What is in a Data Catalog? 6 Things You Should Know in 2024
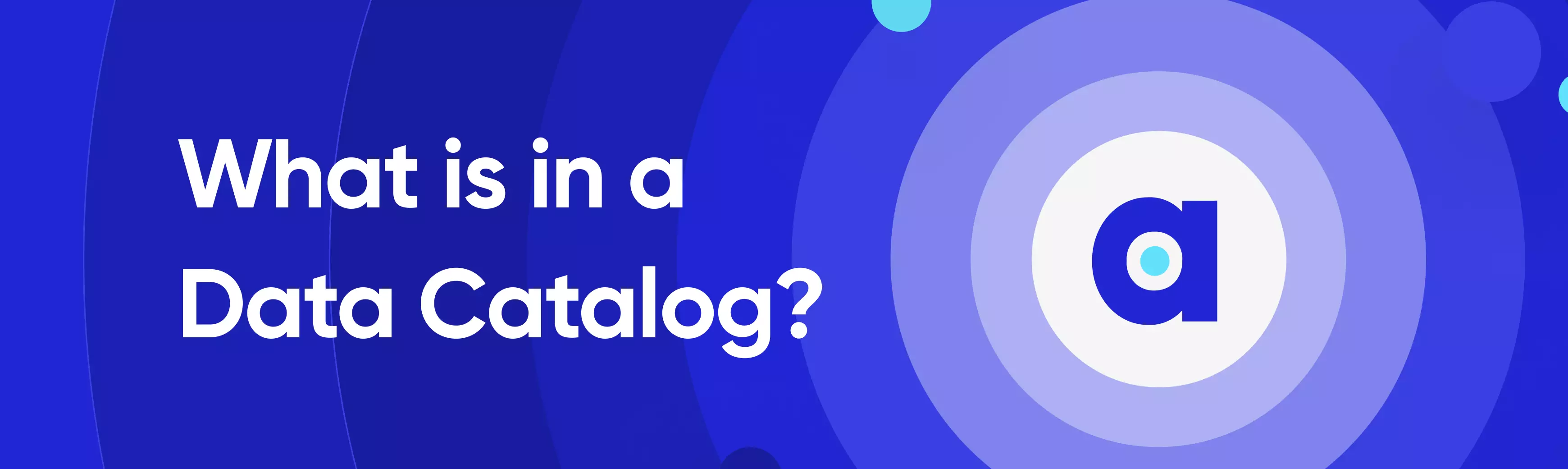
Share this article
A data catalog consists metadata, data profiling, data lineage and relationships, search & discovery, data access & security, and collaboration & social features. It is a centralized and organized repository that serves as a single source of truth for your organization’s data. It enables users to easily discover, understand, and manage data from various sources, including databases, data warehouses, and data lakes.
A data catalog is especially useful when dealing with large-scale and complex data environments, and when aiming to make it accessible to both technical and non-technical users.
Modern data problems require modern solutions - Try Atlan, the data catalog of choice for forward-looking data teams! 👉 Book your demo today
In this article, we will explore:
- What is in a data catalog?
- Integration capabilities of a data catalog
- Ideal features of a data catalog
Ready? Let’s dive in!
Table of contents #
- What is in a data catalog?
- What is a data catalog tool?
- Understanding a data catalog’s integration capabilities and the impact on its features
- What should a data catalog offer: Must-have and good-to-have features to get the most out of it
- Why Atlan with active metadata and AI is a strong data catalog option
- Bringing it all together
- What is in a data catalog? Related reads
What is in a data catalog? #
A data catalog is a critical component of modern data management and analytics. It serves as a centralized repository where an organization’s metadata is stored, making it easier for data professionals and business users to find, understand, and trust the right data for their needs. Here are the detailed components of a data catalog:
A data catalog typically contains the following components:
- Metadata repository
- Data discovery and search
- Data governance and compliance
- Data quality indicators
- Collaboration and social features
- Data lineage and provenance
- Integration with data sources and tools
- User-friendly interface
- Security and privacy controls
- Automated metadata management
- Reporting and analytics
- Customization and extensibility
Let us look into each of the above components in brief:
1. Metadata repository #
The core of a data catalog is its metadata repository. Metadata is essentially data about data. This includes structural metadata (like table names, column names, and data types), descriptive metadata (like data lineage, annotations, or descriptions), and administrative metadata (like who created the data and when).
