Is Data Quality the Secret Sauce to Skyrocketing ROI?
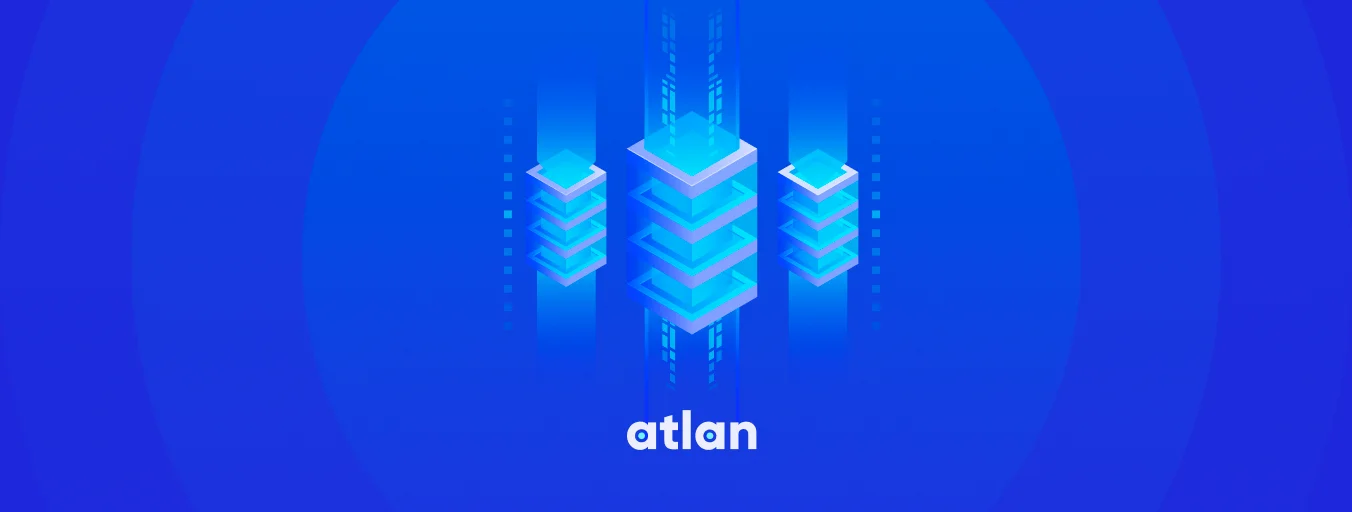
Share this article
In today’s data-driven world, the quality of information can make or break an organization. Accurate, reliable, and timely data is crucial for strategic decision-making, customer engagement, and operational efficiency. However, ensuring high data quality isn’t free; it requires an investment in both resources and technology.
This raises an essential question: What is the Return on Investment (ROI) for data quality initiatives? Understanding ROI on data quality provides organizations with a quantitative measure of the financial gains relative to the costs incurred in improving data quality.
Modern data problems require modern solutions - Try Atlan, the data catalog of choice for forward-looking data teams! 👉 Book your demo today
This crucial metric can offer insights into the value generated by cleaner, more accurate, and more reliable data.
This guide will dive deep into ROI on data quality, exploring its importance, how it can be measured, and strategies for maximizing it.
Let us dive in!
Table of contents #
- What is ROI on data quality?
- 7 Steps to measure ROI on data quality
- Financial impacts of poor data quality for organizations
- How to improve data quality to maximize the ROI of data quality?
- Value associated with improved data quality
- Summary
- ROI on data quality: Related reads
What is ROI on data quality? #
ROI, or Return on Investment, on data quality refers to the measurable financial benefits that an organization gains as a result of investing in improving the quality of its data. The concept operates on the premise that better quality data can lead to more accurate analyses, smarter decision-making, improved operational efficiencies, and ultimately, a stronger competitive advantage.
In simple terms, it’s the value gained versus the money and resources spent on initiatives to improve data quality. Investing in data quality can include a variety of actions such as cleaning existing databases, implementing data governance frameworks, standardizing data collection procedures, and adopting new data management technologies.
While these initiatives often require a substantial investment of both time and financial resources, the end goal is to achieve a higher ROI by enabling the organization to operate more efficiently and make more informed decisions.
The ROI on data quality is usually calculated by measuring the benefits—like increased revenue, cost savings, or improved customer satisfaction—against the investment made to achieve those gains.
