Data Profiling vs Data Quality: 6 Differences to Know!
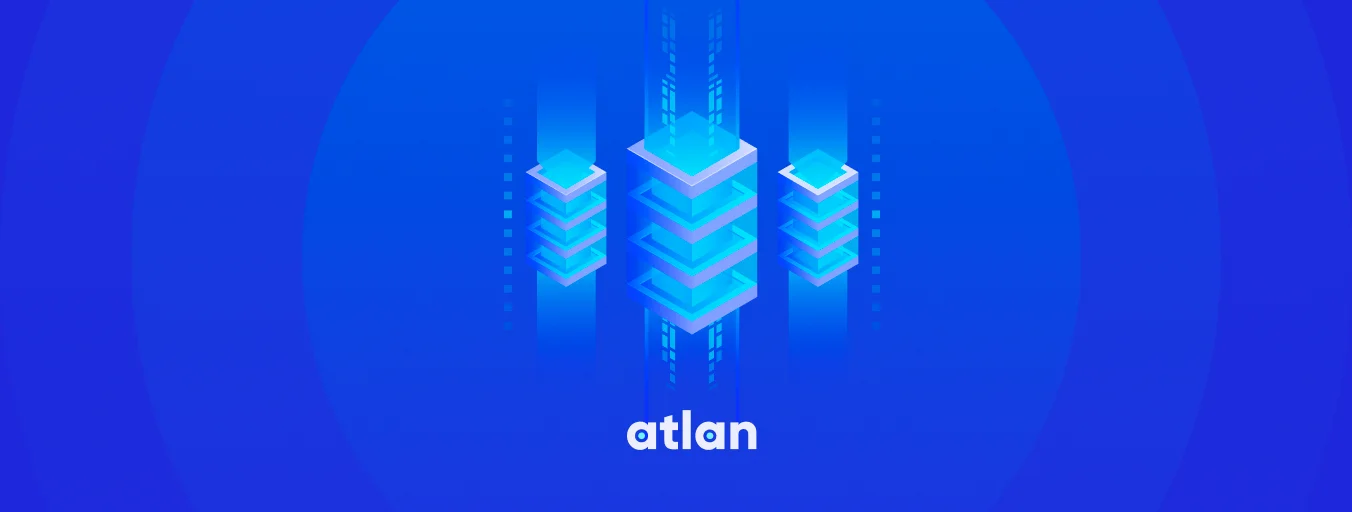
Share this article
In the age of big data, understanding the nuances of data management is crucial. Among the key concepts in this field are data profiling and data quality – two terms often used interchangeably, yet they hold distinct meanings and applications.
This article aims to demystify these terms, shedding light on their unique roles and how they intersect to enhance data integrity and usefulness.
Modern data problems require modern solutions - Try Atlan, the data catalog of choice for forward-looking data teams! 👉 Book your demo today
In this article, we will explore:
- The distinct roles and intersections of data profiling and data quality.
- Practical applications and benefits
- Challenges and best practices for data profiling and data quality
Ready? Let’s dive in!
Table of contents #
- Data profiling vs data quality: 6 Key differences
- Practical applications and benefits
- Data profiling vs data quality: Challenges and best practices
- Conclusion
- Data profiling vs data quality: Related reads
Data profiling vs data quality: 6 Key differences #
Data profiling and data quality are two pillars of modern data management, each playing a critical role in the utilization and interpretation of data. However, their functions and impacts vary, making it essential to understand their distinct purposes and how they complement each other.
Data profiling is the process of examining data available in an existing database and collecting statistics and information about that data. This process is akin to taking a ‘data census.’ It involves understanding the structure, content, relationships, and derivation rules of data.
Data profiling helps identify inconsistencies, anomalies, and deviations, which could indicate deeper issues in data quality or integrity. It is a diagnostic tool used to understand data’s potential for analysis and processing.
On the other hand, data quality refers to the condition or health of data with respect to its suitability for a particular purpose. It encompasses aspects such as accuracy, completeness, reliability, and relevance.
Ensuring high data quality means the data is fit for its intended uses in operations, decision making, and planning. Data quality management involves the processes, policies, and technologies needed to maintain and improve the quality of data throughout its lifecycle.
The intersection of these two concepts is crucial. Data profiling acts as a preliminary step in the broader scope of data quality management. By profiling data, organizations can identify areas where the quality of data may not meet the required standards.
It is through profiling that the dimensions of data quality – accuracy, completeness, consistency, and more – are evaluated.
For example, in a customer database, data profiling might reveal that certain records are incomplete or inconsistent. This discovery directly informs the data quality initiative, which would then aim to correct these issues, ensuring the database’s reliability for marketing or customer service purposes.