2. Data discovery and search #
A data catalog provides robust search functionality to enable users to easily find the data they need. This often includes keyword search, filtering capabilities, and categorization of data assets.
Advanced catalogs may also offer semantic search, which understands the context of search queries, making it easier to find relevant results.
3. Data governance and compliance #
Effective data governance ensures that data is managed and used appropriately, in compliance with internal policies and external regulations.
This includes managing data access controls, setting up data privacy measures, and ensuring data is used ethically.
4. Data quality indicators #
A data catalog often provides insights into the quality of data, such as its accuracy, completeness, consistency, and reliability.
This helps users assess the trustworthiness and suitability of a dataset for their specific use cases.
5. Collaboration and social features #
To foster a collaborative environment, data catalogs often include features such as user ratings, reviews, and comments on datasets.
This social aspect helps users share knowledge and insights about data, and make informed decisions based on collective expertise.
6. Data lineage and provenance #
Understanding where data comes from, how it’s processed, and how it moves through various systems (data lineage) is crucial for assessing its quality and reliability.
A data catalog tracks this lineage and provides a visual representation of the data lifecycle.
7. Integration with data sources and tools #
Data catalogs need to integrate with various data sources (like databases, data lakes, and cloud storage) and tools (like BI platforms, ETL tools, and analytics software) to extract metadata and provide a unified view of an organization’s data landscape.
8. User-friendly interface #
A well-designed user interface is essential for a data catalog. It should be intuitive and easy to navigate, allowing users to find, understand, and interact with data assets efficiently.
9. Security and privacy controls #
Given the sensitive nature of data, data catalogs incorporate robust security measures, including access controls, encryption, and audit trails, to protect data integrity and comply with privacy regulations.
10. Automated metadata management #
Advanced data catalogs use machine learning and AI to automate the process of metadata collection, classification, and curation, which enhances efficiency and accuracy.
11. Reporting and analytics #
Some data catalogs offer reporting and analytics features to track the usage, performance, and impact of data assets within the organization.
12. Customization and extensibility #
Flexibility to customize the data catalog according to specific organizational needs and the ability to extend its capabilities through APIs and plugins can be an important feature.
By bringing together these elements, a data catalog becomes a vital tool for data-driven organizations, enabling better data discovery, quality, governance, and collaboration.
What is a data catalog tool? #
A data catalog tool is a comprehensive solution designed to manage an organization’s data assets, facilitating easier access, understanding, and governance of data. Here’s a detailed explanation of what a data catalog tool encompasses:
Purpose and functionality #
- Centralized data repository: It acts as a centralized repository for metadata (data about data), allowing users to find and manage data across various sources like databases, data lakes, and cloud storage systems.
- Data discovery and search: The tool enables users to easily search for and discover data assets within the organization. Advanced search features include keyword searches, filtering by various attributes, and semantic search capabilities that understand the context of queries.
- Metadata management: It involves the collection, storage, and organization of metadata. This includes structural metadata (like data types and relationships), descriptive metadata (such as annotations or user comments), and administrative metadata (e.g., ownership and usage policies).
Key features #
- Data governance and compliance: The tool supports data governance by managing data access, ensuring data quality, and enforcing policies for data security and compliance with regulations like GDPR or HIPAA.
- Data lineage and provenance: It tracks the lineage of data - where it originates, how it moves, and transforms across systems. This is crucial for understanding data context, quality, and for audit purposes.
- Collaboration features: Many data catalog tools offer social features like user reviews, ratings, and the ability to comment on datasets. This fosters a collaborative environment where users can share insights and best practices.
- Integration capabilities: A robust data catalog tool can integrate with a variety of data sources and tools (like BI platforms, ETL tools, etc.), enabling seamless metadata extraction and management.
- Quality indicators: It provides indicators of data quality, helping users to assess the reliability and suitability of a dataset for their specific needs.
- User interface and experience: They are designed with user-friendly interfaces, making it easy for users to navigate and interact with the catalog and find the necessary data efficiently.
Advanced features #
- AI and machine learning: Advanced tools may use AI and machine learning algorithms to automate metadata collection, categorization, and even suggest relevant datasets based on user behavior or needs.
