Predictive Data Quality & Observability: A Complete Guide!
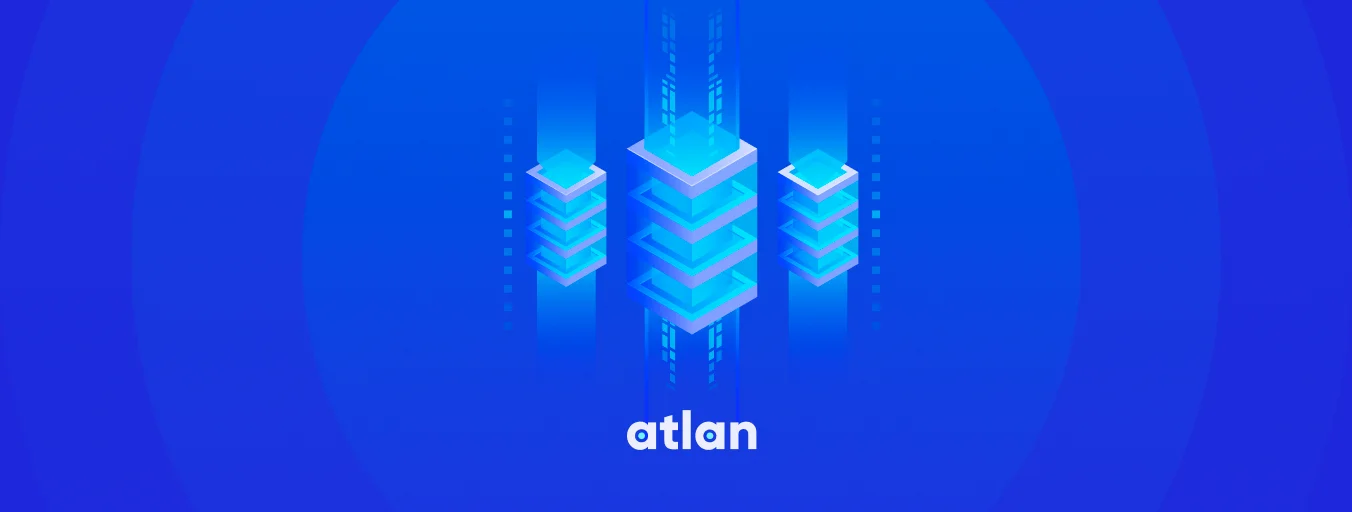
Share this article
According to Gartner, the cost of data downtime can skyrocket to over $5,600 per minute. These data issues can affect both the monetary and productivity levels of your business. This is where predictive data quality and observability become critical.
While predictive data quality applies forward-looking analytics to anticipate data issues before they occur. On the other hand data observability offers real-time visibility into the health and behaviour of complex data ecosystems.
Together, these capabilities allow organizations to optimize data reliability, accuracy, and usability.
Modern data problems require modern solutions - Try Atlan, the data catalog of choice for forward-looking data teams! 👉 Book your demo today
In this article, we will explore the immense power of predictive data quality and observability - from driving efficiency to enabling informed decision-making.
We will also discuss best practices for implementation to help organizations unlock the full potential of their data.
Let us dive in!
Table of contents #
- What is predictive data quality and how is it different from data observability?
- 5 Benefits of implementing predictive data quality and observability
- 5 Step process to implement predictive data quality and observability
- 7 Best practices for predictive data quality and observability
- Summing up
- Predictive data quality and observability: Related reads
What is predictive data quality and how is it different from data observability? #
Predictive data quality is a proactive approach to managing and improving the quality of data in an organization. It leverages predictive analytics to forecast issues before they occur.
Predictive data quality is an essential element in modern data governance strategies, empowering organizations to proactively manage their data assets. The forward-looking approach offers clear advantages, from cost savings to operational improvements.
To know more about predictive data quality check out the following article → Predictive Data Quality: What is It & How to Go About It.