The aim is to demonstrate that the benefits substantially outweigh the costs, thereby justifying the investment in data quality improvements.
7 Steps to measure ROI on data quality #
Measuring the Return on Investment (ROI) on data quality can be a complex task, as it involves quantifying both the tangible and intangible benefits that derive from improving data quality.
However, by focusing on a set of key performance indicators (KPIs) and metrics, organizations can develop a clearer picture of the financial returns generated from their investment in data quality.
Below are some of the steps and metrics involved in measuring ROI on data quality.
- Identify costs of poor data quality
- Calculate the investment
- Define KPIs and metrics
- Calculate ROI
- Monitor and refine
- Present the findings
- Secure buy-in for future initiatives
Let’s delve into a more in-depth analysis of the metrics used for assessing the ROI 0n data quality.
1. Identify costs of poor data quality #
Before you can measure the return, you need to understand the cost. Quantify how much poor data quality has been costing your organization in terms of operational inefficiencies, lost revenue opportunities, and any penalties from non-compliance with regulations.
2. Calculate the investment #
Add up all the costs associated with improving data quality. This can include software and hardware costs, consulting fees, internal man-hours spent on data cleanup and transformation, and ongoing maintenance.
3. Define KPIs and metrics #
Determine the key performance indicators that are most relevant to your organization’s goals. This could include customer retention rates, operational efficiency metrics, or revenue growth. Lets see some examples below.
- Cost savings
Calculate the amount saved due to a reduction in errors, decreased time spent on data-related tasks, and other operational efficiencies gained. Translate these into dollar figures wherever possible.
- Revenue generation
Measure the increase in revenue that can be directly attributed to improved data quality. For instance, if cleaner, more accurate data led to a more successful marketing campaign, quantify the additional revenue generated.
- Time-to-value
Calculate how quickly the organization realized benefits from the data quality initiative compared to the time it took to make the initial investment.
- Customer satisfaction
While more difficult to quantify, improvements in customer satisfaction or Net Promoter Scores (NPS) due to better data can also be considered a return on investment.
4. Calculate ROI #
Use the following formula to calculate ROI:
ROI=(Gain from Investment−Cost of Investment)/Cost of Investment×100
5. Monitor and refine #
ROI on data quality is not a one-time calculation. Continuously monitor the KPIs and metrics, and adjust your data quality initiatives as needed to maximize ROI.
6. Present the findings #
Compile all the information into a comprehensive report detailing how the ROI was calculated, what the findings were, and what steps are recommended for future data quality initiatives.
7. Secure buy-in for future initiatives #
Use the ROI calculations and findings to secure organizational buy-in for ongoing and future data quality projects. High ROI is compelling evidence of the value generated by investing in data quality.
By rigorously measuring ROI on data quality, organizations can not only justify the resources allocated to data quality initiatives but also optimize these initiatives for better financial outcomes.
It’s essential to approach this as an ongoing process that requires regular monitoring and adjustment to align with the organization’s evolving objectives and market conditions.
Financial impacts of poor data quality for organizations #
Poor data quality can have a significant and often underestimated financial impact on organizations. The consequences are not just limited to incorrect reporting; they can permeate various aspects of business operations and decision-making, ultimately affecting the bottom line.
Here are some of the key financial impacts.
- Lost revenue
- Increased operational costs
- Fines and regulatory penalties
- Poor decision-making
- Customer churn
- Reputational damage
- Inventory management issues
- Ineffective marketing
Lets explore each impacts in details.
1. Lost revenue #
One of the most direct impacts of poor data quality is lost revenue. Whether it’s through faulty pricing strategies, incorrect invoicing, or ineffective marketing campaigns, bad data can result in missed opportunities for income generation.
For example, if a retailer’s database contains incorrect information about product availability, it could lead to lost sales both online and in-store.
2. Increased operational costs #
When data is inaccurate or incomplete, additional resources are required to correct errors and fill gaps. This manifests as higher operational costs related to data cleansing, manual verification, and reconciliation efforts.
Employees waste valuable time sifting through poor-quality data, time that could otherwise be used for tasks that add value to the organization.
3. Fines and regulatory penalties #
Many industries are subject to stringent data governance and compliance requirements. Poor data quality can lead to reporting errors and non-compliance with industry regulations, such as GDPR in Europe or HIPAA in the United States.
Non-compliance can result in hefty fines and legal penalties, adding a financial burden to the organization.