- Security and privacy controls: Ensuring data security and privacy is a key aspect, with features like access controls, encryption, and audit trails to protect data and comply with regulations.
- Customization and extensibility: The ability to customize the catalog and extend its capabilities through APIs or plugins can be crucial for organizations with specific needs.
Benefits #
- Improved data accessibility: Makes it easier for users to find and access the right data when they need it.
- Enhanced data literacy: Helps in understanding data, its context, and its use within the organization.
- Efficient data management: Streamlines the process of managing, categorizing, and maintaining data.
- Better compliance and governance: Supports compliance with regulations and internal data policies.
- Informed decision making: By providing quality, lineage, and context, it aids in making data-driven decisions.
Use cases #
- In business intelligence, to find and use relevant datasets for analysis.
- In data governance, to enforce policies and track compliance.
- In IT, for managing and cataloging data across disparate systems.
A data catalog tool is essentially a crucial component for organizations aiming to harness the full potential of their data assets in a governed, efficient, and user-friendly manner.
Understanding a data catalog’s integration capabilities and the impact on its features #
A data catalog can integrate with various components of an organization’s data infrastructure, including databases, data warehouses, data lakes, ETL tools, and business intelligence (BI) tools, among others. The integrations impact the data catalog in several ways:
- Data sources
- ETL and data integration tools
- Data quality and governance tools
- Business intelligence (BI) and analytics tools
- Data science platforms and machine learning frameworks
- Data security and access control systems
Let us look into each of these integrations in brief:
1. Data sources #
A data catalog integrates with databases, data warehouses, and data lakes, which serve as primary data storage systems. This allows the catalog to gather metadata and profile data from various sources and provide a comprehensive view of the data landscape.
2. ETL and data integration tools #
ETL (Extract, Transform, Load) tools and other data integration solutions are used to move and transform data between systems. Integration with these tools enables the data catalog to track data lineage, understand data transformations, and maintain up-to-date metadata.
3. Data quality and governance tools #
Integrating with data quality and governance tools allows the data catalog to enforce data quality rules, apply data governance policies, and track data quality metrics. This helps ensure that the catalog contains accurate, reliable, and compliant information.
4. Business intelligence (BI) and analytics tools #
By integrating with BI and analytics tools, the data catalog can provide context for reports, dashboards, and analyses, making it easier for users to understand and trust the data they’re working with. Integration also allows users to access data catalog features (e.g., search, metadata) directly within their analytics tools, streamlining the data discovery and analysis process.
5. Data science platforms and machine learning frameworks #
Integrating with data science platforms and machine learning frameworks allows data scientists to leverage the data catalog’s metadata, profiling, and lineage information in their workflows. This can improve the efficiency and accuracy of data preparation, feature engineering, and model evaluation.
6. Data security and access control systems #
By integrating with security and access control systems, data catalogs can enforce data protection policies, manage user permissions, and ensure that sensitive data is only accessible to authorized users.
The integrations between a data catalog and other components of your data ecosystem have a significant impact on the catalog’s content and capabilities.
Ultimately, these integrations enhance the value of the data catalog, making it a more effective tool for empowering users and driving data-driven decision-making.
What should a data catalog offer? Must-have and good-to-have features to get the most out of it #
When evaluating data catalog software, it’s essential to consider both the must-have and good-to-have features. These features will help your organization meet its current needs while also ensuring it is future-ready. Here’s a breakdown of the must-have and good-to-have features:
Data catalog software: Must-have features #
Here are the must-have features in data catalog software:
1. Metadata management #
Robust metadata management is crucial for any data catalog. The software should be able to automatically extract, store, and update metadata from various data sources, including technical, business, and operational metadata.
2. Data profiling and quality #
The data catalog should provide data profiling capabilities to assess data quality, identify data anomalies, and highlight data patterns. This helps users trust the data and make informed decisions.
3. Data Lineage and relationship mapping #
The ability to track data lineage and map relationships between data sets is essential. This feature provides transparency into data’s origin, transformations, and dependencies, ensuring users understand the impact of changes on downstream processes.
4. Search and discovery #
A powerful search and discovery functionality is a must for any data catalog. Users should be able to easily find relevant data sets based on keywords, tags, or other criteria.
