The Importance of Data Quality in Financial Services: 5 Reasons!
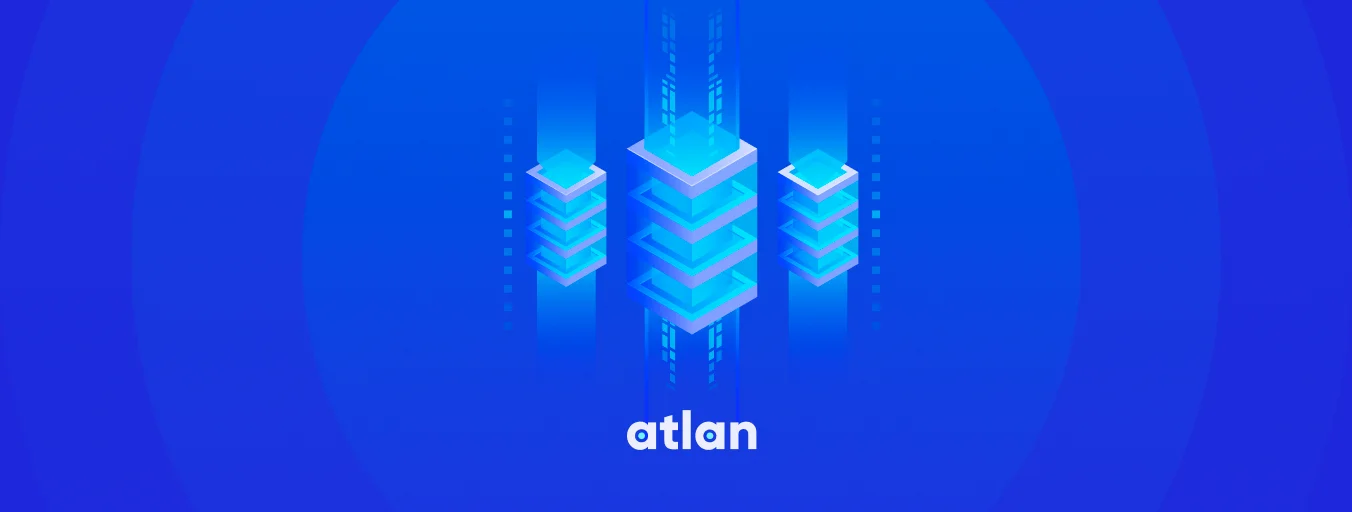
Share this article
According to recent research by Gartner, organizations attribute an average annual loss of $15 million for having poor data quality.
Like any engine, financial services can catastrophically fail if the wrong fuel flows through their systems. In the world of banking, insurance, and investments, that fuel is data. Here is where the importance of data quality in financial services comes in !
When data goes bad, the fallout can be severe, translating into million-dollar fines, customer attrition, flawed lending decisions, and tarnished reputations.
For banks, insurers, and investment firms striving for stability and success, the path forward must start with data excellence.
Modern data problems require modern solutions - Try Atlan, the data catalog of choice for forward-looking data teams! 👉 Book your demo today
With this article, let us understand three key things about data quality in financial services:
- Importance of good data quality in financial services
- Repercussions of poor quality data
- How to stay away from poor-quality data
Let us dive in!
Table of contents #
- The Importance of data quality in financial services: 5 Reasons to know!
- 5 Ways poor data quality can hold the growth of your financial services
- 5 Ways financial how services can steer away from poor quality data
- Summing up
- Importance of data quality in financial services: Related reads
The Importance of data quality in financial services: 5 Reasons to know! #
The importance of data quality in financial services cannot be overstated. In a sector that relies heavily on accurate, timely, and comprehensive information, poor data quality can lead to disastrous consequences for both the organization and its clients.
Here are several reasons why maintaining data quality is important for your financial services:
- Risk management
- Regulatory compliance
- Customer satisfaction
- Operational efficiency
- Competitive advantage
Let us look at them in detail:
1. Risk management #
High-quality data is pivotal for robust risk management in financial organizations. Accurate data feeds into models that predict credit risk, market volatility, and potential fraud.