4. Poor decision-making #
Decisions based on inaccurate data are likely to be flawed, potentially leading to financial losses. For instance, if an investment decision is made based on incorrect market research data, the company might face significant financial setbacks.
Similarly, poor data quality can result in suboptimal resource allocation, reducing the organization’s efficiency and profitability.
5. Customer churn #
Inaccurate customer data can severely impact the quality of customer service, resulting in decreased customer satisfaction and potentially leading to customer churn.
Acquiring new customers is often more expensive than retaining existing ones, so customer loss due to poor data quality can result in increased marketing and acquisition costs.
6. Reputational damage #
While the financial impact of reputational damage may be hard to quantify, it can have long-term implications for revenue and market share.
Poor customer experiences stemming from bad data can lead to negative reviews and bad press, making it challenging to attract new customers or partners, thereby affecting future income.
7. Inventory management issues #
For retail and manufacturing businesses, poor data quality can result in inefficient inventory management. This could mean overstocking or understocking products, both of which have financial repercussions.
Overstocking leads to increased holding costs, while understocking results in lost sales opportunities.
8. Ineffective marketing #
Inaccurate customer profiles and contact lists can render marketing campaigns ineffective. Money spent on targeting the wrong audience is essentially wasted, reducing the ROI of marketing efforts. Poor data can also lead to missed opportunities for upselling or cross-selling.
In summary, poor data quality can have a broad and substantial financial impact on an organization. From increased operational costs to lost revenue and legal penalties, the implications are far-reaching.
Organizations that underestimate the importance of data quality do so at their own peril, often facing reduced profitability and long-term financial instability.
How to improve data quality to maximize the ROI of data quality? #
Maximizing the Return on Investment (ROI) on data quality initiatives requires a comprehensive strategy that addresses both the technical and organizational aspects of data management. By focusing on key areas that are prone to data quality issues and aligning them with business objectives, organizations can significantly improve their data quality.
Here are some steps to take.
- Establish a data governance framework
- Perform data audits
- Implement data quality tools
- Standardize data entry procedures
- Master data management
- Validate data in real-time
- Monitor data quality metrics
- Ensure compliance and security
- Involve business stakeholders
- Periodic review and adaptation
Lets discuss each step in detail.
1. Establish a data governance framework #
Create a formal data governance framework that outlines the roles, responsibilities, and procedures for data management within the organization. This helps ensure that everyone is aligned and accountable for maintaining data quality.
2. Perform data audits #
Conduct regular audits to assess the current state of data quality. This includes identifying inaccuracies, inconsistencies, and redundancies. Use these insights to pinpoint areas that need immediate attention.
3. Implement data quality tools #
Use advanced data quality tools that offer features like data cleansing, validation, and profiling. These tools can automatically correct inaccuracies, verify data against predefined standards, and identify anomalies, thus improving the quality of data.
4. Standardize data entry procedures #
Implement standardized data entry procedures across all organizational departments and channels. Training staff on the importance of data accuracy and how to input data consistently can significantly reduce errors.
5. Master data management #
Adopt a Master data management system to create a single, authoritative view of your data. MDM involves consolidating data from various sources to provide a unified, accurate version of key data entities, while improving data quality and business process management efforts.
6. Validate data in real-time #
Incorporate real-time validation mechanisms to catch errors as they occur. This is especially critical for data that directly influences business decisions and customer interactions.
7. Monitor data quality metrics #
Use Key Performance Indicators (KPIs) to continuously monitor data quality. This will not only indicate the effectiveness of your data quality initiatives but will also provide actionable insights for improvement.
8. Ensure compliance and security #
Make sure that all data quality initiatives comply with relevant regulations like GDPR or HIPAA. This not only protects the organization against legal repercussions but also adds an additional layer of data integrity.
9. Involve business stakeholders #
Involve business users in the process of defining data quality requirements, as they are often the most familiar with how data is used and what quality means in the context of their specific roles.
10. Periodic review and adaptation #
Data quality is not a one-time project but an ongoing process. Regularly review the ROI of your data quality initiatives and adapt your strategies as needed to ensure continuous improvement.
By focusing on these areas, organizations can significantly improve the quality of their data, leading to better decision-making, increased operational efficiency, and a higher ROI on data quality initiatives.
It’s a continuous cycle: improved data quality boosts ROI, which in turn justifies further investment in data quality initiatives, thereby creating a virtuous circle of improvement and value generation.