5. Data access and security #
The data catalog should integrate with your organization’s existing security infrastructure, enforce access controls, and maintain data compliance. This ensures that sensitive data is only accessible to authorized users.
6. Scalability and performance #
As your organization’s data needs to grow, the data catalog should be able to scale and maintain high performance. Choose a solution that can handle increasing data volumes and sources without compromising performance.
Data catalog software: Good-to-have features #
Here are the good-to-have features of a data catalog software:
1. Collaboration and social features #
While not mandatory, collaboration features like commenting, annotations, and reviews can help foster a data-driven culture within your organization and encourage knowledge sharing among users.
2. Integration with BI, analytics, and data science tools #
Integration with your existing BI, analytics, and data science tools allows users to leverage the data catalog’s features directly within their familiar tools, streamlining their workflows.
3. Machine learning and AI capabilities #
AI and machine learning can enhance data catalog features by automating metadata generation, suggesting relationships, and improving search and discovery through natural language processing (NLP).
4. Customization and extensibility #
A data catalog that offers customization and extensibility options allows your organization to tailor the solution to your specific needs and processes, ensuring a better fit for your data ecosystem.
5. Cloud-native architecture #
A cloud-native data catalog provides flexibility, scalability, and cost-effectiveness. It can also simplify integration with cloud-based data storage solutions like Snowflake.
By prioritizing the must-have features and considering the good-to-have ones, you can choose a data catalog solution that best meets your organization’s current and future needs.
Why Atlan with active metadata and AI is a strong data catalog option #
Atlan, with its active metadata platform and AI capabilities, could be a strong option for your data catalog needs. The introduction of Atlan AI as a co-pilot for data teams brings several advantages that can significantly improve your data management and discovery processes.
Let’s look at some key benefits that Atlan AI brings to your organization:
1. Chat-based data discovery and auto-generating SQL #
Atlan AI simplifies data exploration by allowing users to search for data and get answers to their questions using natural language. This eliminates the need to open a query editor or write SQL, making it accessible for both technical and non-technical users.
2. Automated documentation #
Atlan AI streamlines the documentation process by generating a first draft of descriptions and READMEs for your data assets based on their name, schema, and lineage context. This can save time and effort on manual documentation, especially for organizations with a large backlog of assets.
3. Exploratory capabilities #
Atlan AI suggests questions to ask your data and shows you what questions your team is asking, promoting data exploration and insights generation for all team members, regardless of their technical expertise.
4. Integrations with modern data stack #
Atlan’s platform integrates with popular tools like Slack, Snowflake, dbt Labs, Redshift, Looker, Sisense, and Tableau, ensuring seamless connectivity with your existing data infrastructure.
5. Industry recognition #
Atlan has been recognized by Forrester, Gartner, and G2 as a leader in data catalogs, data governance, machine learning data catalogs, and data quality, indicating a strong and reliable solution for your organization.
Considering these features and benefits, Atlan and its AI-powered capabilities could be a great fit for your organization’s data catalog needs. Its focus on simplifying data discovery and management while promoting collaboration and accessibility for all team members.
Bringing it all together #
Deploying a data catalog starts the seeding process of data democratization and data enablement in your organization. It helps users discover, understand, and trust the data while ensuring compliance and governance.
However, not all data catalogs are created equal. To get the most out of it, you need to know its key components, integration capabilities, and its features that help you meet your organization’s current and future needs.
Are you looking for a data catalog for your organization — you might want to check out Atlan.
What is in a data catalog? Related reads #
- AI Data Catalog: Exploring the Possibilities That Artificial Intelligence Brings to Your Metadata Applications & Data Interactions
- How AI Data Governance Shows Potential To Help You Scale Data Security, Integrity, Privacy, and Compliance
- 8 Ways AI-Powered Data Catalogs Save Time Spent on Documentation, Tagging, Querying & More
- Data Catalog: Does Your Business Really Need One?
- Open Source Data Catalog Software: 5 Popular Tools to Consider in 2024
- Enterprise data catalog: Definition, Importance & benefits
- Google Cloud Data Catalog Guide - Everything You Need to Know
- AWS Glue Data Catalog: Architecture, Components, and Crawlers
- Data Catalog Platform: The Key To Future-Proofing Your Data Stack
Share this article