These models guide everything from loan approval to investment strategies. Poor data can lead to bad decisions, such as lending to high-risk clients or making unwise investments, causing financial losses that could have been avoided.
2. Regulatory compliance #
Financial institutions operate under a complex regulatory framework, including laws like the Dodd-Frank Act, GDPR, and Anti-Money Laundering directives.
Accurate data is crucial for compliance reporting, and failure to maintain data quality can result in severe penalties. Regulatory bodies rely on this data to assess the institution’s compliance, and any discrepancies can lead to audits, fines, or legal repercussions.
3. Customer satisfaction #
Today’s customers expect seamless and personalized services, something that’s impossible without high-quality data. Accurate data helps in correctly identifying customer needs, preferences, and behaviors, which are crucial for tailoring services.
Poor data, on the other hand, can result in incorrect billing, failed transactions, or irrelevant product recommendations, all contributing to customer dissatisfaction and attrition.
4. Operational Efficiency #
Data quality directly impacts the efficiency of day-to-day operations. Clean and accurate data reduces the time and resources spent on data verification and correction.
It minimizes errors in transaction processing, billing, and customer communications, thereby lowering operational costs. Inefficient operations due to poor data quality not only increase expenses but also negatively affect customer service.
5. Competitive advantage #
In the data-driven world of finance, high-quality data provides a significant competitive edge. Accurate data allows financial institutions to glean actionable insights for improving services, entering new markets, or launching new products.
Poor data quality undermines these strategic decisions, making it difficult to outperform competitors, thereby leading to lost opportunities and stagnating growth.
For financial services, the importance of data quality cannot be overstated. It is the backbone for risk management, regulatory compliance, customer satisfaction, operational efficiency, and competitive advantage. Investing in high-quality data isn’t just an operational necessity; it’s a strategic imperative.
Beware! 5 Ways poor data quality can hold the growth of your financial services #
Poor data quality in banking isn’t just an operational nuisance; it poses substantial risks to the integrity, performance, and credibility of financial institutions. Understanding these consequences can underscore why importance of data quality in financial services should never be compromised.
Here are several ways how poor data quality can uphold the growth of financial services:
- Financial misreporting
- Strategic errors
- Decreased trust
- Ineffective marketing
- Security risks
- Credit risk misjudgment
- Human resource imbalances
Let us understand each of them in detail:
1. Financial misreporting #
Poor data quality can significantly distort financial reports. When financial statements are inaccurate, it affects both internal decision-making and external trust. For instance, inflated revenue figures could mislead the management into making wrong budget allocations.
Externally, investors may lose faith, and the bank could face legal repercussions for misrepresentation, resulting in a compounded financial mess.
2. Strategic errors #
Decisions about market entry, mergers, or even daily operations rely on data. Poor quality data can mislead decision-makers into implementing strategies that are doomed from the start.
For example, bad market research data could make a bank enter a market where there’s no real demand, resulting in a loss of investment and credibility.
3. Decreased trust #
When stakeholders can’t rely on the bank’s data, trust is eroded. This goes beyond customers and includes employees, shareholders, and regulators.
A single instance of data discrepancy can raise questions about the bank’s credibility, necessitating extra audits and oversight that drain resources and can damage reputation long-term.
4. Ineffective marketing #
High-quality data is crucial for effective marketing strategies. Poor data can lead to wrong customer segmentation and targeting, wasting both time and money.
For instance, if bad data leads to sending mortgage offers to students with no income, the campaign will incur costs while generating no revenue.
5. Security risks #
Faulty or incomplete data can mask the tell-tale signs of security risks or fraud. For example, if transaction data is not accurately recorded or analyzed, the bank might overlook fraudulent activities. This makes the institution vulnerable to cyber-attacks and could lead to financial loss and reputational damage.
6. Credit risk misjudgment #
Banks rely on data to assess the creditworthiness of individuals and corporations. Poor data quality can lead to inaccurate credit scores, causing loans to be given to high-risk parties. When these loans default, the bank incurs a financial loss, and its loan portfolio’s integrity is compromised.
