we’ll introduce you to the world of data governance frameworks, where data ownership, quality standards, security, and cataloging become the cornerstones of responsible data management.
We’ll also explore the concept of a self service analytics maturity model, which helps organizations assess their current state and chart a course for continuous growth and improvement in their analytics capabilities.
- Data governance framework
- Self service analytics maturity model
- Data cataloging and metadata management
- Data privacy and security controls
- Advanced analytics governance
Let’s explore each in detail:
1. Data governance framework #
Effective data governance is essential for maintaining data quality, privacy, and compliance in self service analytics. Implementing a data governance framework ensures that data is used responsibly and in accordance with regulations.
This framework defines roles and responsibilities, establishes data ownership, and sets policies for data access and usage.
2. Self service analytics maturity model #
A self service analytics maturity model helps organizations assess their current state and plan for growth. It typically consists of several stages, from basic data access to advanced analytics and AI-driven insights.
By understanding where they fall on the maturity spectrum, organizations can identify areas for improvement and set realistic goals.
3. Data cataloging and metadata management #
Effective metadata management and data cataloging enable users to discover and understand data assets more easily, facilitating data exploration and analysis.
4. Data privacy and security controls #
Frameworks should include comprehensive data privacy and security controls to ensure compliance with regulations like GDPR and HIPAA. Protecting sensitive data is paramount.
5. Advanced analytics governance #
As organizations mature in their self service analytics journey, governance frameworks should encompass advanced analytics models and AI-driven insights, ensuring that these innovations adhere to governance standards.
In summary #
Self service analytics is not just a buzzword; it’s a transformative approach that empowers organizations to harness the full potential of their data. By breaking down traditional data silos and putting the power of analysis into the hands of non-technical users, self service analytics accelerates decision-making, enhances collaboration, and drives cost efficiencies.
With user-friendly tools, effective strategies, and a keen eye on emerging trends, organizations can embark on a data-driven journey that positions them for success in today’s dynamic business landscape.
As organizations continue to evolve their self service analytics practices and embrace innovations like AI and augmented analytics, the possibilities are boundless. The democratization of data is a powerful force that empowers individuals across all departments to become data champions.
It helps uncover insights that drive growth, improve customer experiences, and adapt to ever-changing market conditions.
To thrive in the data-driven era, organizations must recognize that self service analytics is not just a tool; it’s a strategic imperative that can revolutionize the way they operate and compete in the digital age.
Self service analytics: Related reads #
- Self-Service Data: Definition, Benefits, and Step-by-Step Guide
- Data Governance vs Data Analytics: Which is Best for You?
- Self-Service BI: The Ultimate Guide for You in 2023!
- Data analytics in travel industry: A 2023 guide
- Traditional BI vs Self-Serve BI: Which One Suits You the most?
- Data Transformation: Definition, Processes, and Use Cases
- Data Cleaning, Management, and Tagging: The Best Practices
- Data Consistency 101: Causes, Types and Examples
- Why Visualize Data?
- What is Data Modeling? 5 Steps to Build a Robust Model in 2023
Share this article