Below is a table that highlights the key differences between predictive data quality and data observability:
Aspect | Predictive data quality | Data observability |
---|---|---|
Primary focus | Ensuring the quality and reliability of data used for predictive analytics. | Monitoring the entire data pipeline and ecosystem to understand its current state and performance. |
Goal | To make sure that the data feeding into predictive models is accurate, consistent, and timely. | To provide real-time insight into data health, lineage, and anomalies throughout the data lifecycle. |
Scope | Typically limited to the datasets and features used in predictive models. | Covers all data within an organization, not just those used for predictive analytics. |
Methods | Data cleansing, transformation, and validation specific to predictive modeling needs. | Real-time monitoring, logging, alerting, and tracing across the data pipeline. |
Outcome | Improved accuracy and effectiveness of predictive models. | Increased transparency, trustworthiness, and reliability of all data. |
Key metrics | Completeness, uniqueness, timeliness, relevance, and consistency of data specific to predictive models. | Latency, throughput, error rates, and other metrics related to overall data health and performance. |
Audience | Primarily data scientists and analysts focused on predictive analytics. | Data engineers, data scientists, analysts, and business stakeholders concerned with overall data health. |
Time orientation | Often forward-looking, as it aims to ensure that future predictions are as accurate as possible. | Usually real-time or near-real-time, focusing on the immediate state and issues within the data pipeline. |
Regulatory focus | May be tailored to meet specific regulatory requirements related to the data used in predictive analytics. | Broadly aimed at ensuring compliance across all data, such as GDPR, HIPAA, etc. |
Both predictive data quality and data observability are crucial for an organization’s data strategy but they serve different, albeit complementary, roles.
5 Benefits of implementing predictive data quality and observability #
In today’s data-driven landscape, predictive data quality and observability are not just optional but essential for any organization. They empower companies to not only react to issues but anticipate them, thus optimizing decision-making and operations.
Let us know the benefits of implementing predictive data quality and observability:
- Operational efficiency
- Enhanced decision-making
- Risk mitigation
- Compliance and trust
- Competitive advantage
Let us understand them in detail:
1. Operational efficiency #
Predictive data quality and observability contribute to streamlining operations. By forecasting potential issues and monitoring data systems in real-time, organizations can avoid the disruptions and costs associated with data errors and system failures.
2. Enhanced decision-making #
High-quality, observable data is vital for accurate analytics and reporting, which are the backbone of informed decision-making. Predictive capabilities add another layer by providing foresight, allowing companies to make proactive choices rather than reactive corrections.
3. Risk mitigation #
Predictive data quality helps in anticipating errors and issues that could lead to operational risks or flawed decision-making. Observability provides the diagnostics to understand these risks in real-time, offering a chance to address them proactively.
4. Compliance and trust #
Regulatory compliance requires stringent data quality and reporting standards. Predictive quality and observability facilitate this by ensuring that data is reliable, consistent, and transparent, thereby building trust among stakeholders and regulatory bodies.
5. Competitive advantage #
In a marketplace where data is a significant asset, having superior data quality and system observability can offer a competitive edge. It enables faster, more accurate decision-making, and more reliable operations, setting a company apart from competitors who are not as data-mature.
The importance of predictive data quality and observability transcends departments and industries. It is foundational to operational efficiency, risk management, and strategic decision-making. By investing in these aspects, organizations are not merely keeping up with current trends but are positioning themselves for future success in a data-centric world.
5-Step process to implement predictive data quality and observability #
Successfully implementing predictive data quality and observability requires a systematic approach.
Below is a 5-step process that covers both dimensions in an integrated manner:
- Data audit and inventory
- Establish metrics and benchmarks
- Tool selection and implementation
- Predictive modeling and analytics
- Continuous monitoring and feedback loop
Let us understand them in detail:
1. Data audit and inventory #
- Objective: Understand the current state of data in the organization.
- Actions: catalog all data sources, assess current data quality levels, and identify existing gaps or issues.
Conducting a data audit and creating an inventory are foundational steps in establishing both predictive data quality and data observability. This process involves identifying all data sources, cataloging them, and assessing their current quality and reliability.
It provides a comprehensive view of the existing data landscape, setting the stage for targeted improvements.
2. Establish metrics and benchmarks #
- Objective: Set measurable goals for both data quality and observability.
- Actions: Determine the key metrics for data quality (e.g., accuracy, completeness) and observability (e.g., latency, throughput), and establish benchmarks against which to measure performance.
After understanding your data landscape, the next step is to establish metrics and benchmarks. Metrics help you quantify data quality and observability, providing a standard for performance evaluation.
Benchmarks serve as target performance levels, guiding the improvement process. Without clear metrics and benchmarks, it becomes challenging to measure success and identify areas for improvement.
3. Tool selection and implementation #
- Objective: Choose the right technologies to address the unique needs of your organization.
- Actions: Evaluate and select tools for data quality management and observability platforms. Implement these tools, integrating them into your existing data infrastructure.
Choosing the right tools is critical for the effective management of data quality and observability. The selected tools should offer scalability, ease of integration with existing systems, and capabilities tailored to the specific needs of your organization.