7. Human resource imbalances #
Poor data can also affect human resource management. Inaccurate performance metrics or skewed employee satisfaction surveys can lead to misguided HR strategies. This can result in talent attrition, reduced productivity, and an overall decline in workplace morale, which further dampens the bank’s performance and standing.
The impact of poor data quality in banking can be far-reaching, affecting everything from financial performance to customer relationships and regulatory standing. The consequences are not only immediate but can also result in long-term setbacks, making it imperative for banks to invest in maintaining high-quality data.
5 Ways how financial services can steer away from poor quality data #
Data quality issues are like quicksand for financial services—easy to fall into but hard to escape. Ensuring high-quality data is critical for avoiding the many pitfalls that come with inaccurate or incomplete information.
Here are 5 tips on staying away from the poor data quality:
- Data governance framework
- Data quality audits
- Employee training
- Technological investment
- Vendor and partner assessment
Let us understand these pointers in detail:
1. Data governance framework #
A data governance framework sets the foundation for data quality by defining policies, standards, and responsibilities related to data management. It provides guidelines for data collection, storage, and usage across various departments.
By having a standardized set of procedures, financial services can ensure consistency and accuracy in data, which is essential for compliance, risk assessment, and strategic planning.
2. Data quality audits #
Regular audits of data quality help identify and rectify errors, inconsistencies, and redundancies in stored data. This proactive approach allows financial institutions to clean their databases, enhancing the reliability of their data-driven insights.
Audits can be specifically tailored to focus on critical areas such as customer information, financial transactions, or compliance-related data, making them highly effective.
3. Employee training #
Employees often interact with data at multiple touchpoints, from customer service to account management. Training staff on the importance of data accuracy and the procedures for data input and handling reduces human errors.
A well-trained workforce understands the importance of data quality in financial services and is equipped with the skills to maintain it, thereby becoming an asset in the data governance strategy.
4. Technological investment #
Investing in state-of-the-art data management and analytics tools can automate many aspects of data quality maintenance. These tools offer features like real-time validation, automatic error correction, and deduplication.
By reducing manual handling, the chances of human errors are minimized, making the investment pivotal for maintaining high data quality in the long run.
5. Vendor and partner assessment #
Financial services often rely on third-party vendors and partners for services like cloud storage, data analytics, or customer management systems. It’s crucial to conduct regular assessments of these third parties to ensure they meet data quality and security standards.
Vendor assessments involve reviewing contractual obligations related to data and inspecting their compliance with data governance protocols, safeguarding the institution against external risks to data quality.
Steering away from poor quality data is a multi-pronged effort that requires attention at organizational, technological, and human levels. By implementing a sound data governance framework, conducting regular audits, training employees, investing in technology, and carefully assessing vendors, financial services can significantly minimize the risks associated with poor data quality.
Summing up #
Ultimately, financial services must embrace data excellence as a core strategic priority integral to their success. Implementing robust governance frameworks, continuous monitoring, and a culture of quality are essential building blocks.
While improving data quality demands investment, the return will be manifold in the form of reduced risks, operational excellence, and customer trust. In partnership with clients, regulators, and technology partners, financial sector leaders can foster an ecosystem where high-quality data flows smoothly across boundaries.
As data grows more complex and missions more data-driven, these efforts will only gain urgency over time. For the financial sector, the path to stability and leadership inevitably relies on recognizing data quality as a foremost business imperative.
Importance of data quality in financial services: Related reads #
- Data Quality Measures: Best Practices to Implement
- Data Quality Explained: Causes, Detection, and Fixes
- How to Improve Data Quality in 12 Actionable Steps?
- 6 Reasons Why Data Quality Needs a Data Catalog
- A Blueprint for Bulletproof Data Quality Management
- Data Quality vs Data Governance: How Are They Related?
- Data Quality and Observability - Why Are They Important?
- Resolving Data Quality Issues in the Biggest Markets
- 9 Components to Build the Best Data Quality Framework
Share this article