Aspect | Data profiling | Data quality |
---|---|---|
Definition | The process of analyzing the existing data to understand its structure, content, and relationships. | The measure of data's condition, focusing on its suitability for specific uses. |
Focus | Data characteristics, such as patterns, formats, and anomalies. | Data accuracy, completeness, reliability, and relevance. |
Purpose | To diagnose and understand data, identifying potential issues. | To ensure that data is appropriate and reliable for its intended use. |
Process | Analyzing data to gather statistics and insights. | Implementing processes and policies to maintain and improve data quality. |
Outcome | Understanding of data's structure and identification of inconsistencies. | High-quality data that is fit for decision making and operational use. |
Role in data management | Preliminary step in data quality assessment. | Ongoing process for maintaining data integrity and usability. |
In summary, while data profiling is an analytical process that helps understand the characteristics of data, data quality is an evaluative and corrective process ensuring that data is suitable for use. Both are interdependent and essential for any data-driven organization aiming to make accurate and effective decisions.
Practical applications and benefits of data profiling and data quality #
Understanding the distinctions between data profiling and data quality is not just an academic exercise; it has practical implications and benefits for organizations. By effectively utilizing these processes, companies can enhance their decision-making, improve operational efficiency, and gain a competitive edge.
Here are the benefits and applications:
- Enhanced data understanding through data profiling
- Achieving high-quality data for decision-making
- Synergy of data profiling and data quality in operations
- Case studies and real-world examples
Let’s look at them in detail.
1. Enhanced data understanding through data profiling #
Data profiling enables businesses to gain in-depth insights into customer data, revealing patterns and trends that can inform marketing strategies and customer service improvements.
When merging data from different sources, data profiling helps ensure compatibility and consistency, crucial for accurate analytics and reporting.
2. Achieving high-quality data for decision-making #
With high-quality data, businesses can make more informed and reliable decisions. Data quality ensures that the information used in decision-making processes is accurate, complete, and timely.
Many industries have strict data quality requirements. Maintaining high data quality ensures compliance with these regulations, reducing the risk of legal issues and fines.
3. Synergy of data profiling and data quality in operations #
Combining data profiling and data quality initiatives leads to more efficient data management, as profiling identifies issues that data quality measures can then address.
By identifying and rectifying data issues early, organizations can avoid the high costs associated with bad data, such as errors in customer outreach or flawed strategic decisions.
4. Case studies and real-world examples #
A retail company used data profiling to identify discrepancies in customer data across different channels. Addressing these with data quality measures led to a unified view of the customer, enhancing personalized marketing efforts.
In essence, the practical application of data profiling and data quality not only optimizes data but also translates into tangible benefits for businesses. Whether it’s through more accurate decision-making, regulatory compliance, or operational efficiencies, the synergy of these processes is a cornerstone of effective data management.
Data profiling vs data quality: Challenges and best practices #
Navigating the complexities of data profiling and data quality presents several challenges.
- Volume and variety of data
- Maintaining data quality
- Resource constraints
Let’s look at them in detail:
1. Volume and variety of data #
One of the foremost challenges is the volume and variety of data that organizations handle today. The exponential increase in data sources and the vast amount of data generated make profiling and maintaining quality a daunting task.
This complexity is compounded by the need to integrate diverse data systems, where data from varied sources and formats must be harmonized, adding layers of complexity to both data profiling and quality management.
2. Maintaining data quality #
Another significant challenge lies in maintaining data quality over time. As business environments and data itself are ever-evolving, ensuring the ongoing relevance and accuracy of data is a continuous task that requires vigilance and adaptability.
This is particularly challenging as data can deteriorate or become outdated, requiring ongoing efforts to validate and update it to maintain its integrity.
3. Resource constraints #
Furthermore, organizations often grapple with resource constraints when it comes to data management. Implementing robust data profiling and quality management processes demands not only advanced tools but also skilled personnel trained in these specific areas.
The investment in both technology and training can be substantial, yet it is essential for effective data management.
These challenges, while significant, are not insurmountable. With a strategic approach to data management, organizations can overcome these hurdles and leverage their data effectively for better decision-making and operational efficiency.
Conclusion #
Data profiling, as we’ve seen, is a critical diagnostic tool that helps organizations understand the intricacies of their data, revealing patterns and inconsistencies.
Meanwhile, data quality focuses on ensuring that the data is fit for its intended purpose, underpinning reliable decision-making and operational processes.
However, the journey is not without challenges. The vast volume and variety of data, the need for continuous data quality maintenance, and the complexities of integrating diverse data systems present significant hurdles.
Overcoming these challenges requires a combination of regular data audits, robust data management tools, and a commitment to ongoing training and development.
In essence, the interplay between data profiling and data quality is a cornerstone of modern data management, vital for any organization looking to leverage its data as a strategic asset.
As we conclude, it’s clear that the journey to mastering these practices is ongoing, evolving alongside the ever-changing landscape of data and technology.
Data profiling vs data quality: Related reads #
- 11 Essential Benefits of Data Profiling You Can’t Overlook in 2023!
- Data Profiling: Definition, Techniques, Process, and Examples
- Data Quality is Everyone’s Problem, but Who is Responsible?
- Data Quality and Observability: Key Differences & Relationships!
- Data Quality Measures: Best Practices to Implement
- Data Quality Explained: Causes, Detection, and Fixes
- Data Integrity vs Data Quality: Nah, They Aren’t Same!
- Data Profiling Example: 10 Real World Examples
Share this article