Implementation must be carefully planned and executed to ensure compatibility and to minimize disruption to ongoing operations.
4. Predictive modeling and analytics #
- Objective: Build predictive models to anticipate data quality issues and monitor system behavior.
- Actions: Train models on historical data, focusing on forecasting data quality issues. Use analytics to understand system behavior, setting up alerts for abnormal patterns.
Predictive modelling leverages historical data to anticipate future data quality issues and system behavior. This proactive approach enables organizations to identify potential problems before they occur, thus reducing the impact of data quality issues on decision-making and operations.
5. Continuous monitoring and feedback loop #
- Objective: Ensure ongoing effectiveness and adapt to new challenges.
- Actions: Continuously monitor data quality and system performance using the established metrics and benchmarks. Create a feedback loop to iteratively improve the predictive models and system configurations.
Continuous monitoring and establishing a feedback loop are the concluding steps but are no less important. Monitoring ensures that the system performs according to the established metrics and benchmarks. If deviations are detected, the feedback loop helps in readjusting the predictive models or system configurations.
This 5-step process offers a structured approach to implementing predictive data quality and observability. It’s designed to be iterative, allowing for adjustments and improvements over time. Following this process can significantly enhance both the quality and manageability of an organization’s data assets.
7 Best practices for predictive data quality and observability #
Adhering to best practices can significantly improve the effectiveness of your predictive data quality and observability initiatives. Below are some key guidelines to follow.
- Prioritize critical data elements
- Invest in quality training data
- Choose scalable tools
- Implement real-time alerts
- Foster cross-functional collaboration
- Regularly update predictive models
- Document everything
Let us understand them in detail:
1. Prioritize critical data elements #
- Why: Not all data is equally important. Focusing on critical data can yield a higher return on investment.
- How: Identify which data elements are most crucial for business operations and decision-making, and focus your quality and observability efforts on them.
2. Invest in quality training data #
- Why: Good predictive models depend on high-quality training data.
- How: Collect, clean, and validate your data thoroughly before using it to train predictive models for data quality.
3. Choose scalable tools #
- Why: As your organization grows, your tools should be able to accommodate an increasing volume and variety of data.
- How: Opt for tools that offer scalability, both in terms of data handling and feature sets.
4. Implement real-time alerts #
- Why: Immediate notification can help you address issues before they become crises.
- How: Set up real-time alerts based on the predictive analytics, and tie these to actionable steps to be taken when a threshold is crossed.
5. Foster cross-functional collaboration #
- Why: Data quality and observability are cross-departmental concerns.
- How: Establish communication channels between departments like it, business analytics, and operations. Share insights and collaborate on improving data processes.
6. Regularly update predictive models #
- Why: Data patterns change, and models can become outdated.
- How: Periodically retrain your predictive models with new data and adjust the parameters to ensure they are still accurate and relevant.
7. Document everything #
- Why: Proper documentation can save time and avoid confusion.
- How: Document your methods, tools, models, and most importantly, the findings from your predictive data quality and observability initiatives.
By implementing these best practices, organizations can optimize their predictive data quality and observability initiatives for more impactful outcomes. Both the quality and transparency of data are essential for making informed decisions, and following these guidelines can significantly aid in achieving these objectives.
Summing up #
Predictive data quality and observability provide the critical capabilities needed to get ahead of data issues, monitor systems proactively, and optimize data usability.
By implementing predictive analytics, setting clearly defined data quality metrics, choosing adaptable tools, companies can reap immense benefits. However, to realize the full advantages, companies must make these capabilities central elements of their data strategy.
The power of high-quality, observable data is real and substantial. Organizations that lean into predictive data quality and observability place themselves in the best position to leverage data for competitive advantage, risk mitigation, and long-term success.
Predictive data quality and observability: Related reads #
- Data Observability: Definition, Key Elements, & Benefits
- Automation for Data Engineering Teams: What, Why & How?
- Data Quality Explained: Causes, Detection, and Fixes
- 12 Popular Data Observability Tools in 2023: An Overview
- Observability vs. Monitoring: How Are They Different?
- Data Quality Measures: Best Practices to Implement
- How To Improve Data Quality In 12 Actionable Steps?
- 9 Steps to Improve Data Quality in Healthcare in 2023
- Data Quality in Data Governance: The Crucial Link That Ensures Data Accuracy and Integrity
- 6 Popular Open Source Data Quality Tools To Know in 2023: Overview, Features & Resources
Share this article