Value associated with improved data quality #
Data quality is increasingly recognized as a critical factor in the success of any organization.
High-quality data serves as the foundation for various business activities, from operational processes to strategic decision-making.
Here are some values associated with improved data quality for organizations.
- Improved decision-making
- Increased operational efficiency
- Enhanced customer experience
- Competitive advantage
- Compliance and risk management
- Better financial management
- Increased revenue and profitability
- Innovation and adaptability
Let us discuss the values in detail:
1. Improved decision-making #
Accurate, reliable, and timely data is crucial for making informed decisions. High-quality data provides the insights that executives, managers, and even front-line employees need to make choices that are in the best interest of the organization.
Whether it’s deciding on a new market to enter or identifying efficiency improvements, quality data is indispensable.
2. Increased operational efficiency #
Poor data quality can be a significant drain on resources. Errors need to be corrected, discrepancies must be reconciled, and bad data generally leads to inefficient processes.
On the other hand, high-quality data streamlines operational workflows, reducing the time and effort needed to complete tasks. This operational efficiency often translates into cost savings and better allocation of resources.
3. Enhanced customer experience #
Understanding customer behaviour, needs, and preferences is easier when you have quality data. Organizations can offer more personalized and effective services or products, thereby enhancing customer satisfaction and loyalty.
This not only improves the customer experience but also boosts the organization’s reputation and profitability.
4. Competitive advantage #
Quality data can be a significant differentiator in today’s competitive marketplace. Organizations with better data can gain insights more quickly, react to market changes more effectively, and generally outperform their competitors who make decisions based on poor-quality data.
5. Compliance and risk management #
High-quality data is essential for fulfilling various regulatory requirements. Data inaccuracies can lead to non-compliance, resulting in fines and penalties.
Additionally, quality data is crucial for accurate risk assessment, helping organizations to better prepare for potential market or operational challenges.
6. Better financial management #
Accurate data allows for precise budgeting, forecasting, and financial reporting. This results in better capital allocation and enhances the organization’s ability to attract investment.
7. Increased revenue and profitability #
High-quality data enables more effective marketing campaigns, better customer segmentation, and more strategic decision-making. These factors often lead to increased sales, higher customer retention rates, and ultimately, greater profitability.
8. Innovation and adaptability #
With quality data, organizations can more readily identify trends and market gaps, providing the insights necessary for innovation. This helps them adapt to changing market conditions and consumer behaviors, ensuring long-term success.
In summary, high-quality data touches almost every aspect of business operations. Its benefits are wide-ranging, from strategic advantages like better decision-making and increased competitiveness to operational benefits such as efficiency and cost savings.
Investing in data quality can yield substantial returns, making it a critical component of modern business strategy.
Summary #
The importance of data quality in today’s data-driven business environment cannot be overstated. Poor [data quality] not only undermines business intelligence but also has a measurable financial impact.
Consequently, organizations that invest in improving data quality can expect a favorable Return on Investment (ROI).
Tools like data audits, data governance frameworks, Master Data Management systems, and real-time validation can substantially improve data quality. Employee training and cross-departmental collaboration further ensure that the entire organization is geared towards maintaining high data quality standards.
Therefore, given its crucial role in organizational success, an investment in data quality should not be viewed as an expense but rather as a strategic initiative that has the potential to deliver significant returns.
By making data quality a priority, organizations can unlock their data’s true potential, driving not just short-term gains but also long-term sustainable growth.
ROI on data quality: Related reads #
- Data Quality Measures: Best Practices to Implement
- How To Improve Data Quality In 12 Actionable Steps?
- Data Quality Fundamentals: Why It Matters in 2023!
- Data Quality Dimensions: A Primer on Data Quality Measurement
- Data Quality and Observability - Why Are They Important?
- 6 Reasons Why Data Quality Needs a Data Catalog
- A Blueprint for Bulletproof Data Quality Management
- Data Quality Issues? Here Are 6 Ways to Fix Them!
- How to Ensure Data Quality in Healthcare Data: Best Practices and Key Considerations
- Data Quality in Data Governance
- 6 Popular Open Source Data Quality Tools in 2023: Overview, Features & Resources
- Automated quality control of data pipelines
- Resolving Data Quality Issues in the Biggest Markets
- Bad Data: How It Sneaks in & How to Manage It in 2023
Share this article